pdirpath = os.path.join(os.path.split(d)[0])语句什么含义
时间: 2023-11-23 14:53:59 浏览: 172
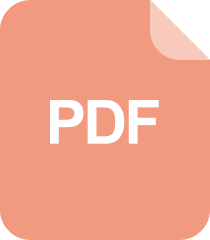
Python对文件和目录进行操作的方法(file对象/os/os.path/shutil 模块)
这条语句的含义是将路径d中的目录部分分割出来,并将其与空字符串连接起来,得到一个新的路径字符串pdirpath。其中,os.path.split(d)用于获取路径d的目录部分,而os.path.join()则用于将目录部分与空字符串连接起来,得到新的路径字符串。这条语句的作用是获取路径d所在的目录路径。
阅读全文
相关推荐
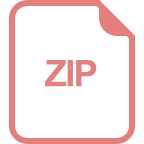
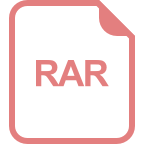















