MATLAB建立评价模型怎么建
时间: 2023-08-24 08:03:50 浏览: 176
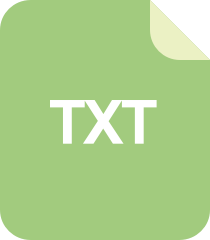
云模型的MATLAB实现
在MATLAB中,建立评价模型的具体方法取决于评价模型的类型和数据集的特点。下面我将介绍一个简单的评价模型建立的流程,供参考。
假设我们的数据集包含2个特征和1个输出变量,我们要建立一个评价模型来预测输出变量的值。我们可以按照以下步骤进行建模:
1. 导入数据集。我们可以使用MATLAB的`readtable`函数来导入数据集,例如:
```matlab
data = readtable('data.csv');
```
2. 数据预处理。我们需要对数据进行预处理,例如去掉缺失值、归一化等操作。这些操作可以使用MATLAB的函数来完成,例如`rmmissing`和`normalize`函数。
3. 划分训练集和测试集。我们需要将数据集随机划分为训练集和测试集,例如:
```matlab
cv = cvpartition(size(data,1),'HoldOut',0.3);
idx = cv.test;
dataTrain = data(~idx,:);
dataTest = data(idx,:);
```
这里我们将数据集划分为70%的训练集和30%的测试集。
4. 建立评价模型。根据数据集的特点和建模目的,我们可以选择不同的评价模型。例如,如果输出变量是连续值,我们可以选择线性回归、支持向量回归等模型;如果输出变量是离散值,我们可以选择分类模型,如决策树、SVM等。
以线性回归模型为例,我们可以使用MATLAB的`fitlm`函数来建立模型,例如:
```matlab
mdl = fitlm(dataTrain,'OutputVariable ~ InputVariable1 + InputVariable2');
```
这里我们假设输出变量为`OutputVariable`,输入变量为`InputVariable1`和`InputVariable2`。
5. 评估模型性能。我们需要使用测试集数据来评估模型的性能,例如计算预测误差、确定系数等指标。这些指标可以使用MATLAB的函数来计算,例如`predict`和`rsquared`函数。
```matlab
yTest = dataTest.OutputVariable;
yPred = predict(mdl,dataTest);
r2 = rsquared(yTest,yPred);
```
这里`yTest`是测试集的输出变量,`yPred`是模型的预测输出变量,`r2`是确定系数。
通过以上步骤,我们可以建立一个简单的评价模型并评估其性能。当然,具体建模过程还需要考虑数据集的特点和建模目的,需要根据实际情况进行调整。
阅读全文
相关推荐



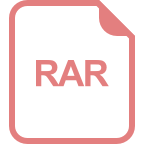
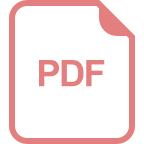
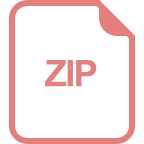
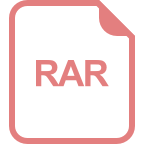
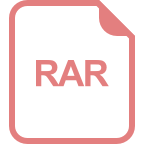
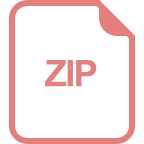
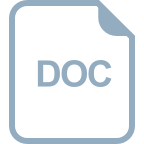
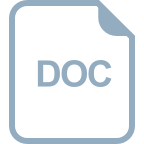
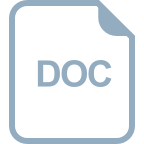
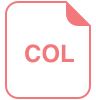


