fast maximum likelihood
时间: 2023-10-29 15:05:00 浏览: 42
Fast maximum likelihood is a method used in statistics and machine learning to estimate the parameters of a probability distribution that best fit a given set of data. The maximum likelihood estimation (MLE) method involves finding the values of the parameters that maximize the likelihood function, which is the probability of observing the given data under the assumed probability distribution.
Fast maximum likelihood methods are those that are computationally efficient and can quickly converge to the optimal solution. Some examples of fast MLE algorithms include the Expectation-Maximization (EM) algorithm, the Newton-Raphson algorithm, and the Quasi-Newton algorithm. These algorithms can be used to estimate the parameters of various probability distributions, such as the Gaussian, Poisson, and exponential distributions.
Fast MLE is particularly useful in large-scale data analysis, where the amount of data can be massive and the computation time for traditional MLE methods can be prohibitive. By using fast MLE algorithms, it is possible to estimate the parameters of a probability distribution in a timely and efficient manner, allowing for faster and more accurate data analysis.
相关推荐
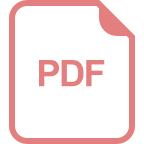
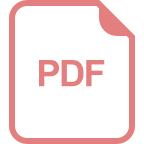














