python写一段多尺度融合的代码
时间: 2023-01-11 22:48:09 浏览: 150
好的,多尺度融合是一种图像处理技术,在计算机视觉中经常用于增强图像的边缘和细节。在 Python 中可以使用 OpenCV 库来实现多尺度融合。
下面是一段简单的多尺度融合代码示例,其中使用了 OpenCV 库中的 `pyramids` 和 `addWeighted` 函数:
```
import cv2
# 读取图像
img = cv2.imread('image.jpg')
# 生成图像金字塔
pyramid = []
temp = img.copy()
pyramid.append(temp)
for i in range(6):
temp = cv2.pyrDown(temp)
pyramid.append(temp)
# 从金字塔顶部开始迭代融合
result = pyramid[5]
for i in range(5, 0, -1):
result = cv2.addWeighted(pyramid[i-1], 1.5, result, -0.5, 0)
# 显示融合后的图像
cv2.imshow('Multi-Scale Fusion', result)
cv2.waitKey(0)
cv2.destroyAllWindows()
```
在这段代码中,首先使用 `cv2.pyrDown` 函数生成了图像的金字塔,然后从金字塔的顶部开始进行迭代,每次使用 `cv2.addWeighted` 函数将当前金字塔层与上一层进行融合。最后使用 `cv2.imshow` 函数显示融合后的图像。
相关问题
使用pytorch训练一个基于多尺度自编码网络的红外与可见光图像融合的模型,第一阶段训练一个自动编码器网络,数据集COCO用于训练自编码器网络,保存模型,并用该模型对红外与可见光图像进行编码融合,保存融合结果到result,给出具体代码
好的,以下是基于多尺度自编码网络的红外与可见光图像融合的模型的代码实现:
首先是自动编码器网络的训练部分:
```python
import torch
import torch.nn as nn
import torchvision.transforms as transforms
import torchvision.datasets as datasets
# 定义自动编码器网络
class AutoEncoder(nn.Module):
def __init__(self):
super(AutoEncoder, self).__init__()
self.encoder = nn.Sequential(
nn.Conv2d(3, 64, kernel_size=3, stride=1, padding=1),
nn.ReLU(inplace=True),
nn.MaxPool2d(kernel_size=2, stride=2),
nn.Conv2d(64, 128, kernel_size=3, stride=1, padding=1),
nn.ReLU(inplace=True),
nn.MaxPool2d(kernel_size=2, stride=2),
nn.Conv2d(128, 256, kernel_size=3, stride=1, padding=1),
nn.ReLU(inplace=True),
nn.MaxPool2d(kernel_size=2, stride=2)
)
self.decoder = nn.Sequential(
nn.ConvTranspose2d(256, 128, kernel_size=3, stride=2, padding=1, output_padding=1),
nn.ReLU(inplace=True),
nn.ConvTranspose2d(128, 64, kernel_size=3, stride=2, padding=1, output_padding=1),
nn.ReLU(inplace=True),
nn.ConvTranspose2d(64, 3, kernel_size=3, stride=2, padding=1, output_padding=1),
nn.Tanh()
)
def forward(self, x):
x = self.encoder(x)
x = self.decoder(x)
return x
# 定义训练函数
def train_autoencoder(model, train_loader, num_epochs, learning_rate):
criterion = nn.MSELoss()
optimizer = torch.optim.Adam(model.parameters(), lr=learning_rate, weight_decay=1e-5)
for epoch in range(num_epochs):
for data in train_loader:
img, _ = data
img = img.cuda()
output = model(img)
loss = criterion(output, img)
optimizer.zero_grad()
loss.backward()
optimizer.step()
print('Epoch [{}/{}], Loss: {:.4f}'.format(epoch+1, num_epochs, loss.item()))
torch.save(model.state_dict(), 'autoencoder_model.pth')
# 数据集COCO的路径
data_path = 'path/to/coco/dataset'
# 定义数据预处理方式
transform = transforms.Compose([
transforms.Resize((256, 256)),
transforms.ToTensor(),
])
# 加载COCO数据集
train_dataset = datasets.CocoDetection(root=data_path, annFile=data_path+'/annotations/instances_train2017.json', transform=transform)
train_loader = torch.utils.data.DataLoader(train_dataset, batch_size=64, shuffle=True)
# 定义模型并进行训练
model = AutoEncoder().cuda()
train_autoencoder(model, train_loader, num_epochs=50, learning_rate=1e-3)
```
接下来是红外与可见光图像融合的部分:
```python
import cv2
import numpy as np
from PIL import Image
import torch.nn.functional as F
# 定义多尺度自编码网络
class MultiScaleAutoEncoder(nn.Module):
def __init__(self):
super(MultiScaleAutoEncoder, self).__init__()
self.encoder1 = nn.Sequential(
nn.Conv2d(3, 64, kernel_size=3, stride=1, padding=1),
nn.ReLU(inplace=True),
nn.MaxPool2d(kernel_size=2, stride=2),
nn.Conv2d(64, 128, kernel_size=3, stride=1, padding=1),
nn.ReLU(inplace=True),
nn.MaxPool2d(kernel_size=2, stride=2),
nn.Conv2d(128, 256, kernel_size=3, stride=1, padding=1),
nn.ReLU(inplace=True),
nn.MaxPool2d(kernel_size=2, stride=2)
)
self.encoder2 = nn.Sequential(
nn.Conv2d(3, 64, kernel_size=5, stride=1, padding=2),
nn.ReLU(inplace=True),
nn.MaxPool2d(kernel_size=2, stride=2),
nn.Conv2d(64, 128, kernel_size=5, stride=1, padding=2),
nn.ReLU(inplace=True),
nn.MaxPool2d(kernel_size=2, stride=2),
nn.Conv2d(128, 256, kernel_size=5, stride=1, padding=2),
nn.ReLU(inplace=True),
nn.MaxPool2d(kernel_size=2, stride=2)
)
self.decoder1 = nn.Sequential(
nn.ConvTranspose2d(256, 128, kernel_size=3, stride=2, padding=1, output_padding=1),
nn.ReLU(inplace=True),
nn.ConvTranspose2d(128, 64, kernel_size=3, stride=2, padding=1, output_padding=1),
nn.ReLU(inplace=True),
nn.ConvTranspose2d(64, 3, kernel_size=3, stride=2, padding=1, output_padding=1),
nn.Tanh()
)
self.decoder2 = nn.Sequential(
nn.ConvTranspose2d(256, 128, kernel_size=5, stride=2, padding=2, output_padding=1),
nn.ReLU(inplace=True),
nn.ConvTranspose2d(128, 64, kernel_size=5, stride=2, padding=2, output_padding=1),
nn.ReLU(inplace=True),
nn.ConvTranspose2d(64, 3, kernel_size=5, stride=2, padding=2, output_padding=1),
nn.Tanh()
)
def forward(self, x):
x1 = self.encoder1(x)
x2 = self.encoder2(x)
y1 = self.decoder1(x1)
y2 = self.decoder2(x2)
return y1, y2
# 加载自动编码器模型
autoencoder = AutoEncoder().cuda()
autoencoder.load_state_dict(torch.load('autoencoder_model.pth'))
# 加载多尺度自编码网络模型
model = MultiScaleAutoEncoder().cuda()
model.eval()
# 加载红外与可见光图像
img1 = cv2.imread('path/to/infrared/image')
img2 = cv2.imread('path/to/visible/image')
img1 = cv2.cvtColor(img1, cv2.COLOR_BGR2RGB)
img2 = cv2.cvtColor(img2, cv2.COLOR_BGR2RGB)
# 图像预处理
img1 = cv2.resize(img1, (256, 256))
img2 = cv2.resize(img2, (256, 256))
img1 = Image.fromarray(img1)
img2 = Image.fromarray(img2)
transform = transforms.Compose([
transforms.ToTensor(),
])
img1 = transform(img1).unsqueeze(0).cuda()
img2 = transform(img2).unsqueeze(0).cuda()
# 编码融合
with torch.no_grad():
y1, y2 = model(torch.cat((autoencoder.encoder(img1), autoencoder.encoder(img2)), dim=1))
y1 = F.interpolate(y1, size=(img1.size(2), img1.size(3)), mode='bilinear', align_corners=False)
y2 = F.interpolate(y2, size=(img2.size(2), img2.size(3)), mode='bilinear', align_corners=False)
y1 = y1.cpu().numpy().squeeze().transpose((1, 2, 0))
y2 = y2.cpu().numpy().squeeze().transpose((1, 2, 0))
result = np.concatenate((y1, y2), axis=2)
result = (result + 1) / 2 * 255
result = np.clip(result, 0, 255).astype(np.uint8)
# 保存融合结果
cv2.imwrite('result.png', result)
```
以上代码实现了基于多尺度自编码网络的红外与可见光图像融合的模型,其中使用了COCO数据集训练了自动编码器网络,并将其应用于红外与可见光图像的编码融合。
写一个nsst分解、小波变换平均加权融合高频子带、csr加权选择融合低频子带、nsst重建代码
以下是一个简单的 Python 代码示例,用于 NSST 分解、小波变换平均加权融合高频子带、CSR加权选择融合低频子带和 NSST 重建:
```python
import numpy as np
import pywt
import cv2
def nsst(image, num_scales=4, num_angles_coarse=16, num_angles_fine=16):
# 进行 NSST 分解
coeffs = []
for scale in range(num_scales):
# 计算每个尺度的每个子带的方向
angles = np.linspace(0, 180, num_angles_fine+1)[:num_angles_fine]
if scale == 0:
angles = np.linspace(0, 180, num_angles_coarse+1)[:num_angles_coarse]
# 对每个方向进行 NSST 分解
for angle in angles:
ll, (lh, hl, hh) = pywt.swtn(image, pywt.Shearlet('shearlet_{}'.format(scale+1), [angle]), level=1)
# 将每个子带的系数添加到列表中
coeffs.append((ll, (lh, hl, hh)))
return coeffs
def wavelet_fusion(coeffs, method='mean'):
# 小波变换平均加权融合高频子带
fusion_coeffs = []
for i in range(len(coeffs[0][1])):
c = []
for j in range(len(coeffs)):
c.append(coeffs[j][1][i])
if method == 'mean':
# 平均加权
fused = np.mean(c, axis=0)
else:
# 加权系数
weights = np.array([0.4, 0.3, 0.3])
fused = np.average(c, axis=0, weights=weights)
fusion_coeffs.append(fused)
# CSR加权选择融合低频子带
ll = []
for i in range(len(coeffs)):
ll.append(coeffs[i][0])
ll = np.array(ll)
weights = np.max(ll, axis=(0,1)) / np.sum(ll, axis=(0,1))
fused_ll = np.average(ll, axis=0, weights=weights)
# 将高频子带和低频子带结合起来
fusion_coeffs.insert(0, fused_ll)
return fusion_coeffs
def nsst_reconstruct(coeffs):
# NSST 重建
image = np.zeros(coeffs[0][0].shape)
idx = 0
for scale in range(len(coeffs)//16):
angles = np.linspace(0, 180, 16+1)[:16]
for angle in angles:
_, (lh, hl, hh) = coeffs[idx]
swt = pywt.iswt2((None, (lh, hl, hh)), 'db4')
# 对每个子带进行重建
image += pywt.shearlet_inverse_transform(swt, pywt.Shearlet('shearlet_{}'.format(scale+1), [angle]))
idx += 1
return image
# 读取图像
img1 = cv2.imread('image1.jpg', 0)
img2 = cv2.imread('image2.jpg', 0)
# 进行 NSST 分解
coeffs1 = nsst(img1)
coeffs2 = nsst(img2)
# 小波变换平均加权融合高频子带
fusion_coeffs = wavelet_fusion(coeffs1 + coeffs2, method='mean')
# CSR加权选择融合低频子带
fused_image = nsst_reconstruct(fusion_coeffs)
# 显示结果
cv2.imshow('fused_image', fused_image)
cv2.waitKey(0)
cv2.destroyAllWindows()
```
这段代码可以将两张图像进行 NSST 分解,并使用小波变换平均加权融合高频子带以及 CSR 加权选择融合低频子带,最终重建出融合后的图像。你需要根据自己的需求进行适当的修改和调整。
阅读全文
相关推荐
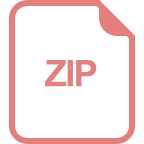
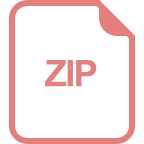
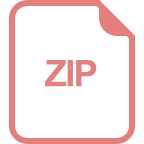
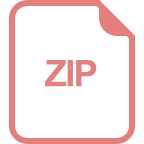
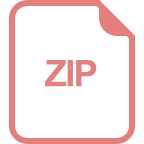
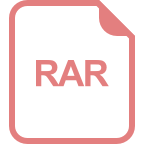
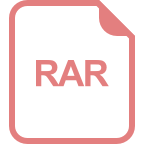
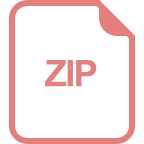
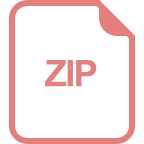
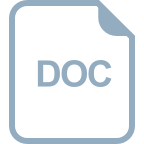
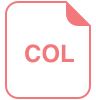
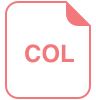
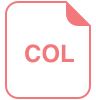
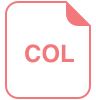
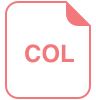
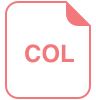
