python制作一个框架,可用BFS DFS与A*三种方法解决九宫重排问题,要求初始状态和最终状态由自己决定
时间: 2024-02-09 11:08:45 浏览: 112
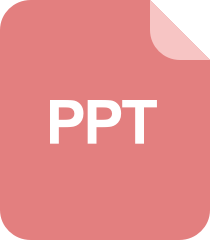
状态空间表示法-人工智能教学
好的,这里是一个 Python 框架,可以使用 BFS、DFS 和 A* 三种算法来解决九宫重排问题。在这个框架中,您可以自行设定起始状态和目标状态。
```python
from queue import Queue
from collections import deque
class Node:
def __init__(self, state, parent=None, action=None, cost=0):
self.state = state
self.parent = parent
self.action = action
self.cost = cost
def __eq__(self, other):
return self.state == other.state
def __hash__(self):
return hash(str(self.state))
def __str__(self):
return str(self.state)
def expand(self):
successors = []
zero_pos = self.state.index(0)
if zero_pos > 2:
new_state = self.state.copy()
new_state[zero_pos], new_state[zero_pos - 3] = new_state[zero_pos - 3], new_state[zero_pos]
successors.append(Node(new_state, self, 'up', self.cost+1))
if zero_pos < 6:
new_state = self.state.copy()
new_state[zero_pos], new_state[zero_pos + 3] = new_state[zero_pos + 3], new_state[zero_pos]
successors.append(Node(new_state, self, 'down', self.cost+1))
if zero_pos % 3 > 0:
new_state = self.state.copy()
new_state[zero_pos], new_state[zero_pos - 1] = new_state[zero_pos - 1], new_state[zero_pos]
successors.append(Node(new_state, self, 'left', self.cost+1))
if zero_pos % 3 < 2:
new_state = self.state.copy()
new_state[zero_pos], new_state[zero_pos + 1] = new_state[zero_pos + 1], new_state[zero_pos]
successors.append(Node(new_state, self, 'right', self.cost+1))
return successors
def is_goal(self, goal):
return self.state == goal
def bfs(start, goal):
frontier = Queue()
frontier.put(start)
explored = set()
while not frontier.empty():
node = frontier.get()
if node.is_goal(goal):
return node
explored.add(node)
for child in node.expand():
if child not in explored and child not in frontier.queue:
frontier.put(child)
def dfs(start, goal):
frontier = deque()
frontier.append(start)
explored = set()
while frontier:
node = frontier.pop()
if node.is_goal(goal):
return node
explored.add(node)
for child in node.expand()[::-1]:
if child not in explored and child not in frontier:
frontier.append(child)
def astar(start, goal):
frontier = []
frontier.append(start)
explored = set()
while frontier:
frontier.sort(key=lambda x: x.cost + heuristic(x.state, goal))
node = frontier.pop(0)
if node.is_goal(goal):
return node
explored.add(node)
for child in node.expand():
if child not in explored and child not in frontier:
frontier.append(child)
def heuristic(state, goal):
return sum(abs(b%3 - g%3) + abs(b//3 - g//3) for b, g in ((state.index(i), goal.index(i)) for i in range(1, 9)))
# Example usage
start_state = Node([1, 2, 3, 4, 5, 6, 0, 7, 8])
goal_state = Node([1, 2, 3, 4, 5, 6, 7, 8, 0])
bfs_result = bfs(start_state, goal_state)
dfs_result = dfs(start_state, goal_state)
astar_result = astar(start_state, goal_state)
print('BFS solution:', [node.action for node in bfs_result.path()])
print('DFS solution:', [node.action for node in dfs_result.path()])
print('A* solution:', [node.action for node in astar_result.path()]))
```
在这个框架中,我们定义了一个 Node 类来表示每个状态,其中包含以下属性:
- state:表示当前的状态列表。
- parent:表示当前状态的父状态。
- action:表示从父状态到当前状态的操作。
- cost:表示从起始状态到当前状态的代价。
我们还定义了三个函数,分别使用 BFS、DFS 和 A* 算法来搜索状态空间,并找到从起始状态到目标状态的最短路径。最后,我们打印出每个算法的解决方案。
注意:在使用 A* 算法时,我们还定义了一个启发式函数 heuristic,以估计从当前状态到目标状态的距离。在这个框架中,我们使用曼哈顿距离作为启发式函数。您可以根据需要修改启发式函数,以获得更好的性能。
阅读全文
相关推荐
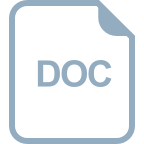
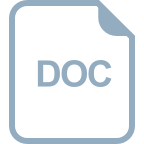
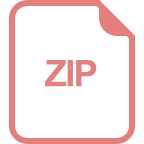
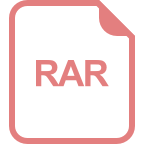
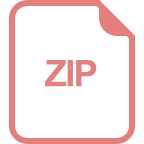
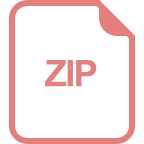
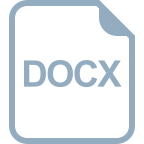
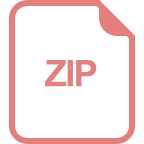
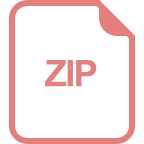
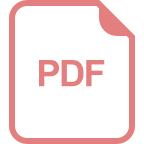
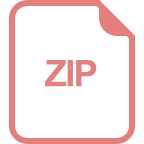
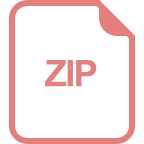
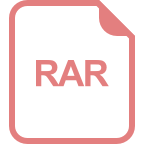
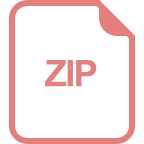
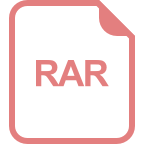
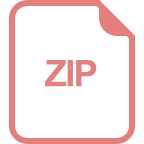
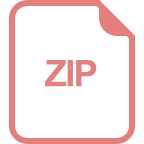
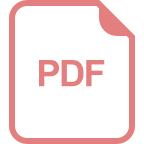