combined_transformation是什么?怎么来的?为什么内参矩阵要求逆
时间: 2024-02-03 16:02:08 浏览: 137
`combined_transformation` 是相机姿态估计中的一个变换矩阵,用于将世界坐标系下的点变换到相机坐标系下。它是由相机的旋转矩阵和平移向量计算得到的。具体地,`combined_transformation` 的计算方式为:
```
combined_transformation = rotation.matmul(torch.inverse(intrinsics))
```
其中,`rotation` 是相机的旋转矩阵,`intrinsics` 是相机内参矩阵。这行代码的作用是将相机的旋转矩阵转换为相机坐标系下的旋转矩阵。
内参矩阵需要求逆的原因是,在将世界坐标系下的点变换到相机坐标系下时,需要将像素坐标系下的点转换到相机坐标系下。而相机内参矩阵描述了像素坐标系和相机坐标系之间的关系,因此需要用到内参矩阵。具体来说,在将像素坐标系下的点变换到相机坐标系下时,需要将点的像素坐标表示为一个齐次坐标(Homogeneous Coordinate),然后用相机内参矩阵的逆矩阵将其转换到相机坐标系下。因此,内参矩阵需要求逆才能进行像素坐标系和相机坐标系之间的转换。
相关问题
combined_transformation = rotation.matmul(torch.inverse(intrinsics))是什么意思?
这段代码是在进行相机姿态估计时使用的。其中,`intrinsics` 是相机内参矩阵,`rotation` 是相机的旋转矩阵。这行代码的作用是:将相机旋转矩阵转换为相机坐标系下的旋转矩阵。具体来说,它首先通过 `torch.inverse(intrinsics)` 计算出相机坐标系下的投影矩阵,然后用该投影矩阵左乘相机旋转矩阵 `rotation`,得到的结果 `combined_transformation` 就是相机坐标系下的旋转矩阵。
def get_geometry(self, intrinsics, extrinsics): """Calculate the (x, y, z) 3D position of the features. """ rotation, translation = extrinsics[..., :3, :3], extrinsics[..., :3, 3] B, N, _ = translation.shape # Add batch, camera dimension, and a dummy dimension at the end points = self.frustum.unsqueeze(0).unsqueeze(0).unsqueeze(-1) # Camera to ego reference frame points = torch.cat((points[:, :, :, :, :, :2] * points[:, :, :, :, :, 2:3], points[:, :, :, :, :, 2:3]), 5) combined_transformation = rotation.matmul(torch.inverse(intrinsics)) points = combined_transformation.view(B, N, 1, 1, 1, 3, 3).matmul(points).squeeze(-1) points += translation.view(B, N, 1, 1, 1, 3) # The 3 dimensions in the ego reference frame are: (forward, sides, height) return points这段代码的意思是什么?
这段代码是一个用于将二维图像特征点映射到三维空间中的函数。它接收相机内参和外参作为输入,并返回每个特征点的三维坐标。
在函数中,首先从外参中提取旋转和平移矩阵。然后将输入的二维特征点扩展成一个四维张量,其中第一维是batch size,第二维是相机数量,第三维是特征点数量,第四维是x、y和一个占位的维度。
接下来,通过一系列的数学运算将特征点从相机坐标系转换到自车坐标系。这里的转换包括以下几个步骤:
1. 将二维特征点的前两个维度(x和y)乘以深度(z)并将其放在新的第三个维度中,这样就得到了三维特征点的相机坐标。
2. 将相机坐标转换为自车参考系下的坐标。这里使用了相机内参矩阵和旋转矩阵进行计算。
3. 将平移矩阵加到每个特征点的位置上,以获得相对于自车坐标系的绝对位置。
最后,返回每个特征点的三维坐标。
阅读全文
相关推荐
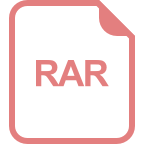
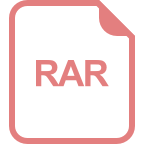
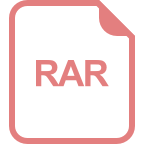
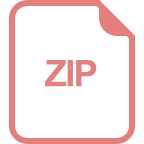
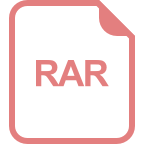
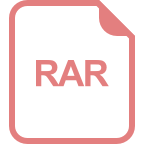
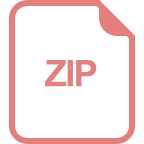
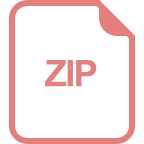
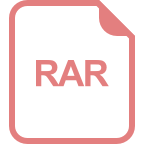
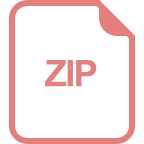
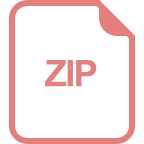
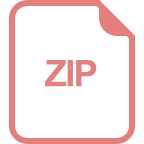
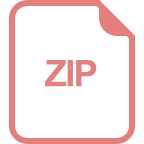
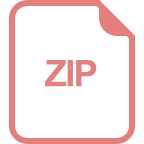
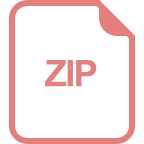