resnet50对cifar10分类
时间: 2023-09-27 18:04:28 浏览: 40
ResNet50是一种深度卷积神经网络,通常用于图像分类任务,它包含50个卷积层和全连接层。CIFAR-10是一个包含10个类别的图像分类数据集,每个类别有6000张32x32像素的彩色图像。由于CIFAR-10图像尺寸较小,通常使用较浅的卷积神经网络进行分类。
尝试使用ResNet50对CIFAR-10进行分类通常会面临两个问题。首先,CIFAR-10图像尺寸较小,ResNet50的输入尺寸为224x224,需要对图像进行缩放处理。其次,CIFAR-10数据集相对较小,使用ResNet50容易出现过拟合问题,需要使用数据增强和正则化等技术进行优化。
因此,对于CIFAR-10分类任务,通常使用轻量级的卷积神经网络如LeNet、AlexNet或VGG等进行分类,这些模型具有较少的参数和计算量,可以更好地适应CIFAR-10数据集。
相关问题
resnet50训练cifar10
根据提供的引用内容,可以得知以下信息:
1. 引用提供了使用Pytorch实现ResNet50模型对CIFAR-100数据集进行训练的代码,其中包括数据集的下载、模型的训练、损失函数的选择和优化器的使用等内容。
2. 引用提供了训练时的一些参数设置,包括训练次数、批次大小、分类类别数量、学习率大小等。
3. 引用提供了使用ResNet对CIFAR-10数据集进行测试的一些经验,包括将数据集分辨率扩大到32X32或224X224等。
综上所述,如果想要使用ResNet50模型对CIFAR-10数据集进行训练,可以参考引用中的代码,并根据实际情况进行参数设置和数据预处理。同时,可以参考引用中的经验,对数据集进行适当的处理,以提高模型的训练效果和识别准确率。
resnet18实现cifar10分类
下面是一个使用ResNet-18模型实现CIFAR-10分类的示例代码:
```python
import torch
import torchvision
import torchvision.transforms as transforms
import torch.nn as nn
import torch.optim as optim
from torch.utils.data import DataLoader
# 数据预处理
transform_train = transforms.Compose([
transforms.RandomCrop(32, padding=4),
transforms.RandomHorizontalFlip(),
transforms.ToTensor(),
transforms.Normalize((0.5, 0.5, 0.5), (0.5, 0.5, 0.5))
])
transform_test = transforms.Compose([
transforms.ToTensor(),
transforms.Normalize((0.5, 0.5, 0.5), (0.5, 0.5, 0.5))
])
# 加载CIFAR-10数据集
trainset = torchvision.datasets.CIFAR10(root='./data', train=True, download=True, transform=transform_train)
trainloader = DataLoader(trainset, batch_size=128, shuffle=True, num_workers=2)
testset = torchvision.datasets.CIFAR10(root='./data', train=False, download=True, transform=transform_test)
testloader = DataLoader(testset, batch_size=100, shuffle=False, num_workers=2)
# 定义ResNet-18模型
class ResNet(nn.Module):
def __init__(self):
super(ResNet, self).__init__()
self.resnet18 = torchvision.models.resnet18(pretrained=False)
self.fc = nn.Linear(1000, 10) # CIFAR-10有10个类别
def forward(self, x):
x = self.resnet18(x)
x = self.fc(x)
return x
model = ResNet()
# 定义损失函数和优化器
criterion = nn.CrossEntropyLoss()
optimizer = optim.SGD(model.parameters(), lr=0.001, momentum=0.9)
# 训练模型
device = torch.device("cuda" if torch.cuda.is_available() else "cpu")
model.to(device)
for epoch in range(10):
running_loss = 0.0
for i, data in enumerate(trainloader, 0):
inputs, labels = data
inputs, labels = inputs.to(device), labels.to(device)
optimizer.zero_grad()
outputs = model(inputs)
loss = criterion(outputs, labels)
loss.backward()
optimizer.step()
running_loss += loss.item()
if i % 200 == 199:
print('[%d, %5d] loss: %.3f' % (epoch + 1, i + 1, running_loss / 200))
running_loss = 0.0
print('Finished Training')
# 在测试集上评估模型
correct = 0
total = 0
with torch.no_grad():
for data in testloader:
images, labels = data
images, labels = images.to(device), labels.to(device)
outputs = model(images)
_, predicted = torch.max(outputs.data, 1)
total += labels.size(0)
correct += (predicted == labels).sum().item()
print('Accuracy on the test images: %d %%' % (100 * correct / total))
```
这段代码使用了PyTorch框架,首先定义了数据预处理的方式,然后使用`torchvision.datasets.CIFAR10`加载CIFAR-10数据集,并使用`torch.utils.data.DataLoader`创建数据加载器。接着定义了一个基于ResNet-18的模型,包含一个全连接层用于分类。然后定义了损失函数和优化器。最后,使用训练数据对模型进行训练,并在测试集上评估模型的准确率。
相关推荐
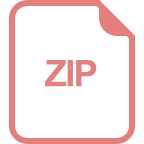
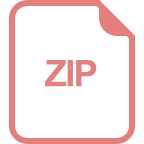
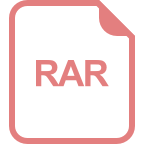












