from sklearn.ensemble import GradientBoostingRegressor
时间: 2023-11-18 21:06:34 浏览: 98
这是一个引入 scikit-learn 库中的 GradientBoostingRegressor 模块的操作。GradientBoostingRegressor 是一种基于梯度提升树(Gradient Boosting Tree)的回归算法,它能够解决回归问题,并且在处理非线性关系和高维数据方面表现良好。
具体来说,GradientBoostingRegressor 通过迭代地训练多棵树,不断地提高模型的预测能力。在每一轮迭代中,算法会根据当前模型的残差(即实际值与预测值之间的差异)拟合一棵新的树,并且将这棵树的预测结果加到原来的模型中。这样,模型就可以逐步逼近真实的函数关系,从而获得更好的预测效果。
GradientBoostingRegressor 模块提供了许多参数来控制算法的行为,如学习率、树的个数、树的深度等,可以根据具体情况进行调整。
相关问题
解释这段代码import numpy as np import pandas as pd from datetime import datetime from scipy.stats import skew from scipy.special import boxcox1p from scipy.stats import boxcox_normmax from sklearn.linear_model import ElasticNetCV, LassoCV, RidgeCV, Ridge from sklearn.ensemble import GradientBoostingRegressor from sklearn.svm import SVR from sklearn.pipeline import make_pipeline from sklearn.preprocessing import RobustScaler, StandardScaler from sklearn.model_selection import KFold, cross_val_score from sklearn.metrics import mean_squared_error as mse from sklearn.metrics import make_scorer from sklearn.neighbors import LocalOutlierFactor from sklearn.linear_model import LinearRegression from mlxtend.regressor import StackingCVRegressor # from xgboost import XGBRegressor # from lightgbm import LGBMRegressor import matplotlib.pyplot as plt import seaborn as sns
这段代码是在Python中导入所需要的库和模块。具体解释如下:
- `import numpy as np`:导入NumPy库,并将其简写为np,以方便使用。
- `import pandas as pd`:导入Pandas库,并将其简写为pd,以方便使用。
- `from datetime import datetime`:从datetime模块中导入datetime函数,用于处理时间数据。
- `from scipy.stats import skew`:从scipy.stats模块中导入skew函数,用于计算数据的偏度。
- `from scipy.special import boxcox1p`:从scipy.special模块中导入boxcox1p函数,用于进行Box-Cox变换。
- `from scipy.stats import boxcox_normmax`:从scipy.stats模块中导入boxcox_normmax函数,用于计算Box-Cox变换的参数。
- `from sklearn.linear_model import ElasticNetCV, LassoCV, RidgeCV, Ridge`:从sklearn.linear_model模块中导入ElasticNetCV、LassoCV、RidgeCV、Ridge等函数,用于进行线性回归。
- `from sklearn.ensemble import GradientBoostingRegressor`:从sklearn.ensemble模块中导入GradientBoostingRegressor函数,用于进行梯度提升回归。
- `from sklearn.svm import SVR`:从sklearn.svm模块中导入SVR函数,用于进行支持向量回归。
- `from sklearn.pipeline import make_pipeline`:从sklearn.pipeline模块中导入make_pipeline函数,用于构建机器学习管道。
- `from sklearn.preprocessing import RobustScaler, StandardScaler`:从sklearn.preprocessing模块中导入RobustScaler、StandardScaler函数,用于进行特征缩放。
- `from sklearn.model_selection import KFold, cross_val_score`:从sklearn.model_selection模块中导入KFold、cross_val_score函数,用于进行交叉验证。
- `from sklearn.metrics import mean_squared_error as mse`:从sklearn.metrics模块中导入mean_squared_error函数,并将其简写为mse,用于计算均方误差。
- `from sklearn.metrics import make_scorer`:从sklearn.metrics模块中导入make_scorer函数,用于创建自定义评分函数。
- `from sklearn.neighbors import LocalOutlierFactor`:从sklearn.neighbors模块中导入LocalOutlierFactor函数,用于检测异常值。
- `from sklearn.linear_model import LinearRegression`:从sklearn.linear_model模块中导入LinearRegression函数,用于进行线性回归。
- `from mlxtend.regressor import StackingCVRegressor`:从mlxtend.regressor模块中导入StackingCVRegressor函数,用于进行交叉验证的堆叠模型。
- `import matplotlib.pyplot as plt`:导入matplotlib库,并将其简写为plt,用于绘制图形。
- `import seaborn as sns`:导入seaborn库,并将其简写为sns,用于绘制图形。
from sklearn.model_selection import train_test_split, KFold, cross_val_score from sklearn.linear_model import LinearRegression, Ridge, Lasso from sklearn.neighbors import KNeighborsRegressor from sklearn.svm import LinearSVR, SVR from sklearn.tree import DecisionTreeRegressor from sklearn.ensemble import GradientBoostingRegressor,RandomForestRegressor,BaggingRegressor from xgboost import XGBRegressor # from lightgbm import LGBMRegressor from catboost import CatBoostRegressor models = { " Linear Regression": LinearRegression(), " Linear Regression (L2 Regularization)": Ridge(), " Linear Regression (L1 Regularization)": Lasso(), " K-Nearest Neighbors": KNeighborsRegressor(), "Support Vector Machine (Linear Kernel)": LinearSVR(), " Support Vector Machine (RBF Kernel)": SVR(), " Decision Tree": DecisionTreeRegressor(), " Random Forest": RandomForestRegressor(), " Gradient Boosting": GradientBoostingRegressor(loss='absolute_error'), " XGBoost": XGBRegressor(), " LightGBM": LGBMRegressor(), " CatBoost": CatBoostRegressor(verbose=0) }
这段代码是一个 Python 字典,其中包含了多个回归模型,使用了不同的 Python 库来实现。其中包括了线性回归(Linear Regression)、带 L2 正则化的线性回归(Ridge)、带 L1 正则化的线性回归(Lasso)、K 近邻回归(KNeighborsRegressor)、线性支持向量机回归(LinearSVR)、径向基函数支持向量机回归(SVR)、决策树回归(DecisionTreeRegressor)、随机森林回归(RandomForestRegressor)、梯度提升回归(GradientBoostingRegressor)、XGBoost 回归(XGBRegressor)、LightGBM 回归(LGBMRegressor)和 CatBoost 回归(CatBoostRegressor)等多个模型。这些模型可以用于回归预测任务,每个模型都有不同的优缺点和适用场景。该代码将这些模型都定义在了一个字典中,方便进行调用和比较。同时,该代码还引入了一些常用的模型选择和评估方法,如数据集划分(train_test_split)、K 折交叉验证(KFold)、交叉验证评分(cross_val_score)等。
阅读全文
相关推荐
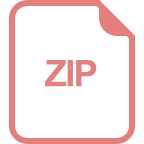
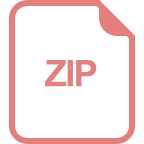













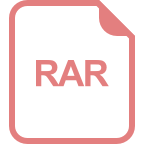