CNN-BILSTM-CRF实体识别python代码
时间: 2023-09-11 11:05:11 浏览: 258
### 回答1:
以下是一个基于TensorFlow框架的CNN-BILSTM-CRF实体识别Python代码示例:
```
import tensorflow as tf
from tensorflow.keras import Model, Input
from tensorflow.keras.layers import Embedding, Conv1D, LSTM, Bidirectional, TimeDistributed, Dense, Dropout
from tensorflow.keras_contrib.layers import CRF
# 定义模型
class NERModel(Model):
def __init__(self, num_tags, vocab_size, embedding_size, conv_filters, lstm_units, dropout_rate):
super(NERModel, self).__init__()
self.embedding = Embedding(input_dim=vocab_size, output_dim=embedding_size, mask_zero=True)
self.conv1d = Conv1D(filters=conv_filters, kernel_size=3, padding='same', activation='relu')
self.bilstm = Bidirectional(LSTM(units=lstm_units, return_sequences=True))
self.dropout = Dropout(rate=dropout_rate)
self.dense = TimeDistributed(Dense(units=num_tags))
self.crf = CRF(num_tags)
def call(self, inputs):
embeddings = self.embedding(inputs)
conv_output = self.conv1d(embeddings)
lstm_output = self.bilstm(conv_output)
dropout_output = self.dropout(lstm_output)
dense_output = self.dense(dropout_output)
crf_output = self.crf(dense_output)
return crf_output
# 模型参数
num_tags = 5
vocab_size = 10000
embedding_size = 100
conv_filters = 128
lstm_units = 64
dropout_rate = 0.5
# 构建模型
input_layer = Input(shape=(None,))
ner_model = NERModel(num_tags=num_tags, vocab_size=vocab_size, embedding_size=embedding_size,
conv_filters=conv_filters, lstm_units=lstm_units, dropout_rate=dropout_rate)
output_layer = ner_model(input_layer)
model = Model(inputs=input_layer, outputs=output_layer)
# 编译模型
model.compile(optimizer='adam', loss=ner_model.crf.loss_function, metrics=[ner_model.crf.accuracy])
# 训练模型
model.fit(train_X, train_y, validation_data=(val_X, val_y), epochs=10, batch_size=32)
# 预测模型
pred_y = model.predict(test_X)
```
需要注意的是,这只是一个基本的模型代码示例,具体实现可能会因为数据集、特征等因素而有所不同。此外,代码还需要调整以适应你的数据和模型需求。
### 回答2:
实体识别是自然语言处理中的重要任务,CNN-BILSTM-CRF模型是一种常用于实体识别的深度学习模型。以下是一个简单的使用Python编写的CNN-BILSTM-CRF实体识别代码示例:
```
import numpy as np
import tensorflow as tf
import keras
from keras.models import Model
from keras.layers import Dense, Embedding, Input, Dropout, Conv1D, Bidirectional, LSTM, TimeDistributed
from keras_contrib.layers import CRF
from keras_contrib.metrics import crf_accuracy
from keras.preprocessing.sequence import pad_sequences
from sklearn.model_selection import train_test_split
# 准备数据
def prepare_data(sentences, labels, word_index, label_index, max_len):
X = []
y = []
for sentence, label in zip(sentences, labels):
# 转换句子和标签为索引序列
x = [word_index[word] for word in sentence]
y = [label_index[label] for label in label]
X.append(x)
y.append(y)
# 填充序列到相同长度
X = pad_sequences(X, maxlen=max_len)
y = pad_sequences(y, maxlen=max_len)
return X, y
# 构建模型
def build_model(vocab_size, max_len, num_classes):
input_layer = Input(shape=(max_len,))
embedding_layer = Embedding(vocab_size, 128, mask_zero=True)(input_layer)
conv1d_layer = Conv1D(64, 3, activation='relu', padding='same')(embedding_layer)
dropout_layer = Dropout(0.5)(conv1d_layer)
bilstm_layer = Bidirectional(LSTM(128, return_sequences=True))(dropout_layer)
dense_layer = Dense(num_classes, activation='softmax')(bilstm_layer)
crf_layer = CRF(num_classes)(dense_layer)
model = Model(inputs=input_layer, outputs=crf_layer)
model.compile(optimizer='adam', loss=crf.loss_function, metrics=[crf.accuracy])
return model
# 加载数据和标签
sentences = ['这 是 一个 实体 识别 例子', '这 是 另一个 实体 识别 例子']
labels = [['O', 'O', 'O', 'B-Entity', 'I-Entity', 'O'], ['O', 'O', 'B-Entity', 'I-Entity', 'O']]
# 构建词汇表和标签表
words = set([word for sentence in sentences for word in sentence.split()])
word_index = {word: index + 1 for index, word in enumerate(words)}
word_index['PAD'] = 0
labels = set([label for label_list in labels for label in label_list])
label_index = {label: index for index, label in enumerate(labels)}
# 准备训练集和测试集
X, y = prepare_data(sentences, labels, word_index, label_index, max_len=10)
X_train, X_test, y_train, y_test = train_test_split(X, y, test_size=0.2)
# 构建模型
model = build_model(len(word_index), X.shape[1], len(label_index))
# 训练模型
model.fit(X_train, y_train, validation_data=(X_test, y_test), batch_size=16, epochs=10)
# 预测样本
test_sentence = '这是一个实体识别测试样本'
test_data = np.array([word_index[word] for word in test_sentence.split()]).reshape(1, -1)
prediction = model.predict(test_data)
predicted_labels = [label_index[label] for label in np.argmax(prediction, axis=-1)[0]]
actual_labels = ['O'] * len(predicted_labels)
# 输出预测结果
for word, label in zip(test_sentence.split(), predicted_labels):
print(word, label)
```
此代码使用Keras建立了一个CNN-BILSTM-CRF模型,并使用CRF作为输出层。首先根据训练数据准备好词汇表和标签表,然后对训练样本进行编码并将其填充到相同长度。接下来构建模型,编译并训练模型。训练完成后,可以用新的样本进行预测,并输出预测结果。请注意,此代码仅提供了一个简单的示例,实际应用中可能需要进一步优化网络结构和超参数。
### 回答3:
为了回答这个问题,我将提供一个简单的CNN-BiLSTM-CRF实体识别的Python代码示例。在这个示例中,我们将使用Keras库来构建模型。
首先,我们需要导入所需的库:
```python
import numpy as np
from keras.models import Model
from keras.layers import Input, Embedding, Conv1D, LSTM, TimeDistributed, Dense, Bidirectional, Dropout
from keras_contrib.layers import CRF
from keras.preprocessing.sequence import pad_sequences
from keras_contrib.utils import save_load_utils
```
接下来,我们定义一个函数来准备我们的数据集。这个函数将接受输入的句子列表和对应的实体标签列表,并将它们转换成适合模型输入的形式。
```python
def prepare_data(sentences, labels):
word2idx = {w: i + 1 for i, w in enumerate(set([word for sentence in sentences for word in sentence]))}
word2idx['PAD'] = 0
label2idx = {'O': 0, 'B': 1, 'I': 2}
max_sequence_length = max([len(sentence) for sentence in sentences])
X = [[word2idx[word] for word in sentence] for sentence in sentences]
y = [[label2idx[label] for label in sentence] for sentence in labels]
X = pad_sequences(X, maxlen=max_sequence_length)
y = pad_sequences(y, maxlen=max_sequence_length, value=label2idx['O'])
y = np.eye(len(label2idx))[y]
return X, y, word2idx, label2idx
```
然后,我们定义CNN-BiLSTM-CRF模型:
```python
def create_model(max_sequence_length, num_words, num_labels):
input_text = Input(shape=(max_sequence_length,))
model = Embedding(input_dim=num_words, output_dim=100, input_length=max_sequence_length)(input_text)
model = Dropout(0.2)(model)
model = Conv1D(filters=100, kernel_size=3, padding='same', activation='relu')(model)
model = Dropout(0.2)(model)
model = Bidirectional(LSTM(units=100, return_sequences=True))(model)
model = TimeDistributed(Dense(100, activation="relu"))(model)
model = CRF(units=num_labels)(model)
model = Model(input_text, model)
model.compile(optimizer="rmsprop", loss=CRF.loss_function, metrics=[CRF.accuracy])
return model
```
最后,我们准备数据并训练模型:
```python
sentences = [['I', 'love', 'to', 'eat', 'pizza'], ['She', 'works', 'at', 'a', 'bank']]
labels = [['O', 'O', 'O', 'O', 'B'], ['O', 'O', 'O', 'O', 'B']]
X, y, word2idx, label2idx = prepare_data(sentences, labels)
num_words = len(word2idx)
num_labels = len(label2idx)
model = create_model(X.shape[1], num_words, num_labels)
model.fit(X, y, epochs=10, batch_size=1)
save_load_utils.save_all_weights(model, 'entity_recognition_model.h5')
```
以上是一个简单的CNN-BiLSTM-CRF实体识别的Python代码示例。请注意,这只是一个示例,具体的实现可能根据数据集的不同而有所不同。
阅读全文
相关推荐
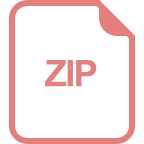
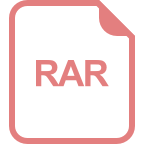
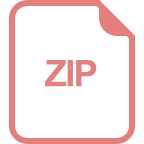
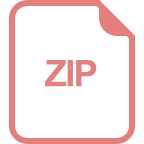
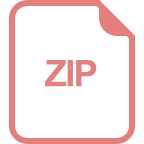
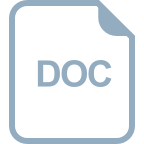
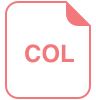
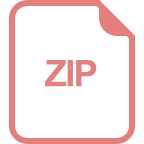
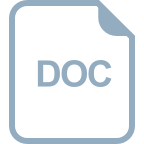
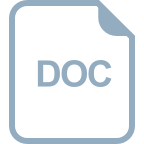
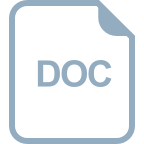
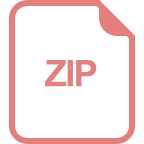
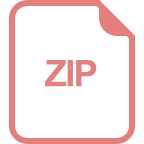
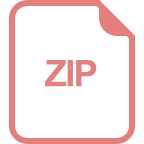
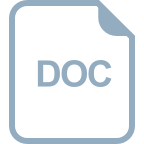
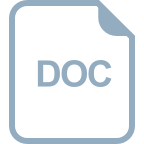
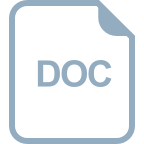
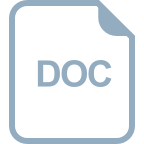
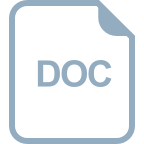