After updating volumes, found 3 active roots
时间: 2024-04-20 21:24:14 浏览: 112
"After updating volumes, found 3 active roots" 这个信息可能是在使用Android设备时出现的。它表示在更新存储卷(volumes)后,发现了3个活动的根目录(active roots)。
在Android系统中,存储卷是指用于存储数据的物理或逻辑储存区域,而根目录是存储卷的顶级目录,通常以斜杠(/)表示。
这个信息可能是由于更新了存储卷的配置或添加了新的存储卷导致的。活动的根目录表示系统当前正在使用的根目录。
如果您遇到这个信息,您可以考虑以下几点:
1. 检查存储卷的配置:确保存储卷的配置正确,并且没有冲突或错误的设置。
2. 检查存储卷的状态:确认所有存储卷都处于正确的状态,并且没有任何故障或问题。
3. 检查根目录的使用情况:查看每个活动的根目录的使用情况,确保它们没有超过容量限制或出现异常情况。
如果您不确定如何处理这个问题,建议您参考设备制造商提供的文档或联系技术支持获取更多帮助。
相关问题
Note that weights smaller than a threshold (0.0001) are zeroed out after updating weights
这句话的意思是,当权重更新后,所有小于0.0001的权重值会被置为零。这个操作通常被称为权重修剪(Weight Pruning),主要用于减少神经网络中的参数量,以达到模型压缩和加速的目的。在神经网络中,一些权重值非常接近于零,有时甚至可以被认为是无用的权重,因为它们对模型的预测准确性没有太大贡献。因此,将这些小于阈值的权重值置为零,可以减少模型中的参数数量,从而降低模型的复杂度并提高模型的通用性。
需要注意的是,权重修剪可能会影响模型的准确性,因为一些小的权重值可能在某些情况下仍然具有重要的作用。因此,需要在权衡模型准确性和模型大小之间做出权衡,并根据具体应用场景来选择合适的阈值。
Given a dataset with only two training samples and . Suppose we want to build a Linear Regression model with learning rate . At first, and . 1)Please calculate the cost . 2)Update the parameters and for one time. 3)Please calculate the new cost after updating.
Given:
- Two training samples: `(2, 3)` and `(4, 5)`
- Initial parameters: `theta0 = 0, theta1 = 0`
- Learning rate: `alpha = 0.01`
To build a Linear Regression model, we need to minimize the cost function. The cost function for Linear Regression is:
```
J(theta0, theta1) = (1/2m) * sum(i=1 to m)[hypothesis(xi) - yi]^2
```
where `m` is the total number of training samples, `xi` is the feature of the `i`th training sample, `yi` is the label of the `i`th training sample, and `hypothesis(xi)` is the predicted value for `xi` using the current values of `theta0` and `theta1`.
1) Calculate the initial cost:
```
hypothesis(2) = theta0 + theta1 * 2 = 0 + 0 * 2 = 0
hypothesis(4) = theta0 + theta1 * 4 = 0 + 0 * 4 = 0
J(theta0, theta1) = (1/4) * [(0 - 3)^2 + (0 - 5)^2] = 13/4 = 3.25
```
So the initial cost is `3.25`.
2) Update the parameters for one time:
```
theta0 = theta0 - alpha * (1/m) * sum(i=1 to m)[hypothesis(xi) - yi] = 0 - 0.01 * (1/2) * [(0 - 3) + (0 - 5)] = 0.04
theta1 = theta1 - alpha * (1/m) * sum(i=1 to m)[(hypothesis(xi) - yi) * xi] = 0 - 0.01 * (1/2) * [(0 - 3) * 2 + (0 - 5) * 4] = 0.11
```
So the updated parameters are `theta0 = 0.04` and `theta1 = 0.11`.
3) Calculate the new cost after updating:
```
hypothesis(2) = theta0 + theta1 * 2 = 0.04 + 0.11 * 2 = 0.26
hypothesis(4) = theta0 + theta1 * 4 = 0.04 + 0.11 * 4 = 0.46
J(theta0, theta1) = (1/4) * [(0.26 - 3)^2 + (0.46 - 5)^2] = 2.69/4 = 0.6725
```
So the new cost after updating is `0.6725`.
阅读全文
相关推荐
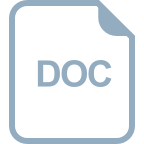
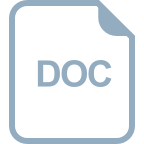
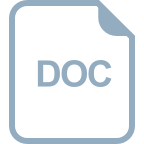
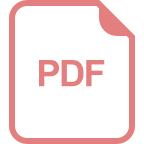
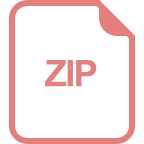











