写一个可以调用excel表格中一列数据进行线性拟合并可视化的python代码
时间: 2023-02-06 08:56:50 浏览: 108
首先,需要使用 Python 中的某个库来读取 Excel 表格中的数据。有许多库可以用来做这件事,比如 pandas、xlrd、openpyxl 等。这里以 pandas 为例,展示如何读取 Excel 表格中的数据:
```python
import pandas as pd
# 读取 Excel 表格中的数据,并将其存储在 DataFrame 中
df = pd.read_excel('file.xlsx', sheet_name='Sheet1')
# 获取 Excel 表格中的一列数据
data = df['column_name']
```
接下来,可以使用 Python 中的 scipy 库来进行线性拟合:
```python
from scipy import stats
# 使用线性回归拟合数据
slope, intercept, r_value, p_value, std_err = stats.linregress(x, y)
# 计算预测值
predictions = intercept + slope * x
```
最后,可以使用 Python 中的 matplotlib 库来进行可视化:
```python
import matplotlib.pyplot as plt
# 绘制原始数据的散点图
plt.scatter(x, y)
# 绘制预测值的拟合直线
plt.plot(x, predictions, 'r')
# 显示图表
plt.show()
```
希望以上内容能够帮助到你!
相关问题
写一个深度图可视化代码
### 回答1:
我可以为您提供一个用于可视化深度图的示例代码:import matplotlib.pyplot as plt
import networkx as nx# 创建一个有向图
G = nx.DiGraph()# 添加节点
G.add_node("nodeA")
G.add_node("nodeB")
G.add_node("nodeC")# 添加边
G.add_edge("nodeA", "nodeB")
G.add_edge("nodeA", "nodeC")# 绘制图形
nx.draw(G, with_labels=True)
plt.show()
### 回答2:
深度图可视化是将深度图像转化为彩色图像显示,以便更直观地观察场景的深度信息。以下是一个基于Python和OpenCV库的深度图可视化代码示例:
```python
import cv2
import numpy as np
def visualize_depth_map(depth_map):
# 获取深度图的最小值和最大值
min_depth = np.min(depth_map)
max_depth = np.max(depth_map)
# 归一化深度图数据到0-255的范围
normalized_depth_map = ((depth_map - min_depth) / (max_depth - min_depth)) * 255
# 将深度图映射到伪彩色图
colormap = cv2.applyColorMap(normalized_depth_map.astype(np.uint8), cv2.COLORMAP_JET)
# 显示彩色深度图
cv2.imshow("Colormap Depth Map", colormap)
cv2.waitKey(0)
cv2.destroyAllWindows()
# 示例深度图数据,0表示最远处,255表示最近处
depth_map = np.random.randint(0, 256, (480, 640)).astype(np.float32)
# 可视化深度图
visualize_depth_map(depth_map)
```
在以上代码中,我们先计算深度图的最小值和最大值,然后将深度值归一化到0-255的范围。接着,利用OpenCV的`cv2.applyColorMap`函数将归一化的深度图像映射到伪彩色图。最后,通过`cv2.imshow`函数显示彩色深度图,并通过`cv2.waitKey`等待用户按下任意按键关闭窗口。
以上是一个简单的深度图可视化代码示例,你可以根据自己的需求进行调整和扩展。
### 回答3:
深度图可视化是指将深度图像转换为彩色图像,以更直观地显示场景中不同深度的物体。下面是一个示例代码,用Python和OpenCV实现深度图可视化:
```python
import cv2
import numpy as np
def visualize_depth_map(depth_map):
# 将深度图进行归一化
normalized_depth = cv2.normalize(depth_map, None, alpha=0, beta=255, norm_type=cv2.NORM_MINMAX, dtype=cv2.CV_8U)
# 将灰度图转换为伪彩色图
colored_depth = cv2.applyColorMap(normalized_depth, cv2.COLORMAP_JET)
return colored_depth
# 读取深度图像
depth_image = cv2.imread("depth_map.png", cv2.IMREAD_GRAYSCALE)
# 调用可视化函数
visualized_depth = visualize_depth_map(depth_image)
# 显示可视化结果
cv2.imshow("Visualized Depth", visualized_depth)
cv2.waitKey(0)
cv2.destroyAllWindows()
```
在这个代码中,首先导入了必要的库:cv2和numpy。然后,定义了一个名为visualize_depth_map的函数,该函数接受输入的深度图像并返回深度图像的彩色可视化结果。
在函数内部,首先对深度图像进行归一化,将像素值范围从0到255进行线性映射。然后,使用cv2.applyColorMap函数将灰度图像转换为伪彩色图像。该函数使用COLORMAP_JET颜色映射将深度值映射到热度颜色范围。
最后,在主函数中,读取深度图像,并调用可视化函数生成彩色的深度图像。最后,使用cv2.imshow函数显示可视化的深度图像,并使用cv2.waitKey和cv2.destroyAllWindows进行窗口管理。
需要注意的是,这只是一个简单的深度图可视化示例,可以根据具体需求进行调整和扩展。
用python读取两列数据来进行线性拟合建立回归模型并可视化
好的,我会尽力使用中文回答您的问题。
首先,你需要有一个包含两列数据的数据集。接着,你可以使用 Python 的 `pandas` 库来读取数据,并将其存储在一个 `DataFrame` 中。例如:
```python
import pandas as pd
df = pd.read_csv('my_data.csv')
```
然后,你可以使用 Python 的 `scikit-learn` 库来建立线性回归模型。首先,你需要将你的数据集分成训练集和测试集。你可以使用 `scikit-learn` 中的 `train_test_split` 函数来完成这一步。例如:
```python
from sklearn.model_selection import train_test_split
X = df[['column1', 'column2']]
y = df['target']
X_train, X_test, y_train, y_test = train_test_split(X, y, test_size=0.2)
```
接着,你可以使用 `scikit-learn` 中的 `LinearRegression` 类来建立线性回归模型。你需要调用 `LinearRegression` 类的 `fit` 方法来训练模型,然后使用 `predict` 方法来对新数据进行预测。例如:
```python
from sklearn.linear_model import LinearRegression
model = LinearRegression()
model.fit(X_train, y_train)
y_pred = model.predict(X_test)
```
最后,你可以使用 Python 的 `matplotlib` 库来可视化你的线性回归模型。你可以使用 `matplotlib` 中的 `scatter` 函数来绘制散点图,并使用 `plot` 函数来绘制回归直线。例如:
```
相关推荐
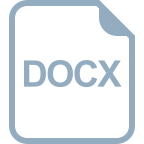
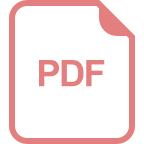
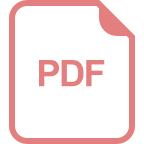













