It looks like you are adding an Embedding layer to your model using Keras. This layer will be used to convert your input text data into a dense vector representation that can be processed by your model. Here's what each of the arguments you've provided to the Embedding layer mean: input_dim: This specifies the size of the vocabulary, or the number of unique words in your input data. It should be set to MAX_WORDS_NUM+1, which ensures that all words in your data are assigned a unique index. output_dim: This specifies the size of the embedding vector for each word. Typically, this is set to a value between 50 and 300, depending on the size of your dataset and complexity of the task. input_length: This specifies the length of each input sequence, or the number of words in each document. It should be set to MAX_SEQUENCE_LEN, which ensures that all input sequences have the same length and can be processed by your model. By adding the Embedding layer to your model, you are essentially creating a lookup table that maps each word in your vocabulary to a dense vector representation. This allows your model to learn meaningful relationships between words and make predictions based on the context of the input data.翻译
时间: 2024-04-05 07:35:10 浏览: 188
看起来你正在使用Keras向你的模型添加一个Embedding层。这个层将被用来将你的输入文本数据转换成一个密集的向量表示,可以被你的模型处理。下面是你提供给Embedding层的每个参数的含义:
- input_dim: 这个参数指定了词汇表的大小,或者说是输入数据中唯一单词的数量。它应该设置为MAX_WORDS_NUM+1,这样确保所有单词都被分配了一个唯一的索引。
- output_dim: 这个参数指定了每个单词的嵌入向量的大小。通常,这个值设置为50到300之间的一个值,取决于数据集的大小和任务的复杂性。
- input_length: 这个参数指定了每个输入序列的长度,或者说是每个文档中单词的数量。它应该设置为MAX_SEQUENCE_LEN,这样确保所有输入序列都具有相同的长度,可以被你的模型处理。
通过向你的模型添加Embedding层,你实际上创建了一个查找表,将词汇表中的每个单词映射到一个密集的向量表示。这样,你的模型可以学习单词之间的有意义的关系,并根据输入数据的上下文进行预测。
相关问题
def train_lstm(n_symbols,embedding_weights,x_train,y_train,x_test,y_test): print ('Defining a Simple Keras Model...') model = Sequential() # or Graph or whatever model.add(Embedding(output_dim=vocab_dim, input_dim=n_symbols, mask_zero=True, weights=[embedding_weights], input_length=input_length)) # Adding Input Length model.add(LSTM(output_dim=50, activation='tanh', inner_activation='hard_sigmoid')) model.add(Dropout(0.5)) model.add(Dense(3, activation='softmax')) # Dense=>全连接层,输出维度=1 model.add(Activation('softmax')) print ('Compiling the Model...') model.compile(loss='categorical_crossentropy', optimizer='adam',metrics=['accuracy']) print ("Train...") # batch_size=32 model.fit(x_train, y_train, batch_size=batch_size, epochs=n_epoch,verbose=1) print ("Evaluate...") score = model.evaluate(x_test, y_test, batch_size=batch_size) yaml_string = model.to_yaml() with open('../model/lstm.yml', 'w') as outfile: outfile.write( yaml.dump(yaml_string, default_flow_style=True) ) model.save_weights('../model/lstm.h5') print ('Test score:', score) print ('Setting up Arrays for Keras Embedding Layer...') n_symbols,embedding_weights,x_train,y_train,x_test,y_test=get_data(index_dict, word_vectors,combined,y) print ("x_train.shape and y_train.shape:") print x_train.shape,y_train.shape train_lstm(n_symbols,embedding_weights,x_train,y_train,x_test,y_test)
这段代码定义了一个名为train_lstm的函数,用于训练一个LSTM模型并保存模型。这个函数包含六个参数,前三个参数n_symbols、embedding_weights和input_length是用于构建Embedding层的参数;后三个参数x_train、y_train和x_test、y_test是用于训练和评估模型的数据集。
具体来说,这个函数的操作包括:
1. 构建一个Sequential模型。
2. 向模型中添加一个Embedding层,使用预训练的词向量作为初始权重,并将输入长度设置为input_length。
3. 向模型中添加一个LSTM层,输出维度为50,激活函数为tanh,内部激活函数为hard_sigmoid。
4. 向模型中添加一个Dropout层,丢弃率为0.5。
5. 向模型中添加一个Dense层,输出维度为3,激活函数为softmax。
6. 编译模型,使用categorical_crossentropy作为损失函数,使用adam作为优化器,评估指标为准确率。
7. 训练模型,使用batch_size=32,训练轮数为n_epoch。
8. 评估模型,计算模型在测试集上的损失和准确率。
9. 将模型的结构保存为YAML文件,将模型的权重保存为HDF5文件。
在函数中,还调用了get_data函数,用于获取训练和测试集。最后,函数输出了模型在测试集上的损失和准确率。
需要注意的是,这段代码中有一些打印语句(print语句),如果你使用的是Python 3,需要将print语句改为print函数的调用形式,即在print后面加上一对括号。同时,这段代码中使用了一些未定义的变量(如vocab_dim、batch_size和n_epoch),你需要在调用train_lstm函数之前先定义这些变量。
def get_data(index_dict,word_vectors,combined,y): n_symbols = len(index_dict) + 1 # 所有单词的索引数,频数小于10的词语索引为0,所以加1 embedding_weights = np.zeros((n_symbols, vocab_dim)) # 初始化 索引为0的词语,词向量全为0 for word, index in index_dict.items(): # 从索引为1的词语开始,对每个词语对应其词向量 embedding_weights[index, :] = word_vectors[word] x_train, x_test, y_train, y_test = train_test_split(combined, y, test_size=0.2) y_train = keras.utils.to_categorical(y_train,num_classes=3) y_test = keras.utils.to_categorical(y_test,num_classes=3) # print x_train.shape,y_train.shape return n_symbols,embedding_weights,x_train,y_train,x_test,y_test ##定义网络结构 def train_lstm(n_symbols,embedding_weights,x_train,y_train,x_test,y_test): print 'Defining a Simple Keras Model...' model = Sequential() # or Graph or whatever model.add(Embedding(output_dim=vocab_dim, input_dim=n_symbols, mask_zero=True, weights=[embedding_weights], input_length=input_length)) # Adding Input Length model.add(LSTM(output_dim=50, activation='tanh')) model.add(Dropout(0.5)) model.add(Dense(3, activation='softmax')) # Dense=>全连接层,输出维度=3 model.add(Activation('softmax')) print 'Compiling the Model...' model.compile(loss='categorical_crossentropy', optimizer='adam',metrics=['accuracy']) print "Train..." # batch_size=32 model.fit(x_train, y_train, batch_size=batch_size, epochs=n_epoch,verbose=1) print "Evaluate..." score = model.evaluate(x_test, y_test, batch_size=batch_size) yaml_string = model.to_yaml() with open('../model/lstm.yml', 'w') as outfile: outfile.write( yaml.dump(yaml_string, default_flow_style=True) ) model.save_weights('../model/lstm.h5') print 'Test score:', score
这段代码是用于训练一个简单的Keras模型,实现情感分析任务的。可以看出,该模型包括了嵌入层、LSTM层、Dropout层和全连接层。其中,嵌入层用于将单词转换为向量表示,LSTM层用于处理序列数据,Dropout层用于防止过拟合,全连接层用于输出分类结果。通过调整模型的参数,训练集和测试集的划分以及优化器等,可以得到不同的模型性能。
阅读全文
相关推荐
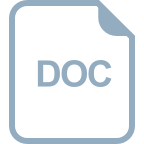
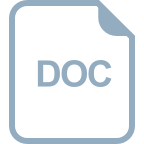
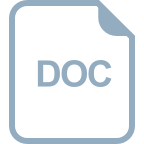
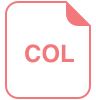

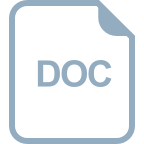
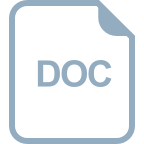
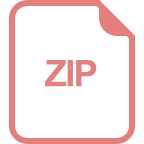
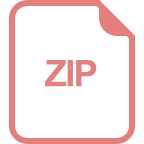
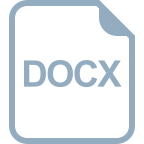
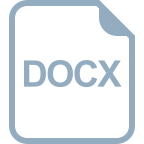
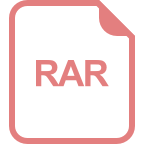