生成对鸢尾花数据集非线性分类,含有最优超参数并且进行可视化的python代码
时间: 2024-05-08 08:19:59 浏览: 13
好的,以下是生成对鸢尾花数据集非线性分类、含有最优超参数并进行可视化的Python代码:
```
# 导入所需库
import numpy as np
import matplotlib.pyplot as plt
from sklearn.datasets import load_iris
from sklearn.model_selection import train_test_split
from sklearn.preprocessing import StandardScaler
from sklearn.svm import SVC
from sklearn.pipeline import Pipeline
from sklearn.model_selection import GridSearchCV
# 加载鸢尾花数据集,并进行划分
iris = load_iris()
X_train, X_test, y_train, y_test = train_test_split(iris.data, iris.target, random_state=0)
# 构建SVM模型
svm = Pipeline([
("scaler", StandardScaler()), # 数据标准化
("svc", SVC(kernel="rbf")) # 核函数采用径向基函数
])
# 设定超参数网格
param_grid = {
"svc__C": [0.1, 1, 10, 100],
"svc__gamma": [0.1, 1, 10, 100]
}
# 使用GridSearchCV寻找最优超参数
grid = GridSearchCV(svm, param_grid=param_grid, cv=5)
grid.fit(X_train, y_train)
# 输出最优超参数并用来构建最终模型
print("Best cross-validation accuracy: {:.2f}".format(grid.best_score_))
print("Best parameters: ", grid.best_params_)
svm = grid.best_estimator_
# 画出决策边界
def plot_decision_boundary(model, ax=None):
if ax is None:
ax = plt.gca()
x_min, x_max = ax.get_xlim()
y_min, y_max = ax.get_ylim()
xx, yy = np.meshgrid(np.linspace(x_min, x_max, 100), np.linspace(y_min, y_max, 100))
Z = model.predict(np.c_[xx.ravel(), yy.ravel()])
Z = Z.reshape(xx.shape)
# 画出等高线
ax.contourf(xx, yy, Z, alpha=0.3)
# 设置x轴和y轴的范围及标签
ax.set_xlim(x_min, x_max)
ax.set_ylim(y_min, y_max)
ax.set_xlabel("Feature 0")
ax.set_ylabel("Feature 1")
# 将数据集降为二维
X_train = X_train[:, :2]
# 构建SVM模型,用最优超参数重新训练
svm = Pipeline([
("scaler", StandardScaler()),
("svc", SVC(kernel="rbf", C=grid.best_params_["svc__C"], gamma=grid.best_params_["svc__gamma"]))
])
svm.fit(X_train, y_train)
# 画出决策边界和训练数据点
plt.scatter(X_train[:, 0], X_train[:, 1], c=y_train, cmap=plt.cm.Set1, edgecolor='k')
plot_decision_boundary(svm)
plt.show()
```
希望以上代码可以对您有所帮助!
相关推荐
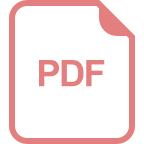
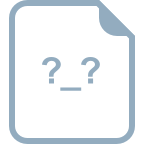
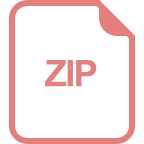














