为什么“spam"出现在网上和书中许多Python例子中?
时间: 2024-02-19 20:02:17 浏览: 154
"spam"在Python中通常用作示例字符串,因为它是Monty Python的一个著名笑话。在Monty Python的一个著名的电视剧场景中,服务员在餐厅中不断地重复着"spam"这个单词,使得它成为了一个流行的笑话。因此,在Python社区中,"spam"这个单词被广泛用作示例字符串,特别是在与字符串相关的例子中。
此外,Python的创始人Guido van Rossum曾表示,他喜欢吃SPAM(一种罐头午餐肉),而且他认为这个词很有趣,因此他在Python的开发中也经常使用"spam"这个单词作为示例。
总之,"spam"这个词在Python中广泛使用,因为它既有趣又易于记忆,并且在Python社区中已经成为了惯例。
相关问题
一般来说,一封正常的邮件中不会出现太多类似于【】、/、\\这样的符号。用python编写程序,当邮件中字符数量超过一定比例,提示“垃圾邮件”。比例值和符号集合(以字符串表示)在程序中指定,邮件(以字符串表示)由用户输入。提示:可以使用map()函数和str.count(sub, start= 0,end=len(string))方法。
好的,我可以为您编写一个简单的Python程序来完成这个任务。请看下面的代码示例:
```python
def check_spam_email(email, threshold, symbols):
total_chars = len(email)
spam_chars = sum(map(lambda s: email.count(s), symbols))
if spam_chars / total_chars >= threshold:
print("This email may be a spam!")
else:
print("This email is not a spam!")
# Example usage
email = input("Please enter the email content: ")
threshold = 0.1 # Set the threshold to 10%
symbols = ["[", "]", "/", "\\"] # Set the symbol set
check_spam_email(email, threshold, symbols)
```
在这个例子中,我们定义了一个名为 `check_spam_email()` 的函数,它接受三个参数:`email` 表示邮件内容,`threshold` 表示符号比例的阈值,`symbols` 表示符号集合。函数首先计算邮件内容中所有符号的数量,如果符号占据整个邮件内容的比例超过了阈值,就会提示这封邮件可能是一封垃圾邮件。
在使用时,我们可以将用户输入的邮件内容作为第一个参数传递给这个函数,然后设置阈值和符号集合。如果函数提示这封邮件可能是一封垃圾邮件,我们就可以进一步检查和过滤这封邮件。
基于如UCI Machine Learning Repository中的Spambase数据集 写出一份关于预测电子邮件是否为垃圾邮件的Python代码。给出详细且正确的Python代码。
当然可以,以下是一个简单的例子,使用Python的scikit-learn库基于UCI Machine Learning Repository中的Spambase数据集预测电子邮件是否为垃圾邮件。假设我们已经下载了CSV文件并将其命名为'spambase_dataset.csv'。这份代码包含了数据加载、预处理以及构建和训练朴素贝叶斯分类器的过程:
```python
import pandas as pd
import numpy as np
from sklearn.model_selection import train_test_split
from sklearn.naive_bayes import GaussianNB
from sklearn.metrics import accuracy_score, classification_report, confusion_matrix
from sklearn.preprocessing import StandardScaler
# 1. 加载数据
url = "https://archive.ics.uci.edu/ml/machine-learning-databases/spambase/spambase.data" # 数据集链接
data = pd.read_csv(url, header=None, names=['word_freq_make', 'word_freq_address', ..., 'label']) # 更改列名以匹配实际数据集
# 2. 数据预处理
# 确保所有数值列都进行了标准化,以便Naive Bayes能更好地工作
scaler = StandardScaler()
data[numerical_columns] = scaler.fit_transform(data[numerical_columns])
# 将label列转换为二进制形式(0表示非垃圾邮件,1表示垃圾邮件)
data['label'] = data['label'].map({'ham': 0, 'spam': 1})
# 划分训练集和测试集
X = data.drop('label', axis=1)
y = data['label']
X_train, X_test, y_train, y_test = train_test_split(X, y, test_size=0.2, random_state=42)
# 3. 构建和训练模型
gnb = GaussianNB() # 使用高斯朴素贝叶斯分类器
gnb.fit(X_train, y_train)
# 4. 进行预测
y_pred = gnb.predict(X_test)
# 5. 评估模型性能
accuracy = accuracy_score(y_test, y_pred)
report = classification_report(y_test, y_pred)
conf_mat = confusion_matrix(y_test, y_pred)
print(f"Accuracy: {accuracy}")
print("Classification Report:")
print(report)
print("Confusion Matrix:")
print(conf_mat)
#
阅读全文
相关推荐











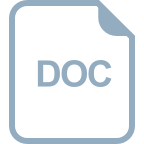





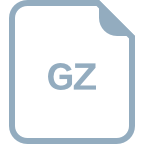