enumerate() 方法使用范例
时间: 2023-12-05 09:04:08 浏览: 25
当然,我可以回答你的问题!
enumerate() 方法用于将一个可遍历的数据对象(如列表、元组或字符串)组合为一个带有索引的序列,常用于 for 循环中。下面是一个使用 enumerate() 的例子:
```
fruits = ['apple', 'banana', 'cherry']
for index, fruit in enumerate(fruits):
print(index, fruit)
```
输出结果为:
```
0 apple
1 banana
2 cherry
```
在这个例子中,我们将 fruits 列表中的元素与对应的索引组合在了一起,并通过 for 循环遍历了这个序列。enumerate() 方法的返回值是一个 enumerate 对象,它包含了每个元素的索引和值。
希望我的回答对你有所帮助!如果你还有其他问题,可以随时问我哦。
相关问题
请给出一个参考代码范例
以下是一个使用PyTorch实现的一维信号GAN生成的参考代码范例:
```python
import torch
import torch.nn as nn
import torch.optim as optim
from torch.utils.data import DataLoader
# 定义生成器网络
class Generator(nn.Module):
def __init__(self, input_size, output_size, hidden_size):
super(Generator, self).__init__()
self.fc1 = nn.Linear(input_size, hidden_size)
self.fc2 = nn.Linear(hidden_size, hidden_size)
self.fc3 = nn.Linear(hidden_size, output_size)
def forward(self, x):
x = nn.functional.relu(self.fc1(x))
x = nn.functional.relu(self.fc2(x))
x = nn.functional.tanh(self.fc3(x))
return x
# 定义判别器网络
class Discriminator(nn.Module):
def __init__(self, input_size, hidden_size):
super(Discriminator, self).__init__()
self.fc1 = nn.Linear(input_size, hidden_size)
self.fc2 = nn.Linear(hidden_size, hidden_size)
self.fc3 = nn.Linear(hidden_size, 1)
def forward(self, x):
x = nn.functional.relu(self.fc1(x))
x = nn.functional.relu(self.fc2(x))
x = nn.functional.sigmoid(self.fc3(x))
return x
# 定义训练过程
def train(generator, discriminator, train_loader, num_epochs, learning_rate):
# 定义损失函数和优化器
criterion = nn.BCELoss()
g_optimizer = optim.Adam(generator.parameters(), lr=learning_rate)
d_optimizer = optim.Adam(discriminator.parameters(), lr=learning_rate)
# 开始训练
for epoch in range(num_epochs):
for i, real_data in enumerate(train_loader):
# 训练判别器
discriminator.zero_grad()
real_data = real_data.view(-1, 1)
real_labels = torch.ones(real_data.size(0), 1)
fake_labels = torch.zeros(real_data.size(0), 1)
real_pred = discriminator(real_data)
d_real_loss = criterion(real_pred, real_labels)
d_real_loss.backward()
noise = torch.randn(real_data.size(0), 100)
fake_data = generator(noise)
fake_pred = discriminator(fake_data.detach())
d_fake_loss = criterion(fake_pred, fake_labels)
d_fake_loss.backward()
d_optimizer.step()
# 训练生成器
generator.zero_grad()
noise = torch.randn(real_data.size(0), 100)
fake_data = generator(noise)
fake_pred = discriminator(fake_data)
g_loss = criterion(fake_pred, real_labels)
g_loss.backward()
g_optimizer.step()
# 输出训练状态
if i % 100 == 0:
print("Epoch [{}/{}], Step [{}/{}], d_real_loss: {:.4f}, d_fake_loss: {:.4f}, g_loss: {:.4f}"
.format(epoch, num_epochs, i, len(train_loader), d_real_loss.item(), d_fake_loss.item(), g_loss.item()))
# 训练数据集
train_data = torch.randn(1000, 1)
# 创建数据加载器
train_loader = DataLoader(train_data, batch_size=50, shuffle=True)
# 创建生成器和判别器
generator = Generator(100, 1, 128)
discriminator = Discriminator(1, 128)
# 训练GAN模型
train(generator, discriminator, train_loader, 20, 0.001)
# 生成新的一维信号
noise = torch.randn(1000, 100)
generated_data = generator(noise)
```
这个代码范例使用一个简单的全连接网络作为生成器和判别器,并使用BCE损失函数和Adam优化器进行训练。在训练完成后,使用生成器网络生成了1000个新的一维信号数据。
写一个俄罗斯方块游戏代码范例
以下是一个简单的俄罗斯方块游戏的代码范例:
```
import pygame
import random
pygame.init()
# 定义游戏窗口大小
WINDOW_WIDTH, WINDOW_HEIGHT = 500, 600
WINDOW = pygame.display.set_mode((WINDOW_WIDTH, WINDOW_HEIGHT))
pygame.display.set_caption("俄罗斯方块")
# 定义方块大小
BLOCK_SIZE = 25
# 定义颜色
WHITE = (255, 255, 255)
BLACK = (0, 0, 0)
RED = (255, 0, 0)
BLUE = (0, 0, 255)
GREEN = (0, 255, 0)
YELLOW = (255, 255, 0)
PURPLE = (255, 0, 255)
ORANGE = (255, 165, 0)
# 定义方块类型和颜色
BLOCKS = {
"I": (BLUE, [(0, 0), (1, 0), (2, 0), (3, 0)]),
"J": (ORANGE, [(0, 0), (0, 1), (1, 1), (2, 1)]),
"L": (YELLOW, [(2, 0), (0, 1), (1, 1), (2, 1)]),
"O": (GREEN, [(0, 0), (1, 0), (0, 1), (1, 1)]),
"S": (PURPLE, [(1, 0), (2, 0), (0, 1), (1, 1)]),
"T": (RED, [(1, 0), (0, 1), (1, 1), (2, 1)]),
"Z": (WHITE, [(0, 0), (1, 0), (1, 1), (2, 1)])
}
# 定义游戏区域
GAME_AREA_WIDTH, GAME_AREA_HEIGHT = 10, 20
GAME_AREA = [[BLACK for _ in range(GAME_AREA_WIDTH)] for _ in range(GAME_AREA_HEIGHT)]
# 定义游戏区域起始位置
GAME_AREA_X, GAME_AREA_Y = 50, 50
# 定义当前方块和下一个方块
current_block = None
next_block = None
# 定义游戏速度和计时器
game_speed = 100
game_timer = 0
# 定义得分
score = 0
# 定义字体
FONT = pygame.font.SysFont("Arial", 30)
def draw_game_area():
"""
绘制游戏区域
"""
for y, row in enumerate(GAME_AREA):
for x, color in enumerate(row):
pygame.draw.rect(WINDOW, color, (GAME_AREA_X + x * BLOCK_SIZE, GAME_AREA_Y + y * BLOCK_SIZE, BLOCK_SIZE, BLOCK_SIZE))
def draw_block(block, x, y):
"""
绘制方块
"""
for bx, by in block[1]:
pygame.draw.rect(WINDOW, block[0], (GAME_AREA_X + (bx + x) * BLOCK_SIZE, GAME_AREA_Y + (by + y) * BLOCK_SIZE, BLOCK_SIZE, BLOCK_SIZE))
def generate_block():
"""
生成方块
"""
global current_block, next_block
if next_block is None:
current_block = random.choice(list(BLOCKS.items()))
else:
current_block = next_block
next_block = random.choice(list(BLOCKS.items()))
return current_block
def is_collision(block, x, y):
"""
检查方块是否与游戏区域或已放置的方块发生碰撞
"""
for bx, by in block[1]:
if x + bx < 0 or x + bx >= GAME_AREA_WIDTH or y + by >= GAME_AREA_HEIGHT:
return True
if y + by >= 0 and GAME_AREA[y + by][x + bx] != BLACK:
return True
return False
def update_game_area():
"""
更新游戏区域状态
"""
global current_block, score
for bx, by in current_block[1]:
GAME_AREA[by + current_y][bx + current_x] = current_block[0]
for y, row in enumerate(GAME_AREA):
if BLACK not in row:
GAME_AREA.pop(y)
GAME_AREA.insert(0, [BLACK for _ in range(GAME_AREA_WIDTH)])
score += 10
def draw_score():
"""
绘制得分
"""
score_text = FONT.render("Score: {}".format(score), True, WHITE)
WINDOW.blit(score_text, (50, 10))
clock = pygame.time.Clock()
while True:
for event in pygame.event.get():
if event.type == pygame.QUIT:
pygame.quit()
quit()
# 更新计时器
game_timer += clock.tick()
# 根据游戏速度更新游戏状态
if game_timer >= game_speed:
game_timer = 0
# 如果当前方块为空,则生成新的方块
if current_block is None:
current_block = generate_block()
current_x, current_y = GAME_AREA_WIDTH // 2 - 2, 0
if is_collision(current_block, current_x, current_y):
pygame.quit()
quit()
# 如果当前方块与游戏区域或已放置的方块发生碰撞,则更新游戏区域状态并清空当前方块
if is_collision(current_block, current_x, current_y + 1):
update_game_area()
current_block = None
# 否则将当前方块下落一格
else:
current_y += 1
# 绘制游戏界面
WINDOW.fill(BLACK)
draw_game_area()
draw_block(current_block, current_x, current_y)
draw_score()
pygame.display.update()
```
相关推荐
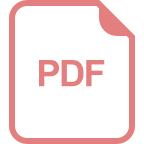
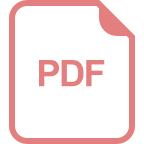







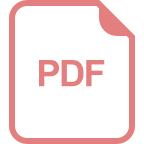
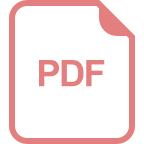
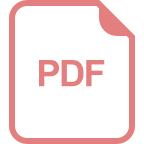