对3个不同角度的usb相机进行拍摄并使用yolo进行检测后的图像进行融合的实体算法代码
时间: 2024-02-27 11:54:35 浏览: 124
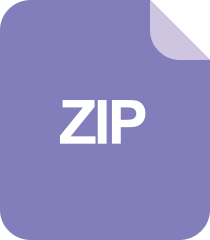
深度学习领域足球比赛视频目标检测(带数据集)-bundesliga-pretrained-yolov7-ball-detect
以下是一个可能的 Python 实现,用于对三个 USB 相机拍摄的图像进行目标检测和融合:
```python
import cv2
import numpy as np
# 1. 加载 YOLO 模型
net = cv2.dnn.readNet("yolov3.weights", "yolov3.cfg")
classes = []
with open("coco.names", "r") as f:
classes = [line.strip() for line in f.readlines()]
colors = np.random.uniform(0, 255, size=(len(classes), 3))
output_layers = [net.getLayerNames()[i[0] - 1] for i in net.getUnconnectedOutLayers()]
# 2. 定义图像检测函数
def detect_objects(img):
height, width, channels = img.shape
blob = cv2.dnn.blobFromImage(img, 0.00392, (416, 416), (0, 0, 0), True, crop=False)
net.setInput(blob)
outs = net.forward(output_layers)
class_ids = []
confidences = []
boxes = []
for out in outs:
for detection in out:
scores = detection[5:]
class_id = np.argmax(scores)
confidence = scores[class_id]
if confidence > 0.5:
center_x = int(detection[0] * width)
center_y = int(detection[1] * height)
w = int(detection[2] * width)
h = int(detection[3] * height)
x = int(center_x - w / 2)
y = int(center_y - h / 2)
boxes.append([x, y, w, h])
confidences.append(float(confidence))
class_ids.append(class_id)
return boxes, confidences, class_ids
# 3. 读取三个相机拍摄的图像
image1 = cv2.imread("image1.jpg")
image2 = cv2.imread("image2.jpg")
image3 = cv2.imread("image3.jpg")
# 4. 对三个图像进行目标检测
boxes1, confidences1, class_ids1 = detect_objects(image1)
boxes2, confidences2, class_ids2 = detect_objects(image2)
boxes3, confidences3, class_ids3 = detect_objects(image3)
# 5. 根据置信度选取最佳的一张图像
best_image = None
best_confidence = 0
if len(confidences1) > 0 and max(confidences1) > best_confidence:
best_image = image1
best_confidence = max(confidences1)
if len(confidences2) > 0 and max(confidences2) > best_confidence:
best_image = image2
best_confidence = max(confidences2)
if len(confidences3) > 0 and max(confidences3) > best_confidence:
best_image = image3
best_confidence = max(confidences3)
# 6. 进行图像融合
merged_image = best_image.copy()
for i in range(len(classes)):
class_id = i
color = colors[class_id]
# 只处理在最佳图像中检测出的目标
for j in range(len(boxes1)):
if class_ids1[j] == class_id and confidences1[j] > 0.5:
x, y, w, h = boxes1[j]
cv2.rectangle(merged_image, (x, y), (x+w, y+h), color, 2)
for j in range(len(boxes2)):
if class_ids2[j] == class_id and confidences2[j] > 0.5:
x, y, w, h = boxes2[j]
cv2.rectangle(merged_image, (x, y), (x+w, y+h), color, 2)
for j in range(len(boxes3)):
if class_ids3[j] == class_id and confidences3[j] > 0.5:
x, y, w, h = boxes3[j]
cv2.rectangle(merged_image, (x, y), (x+w, y+h), color, 2)
# 7. 显示结果图像
cv2.imshow("Result", merged_image)
cv2.waitKey(0)
cv2.destroyAllWindows()
```
需要注意的是,上面的代码只是一个简单的实现,实际场景中可能需要进行更复杂的图像处理和融合操作,具体实现方式可能会因为不同的需求而有所不同。如果您需要更详细的实现方案,可以参考一些相关的论文或者代码库,或者请一位专业的图像处理工程师来帮助您实现。
阅读全文
相关推荐
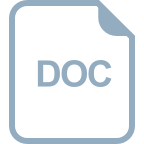
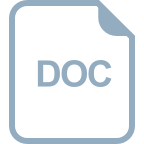
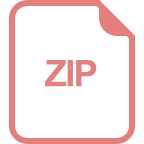
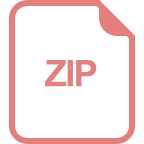
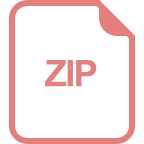
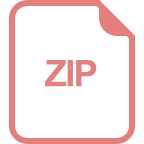
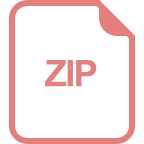
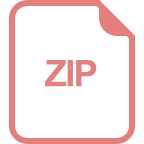
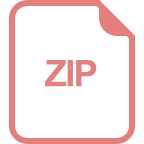
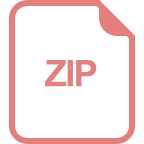
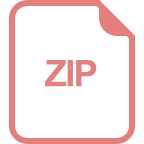
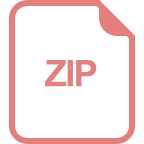
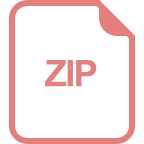
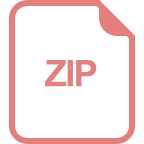
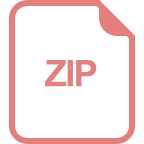
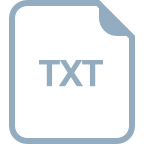
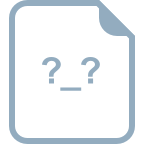