img = skimage.io.imread(image_path_name)请用cv2实现
时间: 2024-02-20 09:00:18 浏览: 26
`skimage.io.imread`函数是scikit-image库中用于读取图像的函数,而`cv2`中也提供了类似的函数`cv2.imread`,你可以使用它来实现相同的功能。下面是使用`cv2.imread`函数来读取图像的代码示例:
```
import cv2
# 读取图像
img = cv2.imread(image_path_name)
# 显示图像
cv2.imshow('image', img)
cv2.waitKey(0)
cv2.destroyAllWindows()
```
其中,`image_path_name`是需要读取的图像的路径和文件名。`cv2.imread`函数返回的是一个numpy数组,表示读取的图像数据。你可以使用`cv2.imshow`函数来显示图像,使用`cv2.waitKey`和`cv2.destroyAllWindows`函数来控制窗口的显示和关闭。
需要注意的是,`cv2.imread`函数默认读取的是BGR格式的图像,而`skimage.io.imread`函数默认读取的是RGB格式的图像。如果你需要使用RGB格式的图像,可以使用以下代码将BGR格式的图像转换为RGB格式:
```
# 将BGR格式的图像转换为RGB格式
img = cv2.cvtColor(img, cv2.COLOR_BGR2RGB)
```
相关问题
class MyDataset(Dataset): def _init__(self, train_path): self.train_path = train_path self.image_path = os.path.join(train_path, 'image') self.label_path = os.path.join(train_path, "label") self.image_path_list = os.listdir(self.image_path) def __getitem__(self, index) -> T_co: image_name = self.image_path_list[index] image_path = os.path.join(self.image_path, image_name) img = cv.imread(image_path) label_name = 'txt'.join(image_name.rsplit(image_name.split('. ')[-1], 1)) label_path = os.path.join(self.label_path, label_name) label = read_label(label_path) return img,label def __len__(self): return Len(self.image_path_list) img, label = my_dataset[data_index]这段代码中img, label = my_dataset[data_index]为什么可以这样用
`img, label = my_dataset[data_index]` 这行代码使用了 Python 的序列解包(sequence unpacking)功能,将 `my_dataset[data_index]` 返回的元组解包成 `img` 和 `label` 两个变量。
在这里,`my_dataset` 是 MyDataset 类的一个实例,`data_index` 是一个整数,它作为索引被传递给 `my_dataset` 的 `__getitem__` 方法。由于 `__getitem__` 方法返回的是一个元组,因此可以使用序列解包将其拆分为两个变量 `img` 和 `label`。
最终,`img` 和 `label` 分别表示了该数据集中第 `data_index` 个图像的图像数据和标签数据。这样就可以使用这些数据进行训练或测试了。
from torch.utils.data import Dataset from torch.utils.data.dataset import T_co import os import cv2 as cv def read_label(path): file = open(path, "r", encoding='utf-8') label = file.readline() file.close() return label class MyDataset(Dataset): def _init__(self, train_path): self.train_path = train_path self.image_path = os.path.join(train_path, 'image') self.label_path = os.path.join(train_path, "label") self.image_path_list = os.listdir(self.image_path) def __getitem__(self, index) -> T_co: image_name = self.image_path_list[index] image_path = os.path.join(self.image_path, image_name) img = cv.imread(image_path) label_name = 'txt'.join(image_name.rsplit(image_name.split('. ')[-1], 1)) label_path = os.path.join(self.label_path, label_name) label = read_label(label_path) return img,label def __len__(self): return Len(self.image_path_list) my_dataset = MyDataset("dataset/train") data_index = 100 img, label = my_dataset[data_index] cv.imshow(label+ str(data_index)+str(Len(my_dataset)) + ')', img) cv.waitKey(0)详解这段代码
这段代码定义了一个自定义的 PyTorch 数据集类 MyDataset,用于加载训练数据集中的图像和标签。其中:
- `_init__` 方法初始化了数据集的路径和图像文件名列表;
- `__getitem__` 方法按照给定的索引 index,读取对应的图像和标签,并返回它们的元组;
- `__len__` 方法返回数据集中图像的数量。
具体来说,这个数据集类假设训练数据集中的图像文件都存储在 "dataset/train/image" 目录下,而对应的标签文件都存储在 "dataset/train/label" 目录下,且标签文件的文件名和对应的图像文件名一致,只是后缀名不同(图像文件的后缀名为图片格式,标签文件的后缀名为 "txt")。标签文件中只包含一个文本行,表示对应图像的标签。
在这个数据集类中,`read_label` 函数用于读取标签文件的内容,`cv.imread` 函数用于读取图像文件的内容,`os.path.join` 函数用于拼接路径,`os.listdir` 函数用于列出目录下的文件名列表。
最后,这段代码创建了一个 MyDataset 类的实例 my_dataset,用于加载 "dataset/train" 目录下的训练数据集。然后它从数据集中取出第 100 个样本,显示它的图像和标签。
相关推荐
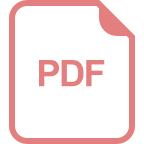
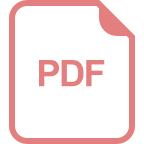
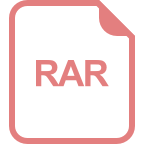










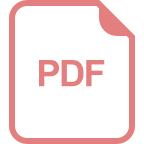
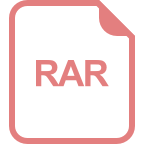
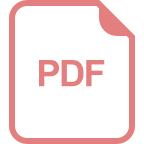