SVM在鸢尾花数据集上的分类
时间: 2024-01-10 10:48:51 浏览: 72
SVM(Support Vector Machine)是一种常用的机器学习算法,适用于分类和回归问题。在鸢尾花数据集上,我们可以使用SVM进行分类任务,将鸢尾花分为三个类别:Setosa、Versicolour和Virginica。
下面是使用Python中的scikit-learn库进行SVM分类的示例代码:
```python
# 导入相关的库和数据集
from sklearn import datasets
from sklearn.model_selection import train_test_split
from sklearn import svm
iris = datasets.load_iris()
X = iris.data
y = iris.target
# 将数据分为训练集和测试集
X_train, X_test, y_train, y_test = train_test_split(X, y, test_size=0.3, random_state=0)
# 使用SVM进行分类
clf = svm.SVC(kernel='linear', C=1)
clf.fit(X_train, y_train)
# 预测测试集数据
y_pred = clf.predict(X_test)
# 输出分类结果
print("Accuracy:", clf.score(X_test, y_test))
```
上述代码中,我们首先加载鸢尾花数据集,然后将数据分为训练集和测试集。接着,我们使用SVM进行分类,并使用训练集对模型进行训练。最后,我们对测试集进行预测,并输出分类准确率。
需要注意的是,这里使用的是线性SVM,在实际应用中可能需要使用其他核函数进行分类。另外,还需要进行参数调节来优化分类效果。
阅读全文
相关推荐
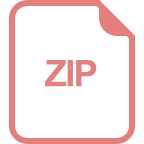
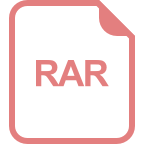
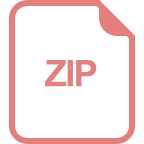
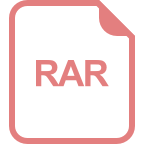








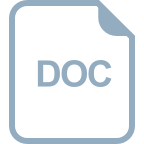
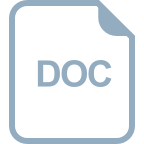
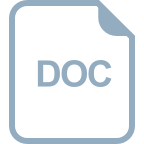

