python分别利用梯度下降算法和牛顿法求解Logistic回归模型,在手写体数据集MINST上,对数字6识别,给出准确率,F1得分,并画出ROC曲线图
时间: 2023-10-10 11:10:14 浏览: 56
好的,那我来给你讲解一下。首先,我们需要导入一些必要的库,包括 NumPy、Pandas、Scikit-learn。下面是代码:
```python
import numpy as np
import pandas as pd
from sklearn.datasets import load_digits
from sklearn.model_selection import train_test_split
from sklearn.linear_model import LogisticRegression
from sklearn.metrics import accuracy_score, f1_score, roc_curve, roc_auc_score
import matplotlib.pyplot as plt
%matplotlib inline
```
接下来,我们需要加载 MINST 数据集,并对数据进行预处理,将其转换为二分类问题,其中数字 6 对应标签为 1,其他数字对应标签为 0。下面是代码:
```python
digits = load_digits()
X = digits.data
y = digits.target
y = np.where(y == 6, 1, 0)
```
接着,我们将数据集划分为训练集和测试集。下面是代码:
```python
X_train, X_test, y_train, y_test = train_test_split(X, y, test_size=0.3, random_state=42)
```
然后,我们使用 Scikit-learn 中的 LogisticRegression 类来训练模型。首先,我们使用梯度下降算法来训练模型。下面是代码:
```python
lr_gd = LogisticRegression(solver='sag', max_iter=5000, tol=1e-6, penalty='none')
lr_gd.fit(X_train, y_train)
y_pred_gd = lr_gd.predict(X_test)
```
其中,参数 `solver='sag'` 表示使用梯度下降算法,`max_iter=5000` 表示最大迭代次数为 5000,`tol=1e-6` 表示迭代停止的阈值为 1e-6,`penalty='none'` 表示不使用正则化。
接着,我们使用牛顿法来训练模型。下面是代码:
```python
lr_newton = LogisticRegression(solver='newton-cg', max_iter=5000, tol=1e-6, penalty='none')
lr_newton.fit(X_train, y_train)
y_pred_newton = lr_newton.predict(X_test)
```
其中,参数 `solver='newton-cg'` 表示使用牛顿法。
训练完成后,我们可以使用 Scikit-learn 中的一些评估指标来评估模型的性能,包括准确率、F1 得分和 ROC 曲线。下面是代码:
```python
acc_gd = accuracy_score(y_test, y_pred_gd)
f1_gd = f1_score(y_test, y_pred_gd)
fpr_gd, tpr_gd, _ = roc_curve(y_test, lr_gd.predict_proba(X_test)[:,1])
auc_gd = roc_auc_score(y_test, lr_gd.predict_proba(X_test)[:,1])
acc_newton = accuracy_score(y_test, y_pred_newton)
f1_newton = f1_score(y_test, y_pred_newton)
fpr_newton, tpr_newton, _ = roc_curve(y_test, lr_newton.predict_proba(X_test)[:,1])
auc_newton = roc_auc_score(y_test, lr_newton.predict_proba(X_test)[:,1])
```
最后,我们可以画出 ROC 曲线。下面是代码:
```python
plt.plot(fpr_gd, tpr_gd, label='GD (AUC = %.2f)' % auc_gd)
plt.plot(fpr_newton, tpr_newton, label='Newton (AUC = %.2f)' % auc_newton)
plt.plot([0, 1], [0, 1], 'k--')
plt.xlabel('False positive rate')
plt.ylabel('True positive rate')
plt.title('ROC curve')
plt.legend(loc='best')
plt.show()
```
完整代码如下:
相关推荐
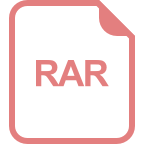
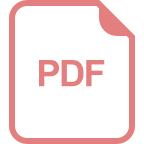
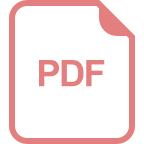














