Visual Studio Code Metrics 介绍一下
时间: 2024-04-06 18:14:11 浏览: 258
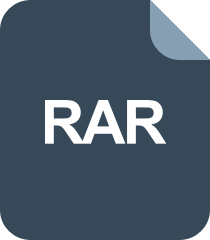
Metrics.rar_数学计算_Visual_C++_
Visual Studio Code Metrics 是 Visual Studio 的一个扩展,用于分析代码质量并生成代码度量报告。它提供了一系列的代码分析指标,包括代码复杂度、类的耦合度、方法长度、代码重复度等等。
使用 Visual Studio Code Metrics,开发者可以更好地了解代码质量,识别潜在的问题,并对代码进行优化。该工具还能够对代码变更进行跟踪,以便在代码发生变化时及时重新分析代码。
Visual Studio Code Metrics 还提供了可定制的报告和图表,方便开发者将分析结果可视化。同时,它还能够与其他工具(如 SonarQube)集成使用,提供更全面的代码质量分析。
阅读全文
相关推荐
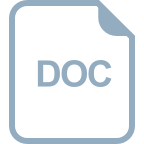
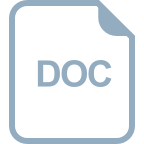
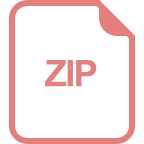

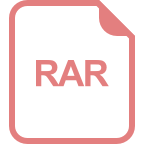
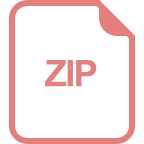
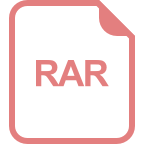
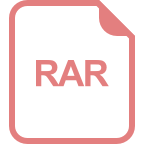
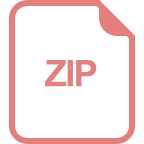
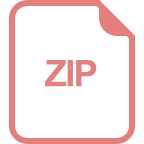
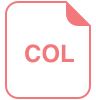
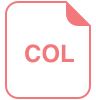
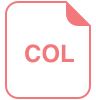
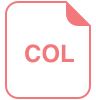



