while ~isempty(indexs(flags)) temp_index = indexs(flags); source = y(:,temp_index(1)); flags(temp_index(1)) = 0; temp_index = temp_index(2:end); temp_flag = []; for i = 1: length(temp_index) corrs = corrcoef(source,y(:,temp_index(i)));%矩阵相关系数 corrs = corrs(1,2); if corrs >= threshold_corr temp_flag(end+1) = i; end end flags(temp_index(temp_flag)) = 0; sgc(:,end+1) = source+sum(y(:,temp_index(temp_flag)),2); g_h = sum(sgc,2); g_h_e = sum((x-g_h).^2); if g_h_e / x_e < threshold_nmse break end end将这块的相似条件改为杰卡德相似系数
时间: 2023-06-16 21:04:12 浏览: 45
要将这段代码的相似条件改为杰卡德相似系数,可以按照以下步骤进行修改:
1. 将计算相关系数的语句改为计算杰卡德相似系数的语句。杰卡德相似系数可以通过计算两个向量的交集与并集的比值来得到,可以使用 MATLAB 自带的 intersect 和 union 函数来实现。修改后的代码如下:
```
for i = 1: length(temp_index)
intersection = intersect(source,y(:,temp_index(i)));
union = union(source,y(:,temp_index(i)));
jaccard = length(intersection) / length(union);
if jaccard >= threshold_jaccard
temp_flag(end+1) = i;
end
end
```
其中,`threshold_jaccard` 是杰卡德相似系数的阈值,可以根据具体需求进行设置。
2. 将计算 NMSE 的语句中的 `g_h` 改为 `sgc`,因为 `sgc` 是当前已经合并的所有信号的和。修改后的代码如下:
```
g_h_e = sum((x-sum(sgc,2)).^2);
```
3. 根据需要,可能还需要对其他部分进行相应的修改。
需要注意的是,杰卡德相似系数与相关系数并不是直接可比的,因为它们的取值范围不同。在使用杰卡德相似系数时,需要根据具体应用场景进行阈值的设置,以确保得到合理的结果。
相关问题
while ~isempty(indexs(flags)) temp_index = indexs(flags); source = y(:,temp_index(1)); flags(temp_index(1)) = 0; temp_index = temp_index(2:end); temp_flag = []; for i = 1: length(temp_index) corrs = corrcoef(source,y(:,temp_index(i))) corrs = corrs(1,2); if corrs >= threshold_corr temp_flag(end+1) = i; 这怎么改成修正余弦相似度
要将这段代码改成修正余弦相似度,需要进行以下修改:
1. 将 `corrcoef` 函数修改为计算修正余弦相似度的函数。修正余弦相似度可以通过以下公式计算:
$$
r_{xy} = \frac{\sum\limits_{i=1}^n (x_i - \bar{x}) (y_i - \bar{y})}{\sqrt{\sum\limits_{i=1}^n (x_i - \bar{x})^2} \sqrt{\sum\limits_{i=1}^n (y_i - \bar{y})^2} + \epsilon}
$$
其中,$x$ 和 $y$ 是要比较的两个向量,$n$ 是向量的长度,$\bar{x}$ 和 $\bar{y}$ 是两个向量的均值,$\epsilon$ 是一个很小的数,防止分母为零。
2. 将 `corrs` 的计算方法改为修正余弦相似度的计算方法,即:
```
corrs = sum((source - mean(source)).*(y(:,temp_index(i)) - mean(y(:,temp_index(i)))))/(sqrt(sum((source - mean(source)).^2))*sqrt(sum((y(:,temp_index(i)) - mean(y(:,temp_index(i)))).^2)) + eps;
```
其中,`source` 是要比较的向量,`y(:,temp_index(i))` 是另一个向量,`eps` 是一个很小的数,防止分母为零。
修改后的代码如下:
```
while ~isempty(indexs(flags))
temp_index = indexs(flags);
source = y(:,temp_index(1));
flags(temp_index(1)) = 0;
temp_index = temp_index(2:end);
temp_flag = [];
for i = 1: length(temp_index)
corrs = sum((source - mean(source)).*(y(:,temp_index(i)) - mean(y(:,temp_index(i)))))/(sqrt(sum((source - mean(source)).^2))*sqrt(sum((y(:,temp_index(i)) - mean(y(:,temp_index(i)))).^2)) + eps;
if corrs >= threshold_corr
temp_flag(end+1) = i;
end
end
flags(temp_index(temp_flag)) = 0;
end
```
indexs = linspace(1,d,d);%生成等差数列。语法为:linspace(x1, x2, n),其中x1是起始值,x2是终止值,n是生成的数字个数 flags = logical(indexs);%逻辑函数 x_e = sum((x-mean(x)).^2);%mean 矩阵均值 方差 sgc = []; g_h = 0; g_h_e = 0; while ~isempty(indexs(flags)) temp_index = indexs(flags); source = y(:,temp_index(1)); flags(temp_index(1)) = 0; temp_index = temp_index(2:end); temp_flag = []; for i = 1: length(temp_index) corrs = corrcoef(source,y(:,temp_index(i)));%矩阵相关系数 corrs = corrs(1,2); if corrs >= threshold_corr temp_flag(end+1) = i; end end flags(temp_index(temp_flag)) = 0; sgc(:,end+1) = source+sum(y(:,temp_index(temp_flag)),2); g_h = sum(sgc,2); g_h_e = sum((x-g_h).^2); if g_h_e / x_e < threshold_nmse break end将这块的相似条件改为余弦相似度
可以将代码中的corrcoef函数替换为pdist2函数,使用余弦相似度计算矩阵之间的相似度。具体代码如下:
indexs = linspace(1,d,d);
flags = logical(indexs);
x_e = sum((x-mean(x)).^2);
sgc = [];
g_h = 0;
g_h_e = 0;
while ~isempty(indexs(flags))
temp_index = indexs(flags);
source = y(:,temp_index(1));
flags(temp_index(1)) = 0;
temp_index = temp_index(2:end);
temp_flag = [];
for i = 1: length(temp_index)
corrs = pdist2(source', y(:,temp_index(i))', 'cosine');
if corrs <= threshold_cosine
temp_flag(end+1) = i;
end
end
flags(temp_index(temp_flag)) = 0;
sgc(:,end+1) = source+sum(y(:,temp_index(temp_flag)),2);
g_h = sum(sgc,2);
g_h_e = sum((x-g_h).^2);
if g_h_e / x_e < threshold_nmse
break
end
end
这样代码中的相似条件就被改为了余弦相似度。
相关推荐
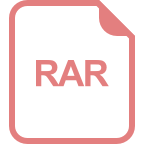
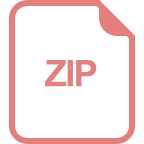
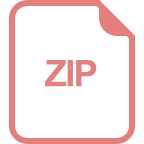










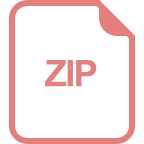
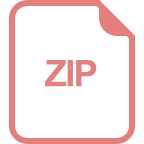
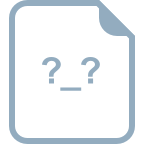