nPop = 100; nGen = 100; pCross = 0.8; pMut = 0.2; nTask = 12; nProj = 3; nRes = 3; resCap = [30, 20, 10]; resCost = [1, 2, 3]; taskRes = [3, 2, 1; 1, 2, 3; 2, 1, 3; 3, 2, 1; 1, 2, 3; 2, 1, 3; 3, 1, 2; 2, 3, 1; 1, 3, 2; 3, 2, 1; 1, 2, 3; 2, 1, 3]; prec = [0 1 0 0 1 0 0 0 0 0 0 0 0 0 1 0 0 0 0 0 0 0 0 0 0 0 0 1 0 1 1 0 0 0 1 0 0 0 0 0 0 0 0 0 0 0 0 0 0 0 0 0 0 0 0 1 0 0 0 0 0 0 0 0 0 0 0 0 1 0 0 0 0 0 0 0 0 0 0 0 0 1 0 0 0 0 0 0 0 0 0 0 0 0 0 1 0 0 0 0 0 0 0 0 0 0 0 0 0 0 0 0 0 0 0 0 0 0 0 0 0 0 0 0 0 0 0 0 0 0 0 0 0 0 0 0 0 0 0 0 0 0 0 0]; pop = initializePopulation(nPop, nTask, nProj); for iGen = 1:nGen [fit, cost] = calculateFitness(pop, nTask, nProj, nRes, resCap, resCost, taskRes, prec); crowd = calculateCrowdingDistance(pop, fit); pop = selection(pop, fit, crowd); pop = crossover(pop, nPop, pCross); pop = mutation(pop, nPop, pMut, nTask, nProj); end [fit, cost] = calculateFitness(pop, nTask, nProj, nRes, resCap, resCost, taskRes, prec); [~, idx] = min(fit); fprintf('最优解:\n'); disp(pop(idx,:));这个代码求的是什么
时间: 2023-06-13 07:03:27 浏览: 34
这段代码实现了一个基于遗传算法的项目调度问题的优化。具体而言,代码中给出了一些参数,如项目中的任务数,项目数,资源数,资源容量,资源成本以及任务与资源之间的关系等。然后通过 initializePopulation 函数初始化种群,然后进行 nGen 轮迭代。在每轮迭代中,通过 calculateFitness 函数计算每个个体的适应度和成本,然后通过 calculateCrowdingDistance 函数计算每个个体的拥挤度,最后通过 selection、crossover 和 mutation 函数进行选择、交叉和变异,得到新的种群。迭代结束后,通过 calculateFitness 函数重新计算每个个体的适应度和成本,并找到适应度最小的个体作为最优解。
相关问题
matlab中Npop = size(population,3)是什么意思
在MATLAB中,`size`函数可以用于获取数组的维度大小。`population`是一个三维数组,`Npop`是一个表示第三维大小的变量。具体地说,`Npop`的值等于`population`数组的第三个维度的大小,也就是该数组中包含的矩阵数量。换句话说,如果`population`是一个`m`行、`n`列、`k`个矩阵的三维数组,则`Npop`的值为`k`。
nPop = 100; nGen = 100; pCross = 0.8; pMut = 0.2; nTask = 12; nProj = 3; nRes = 3; resCap = [30, 20, 10]; resCost = [1, 2, 3]; taskRes = [3, 2, 1; 1, 2, 3; 2, 1, 3; 3, 2, 1; 1, 2, 3; 2, 1, 3; 3, 1, 2; 2, 3, 1; 1, 3, 2; 3, 2, 1; 1, 2, 3; 2, 1, 3]; prec = [0 1 0 0 1 0 0 0 0 0 0 0 0 0 1 0 0 0 0 0 0 0 0 0 0 0 0 1 0 1 1 0 0 0 1 0 0 0 0 0 0 0 0 0 0 0 0 0 0 0 0 0 0 0 0 1 0 0 0 0 0 0 0 0 0 0 0 0 1 0 0 0 0 0 0 0 0 0 0 0 0 1 0 0 0 0 0 0 0 0 0 0 0 0 0 1 0 0 0 0 0 0 0 0 0 0 0 0 0 0 0 0 0 0 0 0 0 0 0 0 0 0 0 0 0 0 0 0 0 0 0 0 0 0 0 0 0 0 0 0 0 0 0 0]; pop = initializePopulation(nPop, nTask, nProj); for iGen = 1:nGen [fit, cost] = calculateFitness(pop, nTask, nProj, nRes, resCap, resCost, taskRes, prec); crowd = calculateCrowdingDistance(pop, fit); pop = selection(pop, fit, crowd); pop = crossover(pop, nPop, pCross); pop = mutation(pop, nPop, pMut, nTask, nProj); end [fit, cost] = calculateFitness(pop, nTask, nProj, nRes, resCap, resCost, taskRes, prec); [~, idx] = min(fit); fprintf('最优解:\n'); disp(pop(idx,:));这个代码中最优解的含义是什么,pop的含义是什么
这个代码是遗传算法用于解决项目调度问题的实现,其中:
- nPop:种群大小;
- nGen:迭代次数(也就是遗传算法的代数);
- pCross:交叉概率;
- pMut:变异概率;
- nTask:任务数;
- nProj:项目数;
- nRes:资源数;
- resCap:每个资源的容量限制;
- resCost:每个资源的使用成本;
- taskRes:每个任务需要消耗的资源数量;
- prec:任务之间的先后关系,如果 prec(i,j) = 1,则表示任务 i 必须在任务 j 之前完成。
pop 是一个 nPop 行 nTask 列的矩阵,代表种群中每个个体(也就是一个项目调度方案)。最优解是指种群中适应度函数值最小的个体,也就是任务完成时间最短的方案。在这段代码中,使用 calculateFitness 函数计算每个个体的适应度函数值,然后选取种群中的精英个体进行交叉和变异操作,得到下一代种群,最后再次计算适应度函数值,找到其中适应度最小的个体就是最优解。
相关推荐
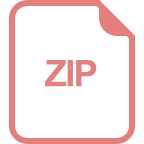
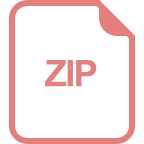
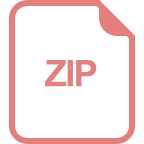













