python随机森林参数调优过程
时间: 2023-07-10 10:36:58 浏览: 87
Python随机森林的参数调优过程如下:
1. 导入必要的库:sklearn.ensemble中的RandomForestClassifier和GridSearchCV。
2. 准备数据集:将原始数据集划分成训练集和测试集。
3. 创建一个随机森林分类器(RandomForestClassifier)对象。
4. 建立参数网格:设定需要调整的参数和参数值,例如n_estimators和max_features等。
5. 创建网格搜索对象(GridSearchCV):传递分类器对象、参数网格和交叉验证的折数等参数。
6. 对训练数据进行网格搜索:fit方法调用。
7. 输出最佳参数:best_params_属性。
8. 用最佳参数训练模型:使用fit方法。
9. 在测试数据上预测:使用predict方法。
10. 输出模型得分:使用score方法。
下面是一个示例代码:
```python
from sklearn.ensemble import RandomForestClassifier
from sklearn.model_selection import GridSearchCV
from sklearn.datasets import make_classification
from sklearn.model_selection import train_test_split
# 准备数据集
X, y = make_classification(n_samples=1000, n_features=10, n_informative=5, n_redundant=0, random_state=0)
X_train, X_test, y_train, y_test = train_test_split(X, y, random_state=0)
# 创建一个随机森林分类器对象
rfc = RandomForestClassifier()
# 建立参数网格
param_grid = {'n_estimators': [10, 50, 100], 'max_features': ['sqrt', 'log2']}
# 创建网格搜索对象
grid_search = GridSearchCV(rfc, param_grid=param_grid, cv=5)
# 对训练数据进行网格搜索
grid_search.fit(X_train, y_train)
# 输出最佳参数
print("Best parameters: {}".format(grid_search.best_params_))
# 用最佳参数训练模型
rfc = RandomForestClassifier(**grid_search.best_params_)
rfc.fit(X_train, y_train)
# 在测试数据上预测
y_pred = rfc.predict(X_test)
# 输出模型得分
print("Model score: {:.2f}".format(rfc.score(X_test, y_test)))
```
在此示例中,我们使用make_classification函数生成一个随机数据集,然后将其划分为训练集和测试集。接下来,我们创建一个随机森林分类器对象,然后建立参数网格。我们使用GridSearchCV函数进行网格搜索,并使用fit方法对训练数据进行拟合。最后,我们输出最佳参数和模型得分。
相关推荐
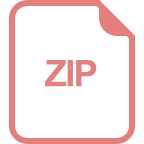
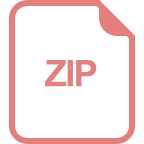
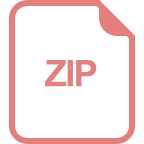














