class _PointnetSAModuleBase(nn.Module): def init(self): super().init() self.npoint = None self.groupers = None self.mlps = None self.pool_method = 'max_pool' def forward(self, xyz: torch.Tensor, features: torch.Tensor = None, new_xyz=None) -> (torch.Tensor, torch.Tensor): """ :param xyz: (B, N, 3) tensor of the xyz coordinates of the features :param features: (B, N, C) tensor of the descriptors of the the features :param new_xyz: :return: new_xyz: (B, npoint, 3) tensor of the new features' xyz new_features: (B, npoint, \sum_k(mlps[k][-1])) tensor of the new_features descriptors """ new_features_list = [] xyz_flipped = xyz.transpose(1, 2).contiguous() if new_xyz is None: new_xyz = pointnet2_utils.gather_operation( xyz_flipped, pointnet2_utils.furthest_point_sample(xyz, self.npoint) ).transpose(1, 2).contiguous() if self.npoint is not None else None for i in range(len(self.groupers)): new_features = self.groupers[i](xyz, new_xyz, features) # (B, C, npoint, nsample) new_features = self.mlpsi # (B, mlp[-1], npoint, nsample) if self.pool_method == 'max_pool': new_features = F.max_pool2d( new_features, kernel_size=[1, new_features.size(3)] ) # (B, mlp[-1], npoint, 1) elif self.pool_method == 'avg_pool': new_features = F.avg_pool2d( new_features, kernel_size=[1, new_features.size(3)] ) # (B, mlp[-1], npoint, 1) else: raise NotImplementedError new_features = new_features.squeeze(-1) # (B, mlp[-1], npoint) new_features_list.append(new_features) return new_xyz, torch.cat(new_features_list, dim=1)你可以给我详细讲解一下这个模块吗,一个语句一个语句的来讲解
时间: 2023-11-26 22:04:05 浏览: 153
这是一个名为_PointnetSAModuleBase的PyTorch模块,是PointNet++中的一个重要组成部分。它是一个基类,用于定义PointNet++中的两种不同的SA(Sampling and Aggregation)模块。接下来,我会一行一行地解释这个模块的代码。
```
class _PointnetSAModuleBase(nn.Module):
```
这里定义了一个名为_PointnetSAModuleBase的PyTorch模块,并继承了nn.Module类。
```
def __init__(self):
super().__init__()
self.npoint = None
self.groupers = None
self.mlps = None
self.pool_method = 'max_pool'
```
这里定义了_PointnetSAModuleBase类的构造函数,并初始化了四个实例变量:self.npoint、self.groupers、self.mlps和self.pool_method。其中,self.npoint是采样点数,self.groupers是对每个采样点进行聚合的模块,self.mlps是一个包含多个MLP(Multi-Layer Perceptron)层的列表,self.pool_method是池化方法,具体可以是最大池化或平均池化。
```
def forward(self, xyz: torch.Tensor, features: torch.Tensor = None, new_xyz=None) -> (torch.Tensor, torch.Tensor):
```
这里定义了_PointnetSAModuleBase类的前向传播函数,输入包括xyz点云坐标张量、features特征张量和new_xyz新的采样点云坐标张量。返回值是一个包含new_xyz和new_features的元组。其中,new_features是根据new_xyz和features计算得到的新特征张量。
```
new_features_list = []
xyz_flipped = xyz.transpose(1, 2).contiguous()
```
这里定义了一个空列表new_features_list和一个翻转了xyz张量维度的张量xyz_flipped。
```
if new_xyz is None:
new_xyz = pointnet2_utils.gather_operation(
xyz_flipped,
pointnet2_utils.furthest_point_sample(xyz, self.npoint)
).transpose(1, 2).contiguous() if self.npoint is not None else None
```
这里判断new_xyz是否为空,如果是,则使用furthest_point_sample函数进行采样,得到一个新的采样点云坐标张量new_xyz。如果self.npoint为空,则将new_xyz设为None。
```
for i in range(len(self.groupers)):
new_features = self.groupers[i](xyz, new_xyz, features) # (B, C, npoint, nsample)
new_features = self.mlpsi # (B, mlp[-1], npoint, nsample)
if self.pool_method == 'max_pool':
new_features = F.max_pool2d(
new_features, kernel_size=[1, new_features.size(3)]
) # (B, mlp[-1], npoint, 1)
elif self.pool_method == 'avg_pool':
new_features = F.avg_pool2d(
new_features, kernel_size=[1, new_features.size(3)]
) # (B, mlp[-1], npoint, 1)
else:
raise NotImplementedError
new_features = new_features.squeeze(-1) # (B, mlp[-1], npoint)
new_features_list.append(new_features)
```
这里遍历self.groupers列表,并对每个采样点进行聚合。对于每个聚合模块,首先将xyz、new_xyz和features传递给它,得到新的new_features张量。然后,将new_features传递给一个包含多个MLP层的列表self.mlps,得到新的new_features张量。接着,根据self.pool_method的值,对new_features张量进行最大池化或平均池化。最后,将new_features张量的最后一个维度压缩掉,并将结果添加到new_features_list列表中。
```
return new_xyz, torch.cat(new_features_list, dim=1)
```
这里返回new_xyz和new_features_list的拼接结果。其中,new_features_list的维度为(B, \sum_k(mlps[k][-1]), npoint),表示每个采样点的特征向量。最后,使用torch.cat函数在第二个维度上进行拼接,得到最终的new_features张量,维度为(B, \sum_k(mlps[k][-1]), npoint)。
阅读全文
相关推荐
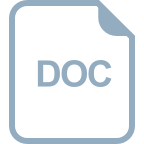
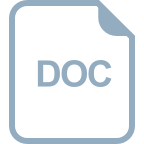
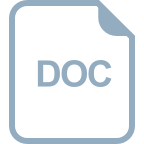












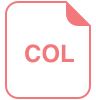
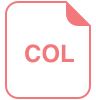

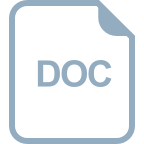