yolov5检测视频流
时间: 2023-07-06 08:42:10 浏览: 202
是的,YOLOv5 可以用于检测视频流。您可以使用 Python 的 OpenCV 库来捕获视频流,然后将每一帧图像输入到 YOLOv5 模型中进行目标检测。以下是一个简单的示例代码:
```python
import cv2
import torch
from models.experimental import attempt_load
from utils.general import non_max_suppression, scale_coords, plot_one_box
from utils.torch_utils import select_device
# Load YOLOv5 model
weights = 'yolov5s.pt'
device = select_device('')
model = attempt_load(weights, map_location=device)
model.to(device).eval()
# Initialize video capture
cap = cv2.VideoCapture(0)
# Define detection threshold
conf_threshold = 0.5
while True:
# Read frame from video capture
ret, frame = cap.read()
# Convert BGR image to RGB
frame = cv2.cvtColor(frame, cv2.COLOR_BGR2RGB)
# Resize image to YOLOv5 input size
img = cv2.resize(frame, (640, 640))
# Normalize image
img = img / 255.0
# Convert image to PyTorch tensor
img = torch.from_numpy(img).float().to(device)
img = img.permute(2, 0, 1).unsqueeze(0)
# Run YOLOv5 inference
detections = model(img)[0]
# Apply NMS
detections = non_max_suppression(detections, conf_threshold)
# Plot bounding boxes and labels on original frame
for detection in detections:
if detection is not None:
detection = scale_coords(img.shape[2:], detection[:, :4], frame.shape).round()
for x1, y1, x2, y2, conf, cls in detection:
label = f'{model.names[int(cls)]} {conf:.2f}'
plot_one_box((x1, y1, x2, y2), frame, label=label)
# Display frame
cv2.imshow('frame', cv2.cvtColor(frame, cv2.COLOR_RGB2BGR))
# Press q to exit
if cv2.waitKey(1) & 0xFF == ord('q'):
break
# Release video capture and destroy windows
cap.release()
cv2.destroyAllWindows()
```
请注意,此代码仅适用于使用 OpenCV 捕获的实时视频流。如果您要检测现有的视频文件,则需要在代码中进行一些修改。
相关推荐
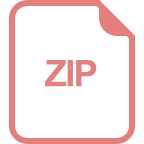














