CLAHE算法自适应阈值
时间: 2023-10-09 18:15:54 浏览: 66
CLAHE算法中的自适应阈值是指在进行直方图均衡化时,用于限制像素值的对比度的一个阈值。在CLAHE算法中,这个阈值是根据图像的局部性质自适应地计算的。具体来说,CLAHE算法将图像分成若干个小块,对每个小块分别计算阈值。为了保证阈值的合理性,CLAHE算法会在计算过程中考虑到块的大小、均值、标准差等因素。一般来说,块越大,阈值就越高,对比度就越低,图像就越平滑;块越小,阈值就越低,对比度就越高,图像就越细节化。因此,在实际应用中,需要根据具体的需求和图像特点来选择适当的块大小和阈值,以获得最佳的图像增强效果。
相关问题
CLAHE自适应调整阈值代码
以下是基于Python编写的CLAHE算法自适应调整阈值的代码示例:
```python
import cv2
def adaptive_clahe(image_path, clip_limit=2.0, tile_size=8):
# 读取图像
image = cv2.imread(image_path, cv2.IMREAD_GRAYSCALE)
# 创建CLAHE对象
clahe = cv2.createCLAHE(clipLimit=clip_limit, tileGridSize=(tile_size, tile_size))
# 自适应调整阈值
adaptive_threshold = cv2.adaptiveThreshold(image, 255, cv2.ADAPTIVE_THRESH_MEAN_C, cv2.THRESH_BINARY, 11, 2)
# 应用CLAHE算法
clahe_image = clahe.apply(image)
# 返回处理后的图像
return clahe_image
```
其中,`image_path`参数表示图像文件的路径,`clip_limit`参数表示限制对比度的阈值,`tile_size`参数表示分块大小。在代码中,首先读取图像,然后创建CLAHE对象,并使用`cv2.adaptiveThreshold`函数自适应调整阈值。最后,将得到的阈值应用于CLAHE算法,生成增强后的图像,并返回其结果。
基于MAD自适应调整CLAHE算法的阈值
基于MAD自适应调整CLAHE算法的阈值,可以使用以下的代码实现:
```python
import cv2
import numpy as np
def adaptive_clahe(image_path, clip_limit=2.0, tile_size=8):
# 读取图像
image = cv2.imread(image_path, cv2.IMREAD_GRAYSCALE)
# 计算图像的中位数和MAD
median = np.median(image)
mad = np.median(np.absolute(image - median))
# 计算CLAHE算法的阈值
threshold = clip_limit * mad / median
# 创建CLAHE对象
clahe = cv2.createCLAHE(clipLimit=threshold, tileGridSize=(tile_size, tile_size))
# 应用CLAHE算法
clahe_image = clahe.apply(image)
# 返回处理后的图像
return clahe_image
```
其中,`image_path`参数表示图像文件的路径,`clip_limit`参数表示固定的对比度限制,`tile_size`参数表示分块大小。在代码中,首先计算图像的中位数和MAD,然后根据公式`threshold = clip_limit * mad / median`计算CLAHE算法的阈值。接着,创建CLAHE对象,并将计算得到的阈值应用于CLAHE算法,生成增强后的图像,并返回其结果。
相关推荐
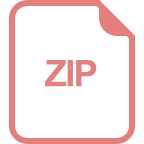
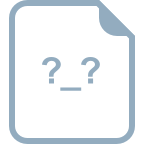












