dataframe中某列中有NA则删除该列
时间: 2024-03-14 19:44:22 浏览: 62
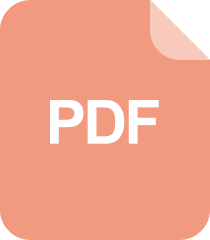
删除DataFrame中值全为NaN或者包含有NaN的列或行方法
在Python中,可以使用pandas库中的DataFrame类型和dropna()方法来删除DataFrame数据中包含NA值的列。假设有一个名为df的DataFrame数据,可以使用以下代码删除包含NA值的列:
```
df = df.dropna(axis=1, how='any')
```
其中,axis参数表示要删除的轴的方向。这里的axis=1表示删除列。how参数表示删除的方式。这里的how='any'表示只要该列中包含一个NA值,就将其删除。如果要求该列中所有值都为NA才删除该列,可以使用how='all'。
上述代码将返回一个新的DataFrame数据,其中已经删除了包含NA值的列。如果要修改原来的DataFrame数据,可以使用inplace=True参数:
```
df.dropna(axis=1, how='any', inplace=True)
```
这里的inplace=True表示直接在原来的DataFrame数据中进行修改。
阅读全文
相关推荐
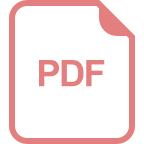

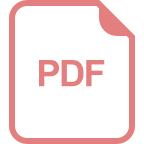
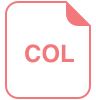
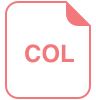
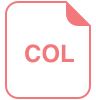
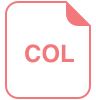
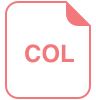
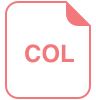
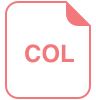
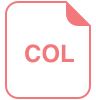
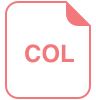
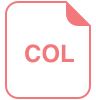
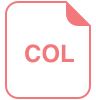



