使用pytorch实现ghost模块替换常规卷积的例子
时间: 2024-04-29 15:24:15 浏览: 10
下面是一个使用pytorch实现ghost模块替换常规卷积的例子:
```python
import torch
import torch.nn as nn
class GhostModule(nn.Module):
def __init__(self, in_channels, out_channels, kernel_size=3, stride=1, padding=1, ratio=2, dw=False):
super().__init__()
self.ratio = ratio
self.out_channels = out_channels
self.kernel_size = kernel_size
self.stride = stride
self.padding = padding
self.dw = dw
self.primary_channels = int(out_channels // ratio)
self.ghost_channels = out_channels - self.primary_channels
if self.dw:
self.primary_conv = nn.Conv2d(in_channels, in_channels, kernel_size, stride, padding, groups=in_channels, bias=False)
else:
self.primary_conv = nn.Conv2d(in_channels, self.primary_channels, kernel_size, stride, padding, bias=False)
self.ghost_conv = nn.Conv2d(in_channels, self.ghost_channels, kernel_size, stride, padding, bias=False)
def forward(self, x):
if self.dw:
primary_out = self.primary_conv(x)
else:
primary_out = self.primary_conv(x[:, :self.primary_channels, :, :])
ghost_out = self.ghost_conv(x)
ghost_out = ghost_out[:, :self.primary_channels, :, :]
out = torch.cat([primary_out, ghost_out], dim=1)
return out
class GhostNet(nn.Module):
def __init__(self, num_classes=1000):
super().__init__()
self.stem = nn.Sequential(
nn.Conv2d(3, 16, 3, stride=2, padding=1, bias=False),
nn.BatchNorm2d(16),
nn.ReLU(inplace=True),
GhostModule(16, 16, kernel_size=3, stride=1, padding=1),
nn.MaxPool2d(2, stride=2),
GhostModule(16, 24, kernel_size=3, stride=1, padding=1),
GhostModule(24, 24, kernel_size=3, stride=1, padding=1),
nn.MaxPool2d(2, stride=2),
GhostModule(24, 40, kernel_size=3, stride=1, padding=1),
GhostModule(40, 40, kernel_size=3, stride=1, padding=1),
nn.MaxPool2d(2, stride=2),
GhostModule(40, 80, kernel_size=3, stride=1, padding=1),
GhostModule(80, 80, kernel_size=3, stride=1, padding=1),
nn.MaxPool2d(2, stride=2),
GhostModule(80, 160, kernel_size=3, stride=1, padding=1),
GhostModule(160, 160, kernel_size=3, stride=1, padding=1),
nn.MaxPool2d(2, stride=2),
GhostModule(160, 320, kernel_size=3, stride=1, padding=1)
)
self.classifier = nn.Sequential(
nn.AdaptiveAvgPool2d((1, 1)),
nn.Flatten(),
nn.Linear(320, num_classes)
)
def forward(self, x):
x = self.stem(x)
x = self.classifier(x)
return x
```
在这个例子中,我们定义了一个GhostModule类,它接受以下参数:
- in_channels:输入特征图的通道数。
- out_channels:输出特征图的通道数。
- kernel_size:卷积核的大小。
- stride:卷积的步幅。
- padding:卷积的填充。
- ratio:primary convolution的输出通道数与ghost convolution的输出通道数之比。
- dw:是否使用深度可分离卷积。
在forward函数中,我们首先使用primary convolution对输入进行卷积,然后使用ghost convolution对输入进行卷积,并将其截取到primary_channels个通道。最后,我们将primary convolution和ghost convolution的输出连接在一起,得到最终的输出。
我们还定义了一个GhostNet类,它使用GhostModule替换了常规卷积,实现了一个基于GhostNet的分类器。在这个例子中,我们使用了一个小型的GhostNet结构,包含了5个GhostModule块和一个全连接层。
相关推荐
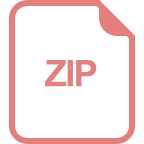
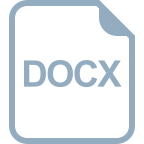














