def Land_cover_pred_plot(array_folder,raster_file, reference_file,ML_algo, plot = False): df_train , train_array = get_data_eval(array_folder,raster_file, reference_file) df_train = df_train.dropna() print(df_train) train_array = np.array(train_array, dtype=object) tile_df = pd.DataFrame() for i, array in enumerate(train_array[0]): # print(train_array[i], train_array_name[i]) tile_df[train_array[1][i]] = np.nan_to_num(array.ravel(), copy=False) # print(train_array[0][i], train_array[1][i]) X_train, X_test, y_train, y_test = train_test_split(df_train.drop('type' , axis = 1),df_train['type'],test_size = 0.1) print(X_train) ML_algo.fit(X_train,y_train) test_pred = ML_algo.predict(X_test) confusion_mat = confusion_matrix(y_test,test_pred) classification_repo = classification_report(y_test, test_pred) test_acc = accuracy_score(y_test, test_pred) print("Confusion Matri : \n", confusion_mat) print("Classification Report : \n", classification_repo) print("Accuracy on Test : ", test_acc) pred_array = ML_algo.predict(tile_df) mask_array = np.reshape(pred_array, train_array[0][0].shape) class_sum = [] for i,j in enumerate(df_train['type'].unique()): sum = (mask_array == j).sum() class_sum.append([j,sum]) print(class_sum) print(mask_array) if plot == True: arr_f = np.array(mask_array, dtype = float) arr_f = np.rot90(arr_f, axes=(-2,-1)) arr_f = np.flip(arr_f,0) plt.imshow(arr_f) plt.colorbar() return mask_array
时间: 2023-06-18 15:02:09 浏览: 134
该函数是一个用于地表覆盖预测和绘图的函数。它需要一个包含训练数据的文件夹路径,一个栅格文件和一个参考文件作为输入。它还需要一个机器学习算法和一个布尔值作为是否要绘制图表的标志。函数调用 get_data_eval 函数来获取训练数据,并使用 train_test_split 函数将其分成训练集和测试集。然后,使用机器学习算法来拟合训练数据,预测测试数据,并计算准确度、混淆矩阵和分类报告。最后,使用训练后的模型来预测栅格文件中的地表覆盖,并将结果绘制成图表(如果 plot 参数为 True)。函数返回预测结果的数组。
阅读全文
相关推荐
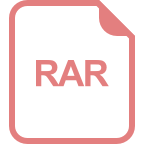
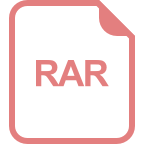
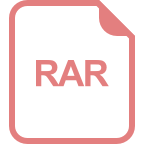
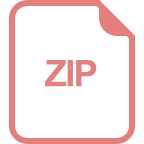
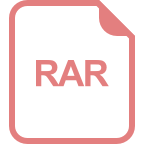