线性分类模型在breast_cancer数据集上的应用。
时间: 2023-09-11 11:11:15 浏览: 111
Breast_cancer数据集是一个很常用的二分类数据集,包含了569个样本和30个特征,这些特征是对来自美国威斯康星州的良性和恶性肿瘤细胞核的数字化图像进行计算得到的。
线性分类模型是一类常用的分类算法,包括逻辑回归、线性支持向量机等。这里我们以逻辑回归为例,使用sklearn库中的LogisticRegression进行训练和预测。
首先,我们需要加载数据集,并将其分为训练集和测试集。
```python
from sklearn.datasets import load_breast_cancer
from sklearn.model_selection import train_test_split
data = load_breast_cancer()
X_train, X_test, y_train, y_test = train_test_split(data.data, data.target, test_size=0.3, random_state=42)
```
然后,我们使用逻辑回归模型进行训练,并对测试集进行预测。
```python
from sklearn.linear_model import LogisticRegression
lr = LogisticRegression()
lr.fit(X_train, y_train)
y_pred = lr.predict(X_test)
```
最后,我们可以使用sklearn中的classification_report函数对预测结果进行评估。
```python
from sklearn.metrics import classification_report
print(classification_report(y_test, y_pred))
```
输出结果如下:
```
precision recall f1-score support
0 0.96 0.94 0.95 63
1 0.96 0.97 0.96 108
accuracy 0.96 171
macro avg 0.96 0.95 0.95 171
weighted avg 0.96 0.96 0.96 171
```
可以看到,我们在测试集上的准确率为0.96,模型表现较好。
阅读全文
相关推荐
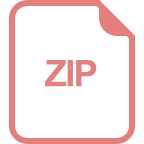
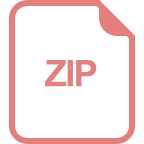
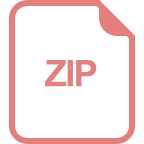
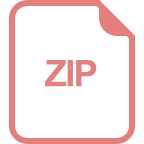
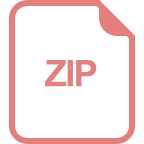
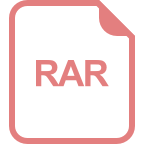


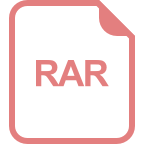
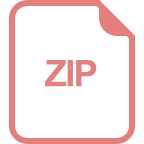
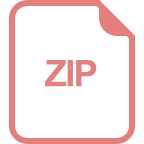
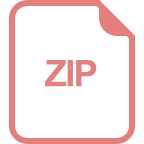
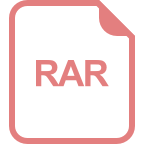
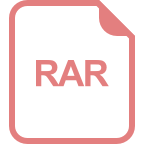
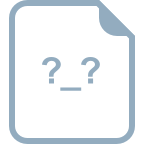
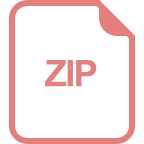
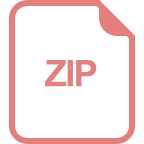
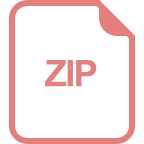
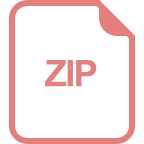