微电网日前优化调度 。算例有代码
时间: 2023-09-22 15:08:03 浏览: 78
很抱歉,我并不清楚你想要什么类型的算例代码,因为微电网的优化调度算法有很多种。以下是一个简单的微电网优化调度算法的 Python 代码示例,其中包括了一个简单的遗传算法优化器:
```python
import random
# 假设微电网有4台发电机,每台发电机分别有3个功率等级可供选择
num_generators = 4
num_power_levels = 3
# 假设微电网需要满足的最小功率需求为100 kW
min_power = 100
# 假设每个功率等级对应的发电机出力分别为[50, 100, 150] kW
power_levels = [50, 100, 150]
# 定义微电网的负载曲线(即负载功率需求随时间变化的曲线)
load_curve = [120, 90, 110, 80, 100, 130, 140, 110, 90, 100]
# 定义遗传算法参数
pop_size = 50 # 种群大小
num_generations = 100 # 迭代次数
mutation_rate = 0.1 # 变异率
# 定义适应度函数
def fitness_function(solution):
# 计算微电网当前时刻的总功率输出
total_power = sum([power_levels[solution[i]][j] for i in range(num_generators) for j in range(len(solution[i])) if solution[i][j] == 1])
# 计算微电网当前时刻的负载
load = load_curve[0]
# 如果微电网当前时刻的总功率输出小于最小功率需求,则适应度为0
if total_power < min_power:
return 0
# 计算微电网当前时刻的适应度,适应度越高表示越优秀
return load / total_power
# 定义随机生成初始解的函数
def generate_random_solution():
return [[random.randint(0, 1) for _ in range(num_power_levels)] for _ in range(num_generators)]
# 定义交叉函数
def crossover(parent1, parent2):
child1 = []
child2 = []
# 以50%的概率对每个基因进行交叉
for i in range(num_generators):
child1_gene = []
child2_gene = []
for j in range(num_power_levels):
if random.random() < 0.5:
child1_gene.append(parent1[i][j])
child2_gene.append(parent2[i][j])
else:
child1_gene.append(parent2[i][j])
child2_gene.append(parent1[i][j])
child1.append(child1_gene)
child2.append(child2_gene)
return (child1, child2)
# 定义变异函数
def mutate(solution):
for i in range(num_generators):
for j in range(num_power_levels):
if random.random() < mutation_rate:
solution[i][j] = 1 - solution[i][j]
return solution
# 初始化种群
population = [generate_random_solution() for _ in range(pop_size)]
# 迭代优化
for generation in range(num_generations):
# 计算每个个体的适应度
fitness_scores = [fitness_function(solution) for solution in population]
# 找出当前种群中适应度最高的个体
best_solution = population[fitness_scores.index(max(fitness_scores))]
print("Generation {}: Best fitness = {}".format(generation, max(fitness_scores)))
# 生成下一代种群
new_population = []
# 选择父母个体进行交叉和变异
for _ in range(pop_size // 2):
parent1 = population[random.randint(0, pop_size - 1)]
parent2 = population[random.randint(0, pop_size - 1)]
child1, child2 = crossover(parent1, parent2)
child1 = mutate(child1)
child2 = mutate(child2)
new_population.append(child1)
new_population.append(child2)
# 将下一代种群替换当前种群
population = new_population
```
上述代码演示了一个简单的微电网优化调度算法,使用遗传算法对微电网的发电机功率进行优化。遗传算法是一种常用的优化算法,其基本思路是通过模拟自然界的进化过程,通过选择、交叉和变异等操作来产生新的解,并不断优化解的质量,最终得到最优解。在上述代码中,适应度函数计算了微电网在当前时刻的总功率输出和负载之间的比例,越高表示越优秀;交叉函数和变异函数分别对父母个体进行基因交叉和基因变异,产生新的解;最终得到的种群中,适应度最高的个体即为当前时刻的最优解。
相关推荐
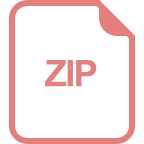
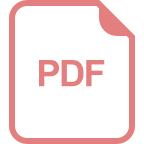
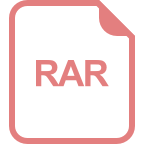


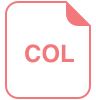
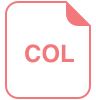










