基于轨迹段聚类的DBSCAN 算法python代码
时间: 2023-06-08 07:09:17 浏览: 418
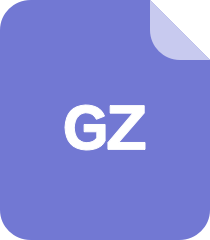
轨迹聚类-trajectory-clustering

以下是基于轨迹段聚类的DBSCAN算法的Python代码:
```
import numpy as np
from sklearn.metrics.pairwise import haversine_distances
def dbscan_trajectory_clustering(X, epsilon, min_samples, metric='haversine'):
"""
Perform DBSCAN clustering on a set of trajectory segments.
Parameters
----------
X : array-like, shape (n_samples, n_features)
The input data representing the trajectory segments. Each row corresponds to a single trajectory
segment and should contain at least two columns representing latitude and longitude.
epsilon : float
The maximum distance between two trajectory segments for them to be considered as belonging to the same
cluster.
min_samples : int
The minimum number of trajectory segments required for a cluster to be considered valid.
metric : string, optional (default='haversine')
The distance metric to use. Should be one of ['haversine', 'euclidean'].
Returns
-------
labels : array-like, shape (n_samples,)
A label array where each element indicates the cluster number of the corresponding trajectory segment.
-1 indicates an outlier.
"""
# Compute pairwise distances between trajectory segments
if metric == 'haversine':
X_rad = np.radians(X[:, :2])
dist_matrix = haversine_distances(X_rad, X_rad) * 6371 * 1000 # Earth radius in meters
elif metric == 'euclidean':
dist_matrix = np.sqrt(np.sum((X[:, :2] - X[:, :2][:, np.newaxis]) ** 2, axis=2))
else:
raise ValueError(f"Unsupported metric: {metric}")
# Perform DBSCAN clustering
labels = np.zeros(X.shape[0], dtype=int)
visited = np.zeros(X.shape[0], dtype=bool)
current_cluster = -1
for i in range(X.shape[0]):
if visited[i]:
continue
visited[i] = True
neighbor_indices = np.where(dist_matrix[i] < epsilon)[0]
if len(neighbor_indices) < min_samples:
labels[i] = -1 # Mark as outlier
else:
current_cluster += 1
labels[i] = current_cluster
j = 0
while j < len(neighbor_indices):
neighbor_index = neighbor_indices[j]
if not visited[neighbor_index]:
visited[neighbor_index] = True
new_neighbor_indices = np.where(dist_matrix[neighbor_index] < epsilon)[0]
if len(new_neighbor_indices) >= min_samples:
neighbor_indices = np.union1d(neighbor_indices, new_neighbor_indices)
if labels[neighbor_index] == 0:
labels[neighbor_index] = current_cluster
j += 1
return labels
```
此代码实现了基于轨迹段聚类的DBSCAN算法,其中输入数据为表示轨迹段的(lat, lon)对,输出一个标签数组表示每个轨迹段所属的簇。该算法可用于抽取轨迹中的行程信息,例如起点、终点、路线等。
阅读全文