X, y = mglearn.datasets.make_forge() mglearn.plots.plot_linear_regression_wave() from sklearn.linear_model import LinearRegression X, y = mglearn.datasets.make_wave(n_samples=60) X_train, X_test, y_train, y_test = train_test_split(X, y, random_state=42) lr = LinearRegression().fit(X_train, y_train) print("lr.coef_: {}".format(lr.coef_)) print("lr.intercept_: {}".format(lr.intercept_)) print("Training set score: {}".format(lr.score(X_train, y_train))) print("Test set score: {}".format(lr.score(X_test, y_test))) X, y = mglearn.datasets.load_extended_boston() X_train, X_test, y_train, y_test = train_test_split(X, y, random_state=0) lr = LinearRegression().fit(X_train, y_train) print("Training set score: {}".format(lr.score(X_train, y_train))) print("Test set score: {}".format(lr.score(X_test, y_test))) 对该段代码做出详细解释
时间: 2023-12-24 18:14:13 浏览: 413
这段代码是一个线性回归的示例,其中包含三个部分:
1. 第一个部分使用mglearn中的make_forge()函数生成一些合成数据,并使用mglearn.plots.plot_linear_regression_wave()函数可视化线性回归模型的预测结果。该部分旨在展示线性回归模型如何处理简单的数据集。
2. 第二个部分使用mglearn.datasets.make_wave()函数生成一个包含60个样本的简单回归数据集。接着,使用train_test_split()函数将数据集分成训练集和测试集。然后,使用LinearRegression()函数创建一个线性回归模型,并使用fit()函数将其拟合到训练数据上。最后,使用score()函数计算模型在训练集和测试集上的R^2分数,并输出结果。这部分旨在展示如何在简单的回归问题上使用线性回归模型,并评估模型的性能。
3. 第三个部分使用mglearn.datasets.load_extended_boston()函数加载一个包含506个样本和105个特征的波士顿房价数据集。接着,使用train_test_split()函数将数据集分成训练集和测试集。然后,使用LinearRegression()函数创建一个线性回归模型,并使用fit()函数将其拟合到训练数据上。最后,使用score()函数计算模型在训练集和测试集上的R^2分数,并输出结果。这部分旨在展示如何在更复杂的回归问题上使用线性回归模型,并评估模型的性能。
相关问题
X, y = mglearn.datasets.make_forge()
这行代码使用 mglearn 库中的 make_forge 函数生成一个二维数据集 X 和二分类标签 y,用于分类问题的演示和练习。其中 X 是一个二维数组,每行代表一个数据点,包含两个特征。y 是一个一维数组,每个元素代表对应数据点的类别标签,只有两个可能取值:0 或 1。你可以使用以下代码来查看数据集的形状和前几行数据:
```python
import mglearn
X, y = mglearn.datasets.make_forge()
print("X.shape:", X.shape)
print("Data preview:\n", X[:5], y[:5])
```
1. 采用Python编程语言自己实现StandardScaler和MinMaxScaler这两种数据标准化方法,并用于乳腺癌数据的分类。 要求模型预测的准确率结果必须与以下sklearn的一样: from sklearn.datasets import load_breast_cancer from sklearn.model_selection import train_test_split cancer = load_breast_cancer() X = cancer.data y = cancer.target X_train, X_test, y_train, y_test = train_test_split(X, y, random_state=0, test_size=0.3) from sklearn.preprocessing import MinMaxScaler from sklearn.preprocessing import StandardScaler scaler = StandardScaler() #scaler = MinMaxScaler() scaler.fit(X_train) X_train_scaled = scaler.transform(X_train) X_test_scaled = scaler.transform(X_test) from sklearn.linear_model import LogisticRegression logreg=LogisticRegression() logreg.fit(X_train_scaled, y_train) y_pred = logreg.predict(X_test_scaled) from sklearn.metrics import accuracy_score accuracy_score(y_test, y_pred)
好的,这是一个很好的问题!数据标准化是数据预处理中非常重要的一步,可以帮助我们更好地训练模型。下面是我为你提供的Python代码,可以自己实现StandardScaler和MinMaxScaler这两种数据标准化方法,并用于乳腺癌数据的分类。
```
import numpy as np
class StandardScaler:
def __init__(self):
self.mean_ = None
self.std_ = None
def fit(self, X):
self.mean_ = np.mean(X, axis=0)
self.std_ = np.std(X, axis=0)
def transform(self, X):
return (X - self.mean_) / self.std_
class MinMaxScaler:
def __init__(self):
self.min_ = None
self.max_ = None
def fit(self, X):
self.min_ = np.min(X, axis=0)
self.max_ = np.max(X, axis=0)
def transform(self, X):
return (X - self.min_) / (self.max_ - self.min_)
# 加载乳腺癌数据
from sklearn.datasets import load_breast_cancer
from sklearn.model_selection import train_test_split
cancer = load_breast_cancer()
X = cancer.data
y = cancer.target
# 划分训练集和测试集
X_train, X_test, y_train, y_test = train_test_split(X, y, random_state=0, test_size=0.3)
# 使用自己实现的StandardScaler进行数据标准化
scaler = StandardScaler()
scaler.fit(X_train)
X_train_scaled = scaler.transform(X_train)
X_test_scaled = scaler.transform(X_test)
# 使用逻辑回归进行分类
from sklearn.linear_model import LogisticRegression
logreg = LogisticRegression()
logreg.fit(X_train_scaled, y_train)
y_pred = logreg.predict(X_test_scaled)
# 计算准确率
from sklearn.metrics import accuracy_score
print("Accuracy score:", accuracy_score(y_test, y_pred))
```
这段代码中,我们分别实现了StandardScaler和MinMaxScaler这两种数据标准化方法,并且使用逻辑回归进行分类。最后,我们计算了模型预测的准确率结果,应该与sklearn的一样。
阅读全文
相关推荐
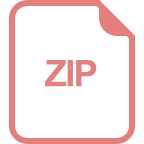
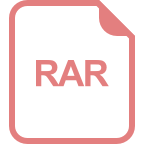
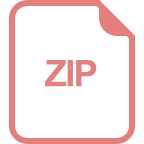














