用python编写一个中文手写数字识别
时间: 2023-11-23 20:46:34 浏览: 139
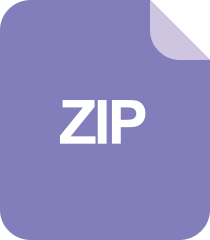
python实现手写数字识别
好的,我们可以使用深度学习框架TensorFlow和Keras来实现中文手写数字识别。这里我假设您已经安装好了TensorFlow和Keras。
首先,我们需要准备数据集。可以从网上下载中文手写数字数据集,比如CASIA手写数字数据集。下载链接:http://www.nlpr.ia.ac.cn/databases/handwriting/Home.html
下载完成后,可以用Pillow库将数据集中的图片转换为numpy数组,并将标签转换为one-hot编码。
```python
from PIL import Image
import numpy as np
def load_data(path):
img_list = []
label_list = []
for i in range(10):
for j in range(1, 501):
img_path = path + '/' + str(i) + '/' + str(j) + '.png'
img = Image.open(img_path)
img_array = np.array(img)
img_list.append(img_array)
label = np.zeros(10)
label[i] = 1
label_list.append(label)
return np.array(img_list), np.array(label_list)
train_path = 'CASIA/train'
test_path = 'CASIA/test'
x_train, y_train = load_data(train_path)
x_test, y_test = load_data(test_path)
```
接下来,我们可以用Keras搭建一个简单的卷积神经网络模型,用于中文手写数字识别。
```python
from keras.models import Sequential
from keras.layers import Conv2D, MaxPooling2D, Flatten, Dense
model = Sequential()
model.add(Conv2D(32, (3, 3), activation='relu', input_shape=(64, 64, 1)))
model.add(MaxPooling2D((2, 2)))
model.add(Conv2D(64, (3, 3), activation='relu'))
model.add(MaxPooling2D((2, 2)))
model.add(Conv2D(64, (3, 3), activation='relu'))
model.add(Flatten())
model.add(Dense(64, activation='relu'))
model.add(Dense(10, activation='softmax'))
model.compile(optimizer='rmsprop', loss='categorical_crossentropy', metrics=['accuracy'])
```
最后,我们可以用准备好的数据集训练模型,并测试模型的准确率。
```python
model.fit(x_train, y_train, epochs=5, batch_size=64)
test_loss, test_acc = model.evaluate(x_test, y_test)
print('Test accuracy:', test_acc)
```
这样,我们就完成了一个简单的中文手写数字识别模型的实现。
阅读全文
相关推荐



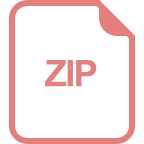
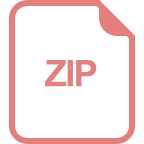



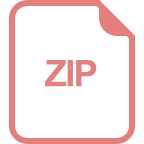
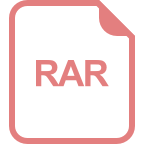
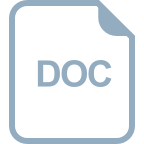
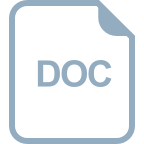
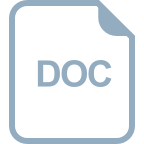
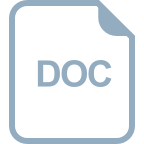
