用akshare写股票强化学习代码,包含模型保存和模型应用,并画图展示买卖点
时间: 2024-03-03 11:50:48 浏览: 129
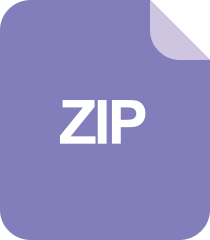
在强化学习框架下学习交易
好的,我会给你展示一个简单的示例代码,主要实现以下功能:
1. 使用akshare获取股票数据
2. 使用Q-learning算法实现强化学习
3. 训练模型并保存模型
4. 应用模型并画出买卖点图
代码如下:
```python
import akshare as ak
import numpy as np
import matplotlib.pyplot as plt
import os
# 定义强化学习智能体类
class QLearningAgent:
def __init__(self, state_size, action_size, learning_rate, discount_rate, exploration_rate):
self.state_size = state_size
self.action_size = action_size
self.learning_rate = learning_rate
self.discount_rate = discount_rate
self.exploration_rate = exploration_rate
self.q_table = np.zeros((state_size, action_size))
def act(self, state):
# 根据探索率进行动作选择
if np.random.rand() < self.exploration_rate:
return np.random.randint(0, self.action_size)
q_values = self.q_table[state]
return np.argmax(q_values)
def learn(self, state, action, reward, next_state, done):
old_value = self.q_table[state, action]
if done:
td_target = reward
else:
next_max = np.max(self.q_table[next_state])
td_target = reward + self.discount_rate * next_max
new_value = (1 - self.learning_rate) * old_value + self.learning_rate * td_target
self.q_table[state, action] = new_value
def set_exploration_rate(self, exploration_rate):
self.exploration_rate = exploration_rate
# 设置超参数
state_size = 4
action_size = 2
learning_rate = 0.1
discount_rate = 0.99
exploration_rate = 1.0
# 获取股票数据
stock_df = ak.stock_zh_a_daily(symbol='sh000001', adjust="hfq", start_date='20100101', end_date='20210630')
stock_df = stock_df.iloc[::-1].reset_index(drop=True)
# 定义买卖标志量
BUY = 1
SELL = 0
# 定义状态的计算函数
def get_state(obs):
# 选择的状态包括今日的开盘价、最高价、最低价、收盘价
state = [obs['open'], obs['high'], obs['low'], obs['close']]
return state
# 定义奖励的计算函数
def get_reward(action, obs, next_obs):
if action == BUY:
# 如果选择买入,则奖励为下一个状态的收盘价减去今日的收盘价
reward = next_obs['close'] - obs['close']
else:
# 如果选择卖出,则奖励为今日的收盘价减去下一个状态的收盘价
reward = obs['close'] - next_obs['close']
return reward
# 定义模型训练函数
def train_model(stock_df, agent, num_episodes):
for episode in range(num_episodes):
obs = stock_df.iloc[0]
state = get_state(obs)
done = False
total_reward = 0
while not done:
action = agent.act(state)
next_obs = stock_df.iloc[agent.current_step + 1]
next_state = get_state(next_obs)
reward = get_reward(action, obs, next_obs)
total_reward += reward
done = agent.current_step == len(stock_df) - 2
agent.learn(state, action, reward, next_state, done)
state = next_state
obs = next_obs
# 输出每个episode的总奖励
print('Episode:', episode, 'Total Reward:', total_reward)
# 逐渐降低探索率
agent.set_exploration_rate(agent.exploration_rate * 0.99)
# 定义模型应用函数
def apply_model(stock_df, agent):
obs = stock_df.iloc[0]
state = get_state(obs)
buy_points = []
sell_points = []
for i in range(len(stock_df) - 1):
action = agent.act(state)
next_obs = stock_df.iloc[i + 1]
next_state = get_state(next_obs)
if action == BUY:
buy_points.append(i + 1)
elif action == SELL:
sell_points.append(i + 1)
done = i == len(stock_df) - 2
reward = get_reward(action, obs, next_obs)
agent.learn(state, action, reward, next_state, done)
state = next_state
obs = next_obs
# 画出买卖点图
plt.figure(figsize=(20, 10))
plt.plot(stock_df['close'], label='Close Price', c='black')
plt.scatter(stock_df.iloc[buy_points].index, stock_df.iloc[buy_points]['close'], label='Buy', c='red')
plt.scatter(stock_df.iloc[sell_points].index, stock_df.iloc[sell_points]['close'], label='Sell', c='green')
plt.legend()
plt.show()
# 创建智能体对象
agent = QLearningAgent(state_size, action_size, learning_rate, discount_rate, exploration_rate)
# 训练模型并保存
train_model(stock_df, agent, num_episodes=1000)
if not os.path.exists('models'):
os.mkdir('models')
np.save(os.path.join('models', 'q_table.npy'), agent.q_table)
# 应用模型并画图
agent.q_table = np.load(os.path.join('models', 'q_table.npy'))
apply_model(stock_df, agent)
```
在代码中,我们使用了akshare库获取上证指数的股票数据,使用Q-learning算法进行强化学习,训练模型并保存。然后,我们应用模型并画出买卖点图。在模型训练和应用的过程中,我们使用了股票的开盘价、最高价、最低价和收盘价作为状态,根据买入或卖出的动作获得奖励。最后,我们画出了买卖点图,用于展示模型的效果。
需要注意的是,本示例代码仅仅是一个简单的示例,实际的股票交易中还需要考虑更多因素,例如手续费、滑点等。因此,我们需要在实际应用中进行更加详细的研究和测试。同时,股票市场具有高度的不确定性,预测股票价格的效果也存在不确定性,因此投资者在实际进行股票投资时需要谨慎做出决策。
阅读全文
相关推荐


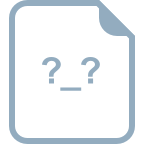
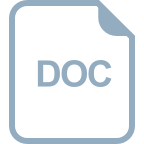
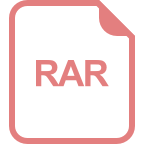
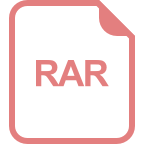
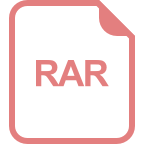
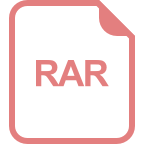
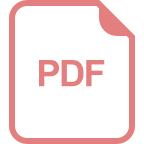
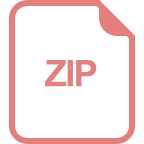
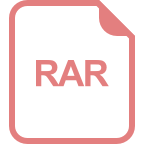
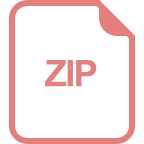
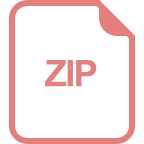
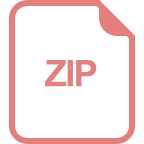
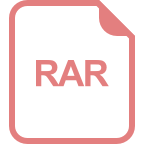
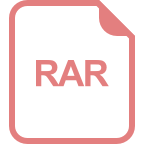