蒙特卡洛算法并行计算代码
时间: 2023-08-28 08:08:12 浏览: 54
蒙特卡洛算法是一种基于随机抽样的计算方法,通常用于求解概率、统计和优化问题。在并行计算方面,蒙特卡洛算法也可以通过将样本分配到不同的处理器上来加速计算。
以下是一个简单的并行蒙特卡洛算法的代码示例:
```
#include <stdio.h>
#include <stdlib.h>
#include <time.h>
#include <omp.h>
#define NUM_SAMPLES 10000000
int main() {
double start_time, end_time;
int count = 0;
double x, y;
int i;
// 初始化随机数种子
srand(time(NULL));
start_time = omp_get_wtime();
// 使用 OpenMP 进行并行计算
#pragma omp parallel for private(x, y) reduction(+:count)
for (i = 0; i < NUM_SAMPLES; i++) {
x = (double)rand() / RAND_MAX;
y = (double)rand() / RAND_MAX;
if (x * x + y * y <= 1) {
count++;
}
}
end_time = omp_get_wtime();
// 计算 π 的近似值
double pi = 4 * (double)count / NUM_SAMPLES;
printf("π ≈ %.6f, time = %.6f seconds\n", pi, end_time - start_time);
return 0;
}
```
这个代码使用 OpenMP 做并行计算,将样本分配到不同的处理器上。`#pragma omp parallel for` 表示将 `for` 循环并行化,`private(x, y)` 表示每个线程分配私有的 `x` 和 `y` 变量,`reduction(+:count)` 表示将 `count` 变量在不同线程间进行累加。
运行该代码,可以得到 π 的近似值和计算时间。注意,随着样本数量的增加,计算结果会更加准确,但计算时间也会增加。可以根据需要调整 `NUM_SAMPLES` 的值来平衡计算精度和计算效率。
相关推荐
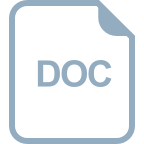
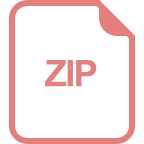
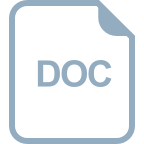














