基于YOLOV5的停车场识别,详细的Python代码以及讲解
时间: 2024-06-09 18:07:06 浏览: 165
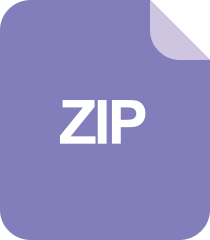
基于YOLOV5的车牌定位和识别源码

首先,YOLOV5是目标检测领域中的一种深度学习算法,主要用于实现目标的快速准确检测。
在停车场识别中,我们可以使用YOLOV5对停车场中的车辆进行检测和识别。下面是基于YOLOV5的停车场识别的Python代码和讲解。
1. 安装YOLOV5
首先,需要安装YOLOV5。可以使用以下命令安装YOLOV5:
```
!git clone https://github.com/ultralytics/yolov5.git
%cd yolov5
!pip install -r requirements.txt
```
2. 下载数据集
接下来,需要下载停车场数据集。可以从以下链接下载:
https://www.kaggle.com/andrewmvd/car-plate-detection
下载完成后,将数据集放在一个文件夹中,例如"data"文件夹。
3. 数据集预处理
在进行训练之前,需要对数据集进行预处理。这包括将数据集划分为训练集和测试集,并将标签转换为YOLO格式。
可以使用以下代码将数据集划分为训练集和测试集:
```
import os
import random
dataset_path = "data"
train_path = "data/train"
test_path = "data/test"
if not os.path.exists(train_path):
os.makedirs(train_path)
if not os.path.exists(test_path):
os.makedirs(test_path)
# 获取所有图像文件的列表
image_files = []
for filename in os.listdir(dataset_path):
if filename.endswith(".jpg"):
image_files.append(os.path.join(dataset_path, filename))
# 随机打乱图像文件列表
random.shuffle(image_files)
# 将前80%的文件用作训练集,后20%的文件用作测试集
split_index = int(0.8 * len(image_files))
train_files = image_files[:split_index]
test_files = image_files[split_index:]
# 将训练集和测试集图像文件复制到相应的目录中
for filename in train_files:
os.system(f"cp {filename} {train_path}")
for filename in test_files:
os.system(f"cp {filename} {test_path}")
```
然后,可以使用以下代码将标签转换为YOLO格式:
```
import os
import csv
import cv2
dataset_path = "data"
train_path = "data/train"
test_path = "data/test"
classes = ["car", "plate"]
# 创建类别名称文件
with open("data/classes.txt", "w") as f:
for c in classes:
f.write(c + "\n")
# 处理训练集和测试集
for split in ["train", "test"]:
path = eval(f"{split}_path")
with open(f"data/{split}.txt", "w") as f:
for filename in os.listdir(path):
if filename.endswith(".jpg"):
# 读取图像文件
img = cv2.imread(os.path.join(path, filename))
height, width, _ = img.shape
# 读取标签文件
label_path = os.path.join(dataset_path, f"{os.path.splitext(filename)[0]}.txt")
with open(label_path, "r") as f2:
labels = csv.reader(f2, delimiter=" ")
for label in labels:
# 转换为YOLO格式
class_index = classes.index(label[0])
x_center = float(label[1]) * width
y_center = float(label[2]) * height
w = float(label[3]) * width
h = float(label[4]) * height
x_min = int(x_center - w / 2)
y_min = int(y_center - h / 2)
x_max = int(x_center + w / 2)
y_max = int(y_center + h / 2)
# 写入文件
f.write(f"{os.path.join(path, filename)} {x_min},{y_min},{x_max},{y_max},{class_index}\n")
```
4. 训练模型
现在,可以使用以下代码训练YOLOV5模型:
```
!python train.py --img 640 --batch 16 --epochs 50 --data data.yaml --cfg models/yolov5s.yaml --weights yolov5s.pt --name parking_lot
```
其中,"--img"指定输入图像的大小,"--batch"指定批次大小,"--epochs"指定训练轮数,"--data"指定数据集配置文件的路径,"--cfg"指定模型配置文件的路径,"--weights"指定预训练模型的路径,"--name"指定训练过程中保存的模型的名称。
5. 测试模型
训练完成后,可以使用以下代码测试模型:
```
!python detect.py --weights runs/train/parking_lot/weights/best.pt --img 640 --conf 0.5 --source data/test
```
其中,"--weights"指定训练完成后保存的最佳模型的路径,"--img"指定输入图像的大小,"--conf"指定置信度阈值,"--source"指定测试集的路径。
6. 结果展示
测试完成后,可以在终端中看到检测结果,也可以在"data/test"文件夹中查看检测结果图像。
以上就是基于YOLOV5的停车场识别的Python代码和讲解。
阅读全文
相关推荐
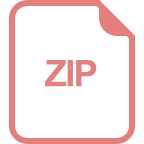
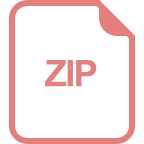
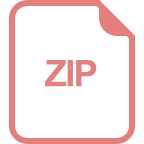
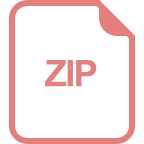
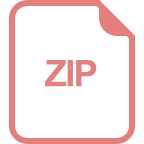
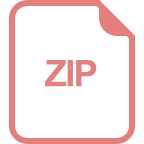
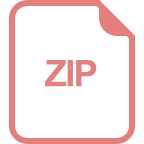
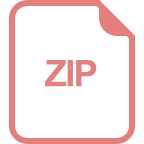
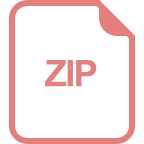
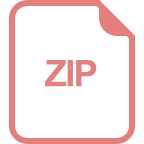
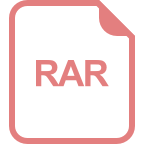
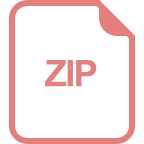
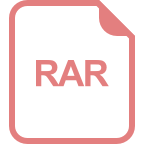
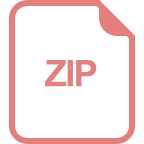
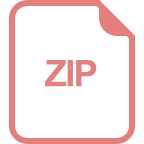
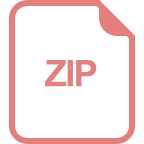
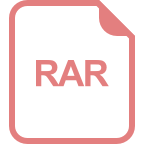