File "E:\PyCharmFile\vgg\vgg19_classification.py", line 173, in <module> model = transfer.ResNet50_model(nb_classes=2, img_rows=image_size, img_cols=image_size, is_plot_model=True)
时间: 2024-03-15 17:41:39 浏览: 69
你的代码中调用了一个名为 `ResNet50_model` 的函数,该函数位于 `transfer` 模块中,并且需要传入四个参数:`nb_classes`、`img_rows`、`img_cols` 和 `is_plot_model`。请检查该函数的定义是否正确,并确保你已经正确地导入了 `transfer` 模块。此外,你还需要检查传入的参数是否正确。如果问题仍然存在,请提供更多的代码信息和错误提示。
阅读全文
相关推荐
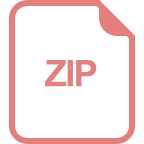
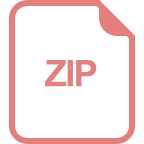
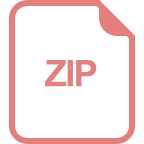
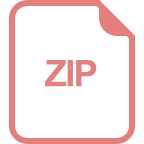
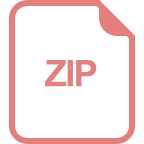
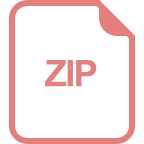
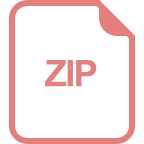
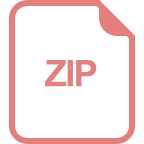
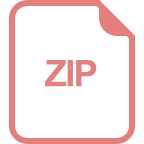
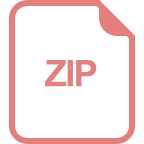
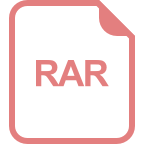
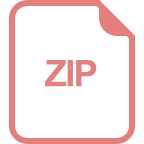
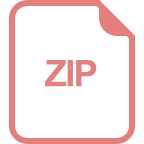
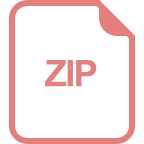
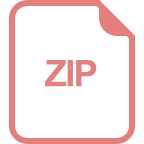
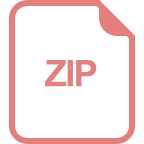
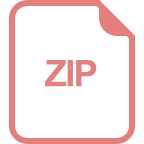
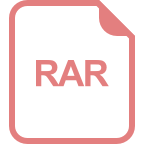