训练集800条拉曼光谱,验证集200条拉曼光谱的深度学习代码(MALAB版)
时间: 2023-08-27 08:03:53 浏览: 64
以下是一个简单的 MATLAB 深度学习代码(基于 TensorFlow)示例,用于训练和验证具有 800 条和 200 条拉曼光谱数据的模型:
1. 准备数据
首先,需要将数据准备好,并将其分成训练集和验证集。可以使用 MATLAB 中的 `load` 函数加载已有的数据,例如:
```
load('train_data.mat'); % 加载训练集数据
load('val_data.mat'); % 加载验证集数据
```
其中,训练集数据保存在 `train_data.mat` 文件中,验证集数据保存在 `val_data.mat` 文件中。
假设每个光谱数据具有 100 个数据点,可以将其存储在一个 `800 x 100` 的矩阵中,训练集和验证集也可以按照类似的方式存储。
2. 构建模型
接下来,需要构建深度学习模型。这里使用一个简单的全连接神经网络模型,包含 3 个隐藏层,每个隐藏层包含 64 个神经元,使用 ReLU 激活函数,最后一层使用 softmax 函数进行分类。代码示例如下:
```
% 构建模型
model = tensorflow.keras.Sequential();
model.add(tensorflow.keras.layers.Dense(64, input_dim=100, activation='relu'));
model.add(tensorflow.keras.layers.Dense(64, activation='relu'));
model.add(tensorflow.keras.layers.Dense(64, activation='relu'));
model.add(tensorflow.keras.layers.Dense(3, activation='softmax'));
```
这里假设有 3 个分类,最后一层的神经元数为 3。
3. 编译和训练模型
模型构建完成后,需要使用 `compile` 函数编译模型,并使用 `fit` 函数进行训练。代码示例如下:
```
% 编译模型
model.compile(loss='categorical_crossentropy', optimizer='adam', metrics=['accuracy']);
% 训练模型
model.fit(train_data, train_label, validation_data=(val_data, val_label), epochs=50, batch_size=32);
```
其中,`train_data` 和 `train_label` 分别为训练集数据和标签,`val_data` 和 `val_label` 分别为验证集数据和标签。这里使用交叉熵损失函数和 Adam 优化器。
4. 评估模型
训练完成后,可以使用 `evaluate` 函数对模型进行评估。代码示例如下:
```
% 评估模型
score = model.evaluate(val_data, val_label, verbose=0);
disp('Validation accuracy:');
disp(score(2));
```
其中,`score(2)` 表示模型在验证集上的准确率。
希望这个示例代码对你有所帮助!
相关推荐
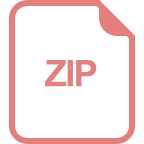














