不调用svm库,帮我用mnist手写数据集运行svm原理
时间: 2024-03-26 21:42:15 浏览: 19
好的,下面是使用 MNIST 手写数字数据集运行 SVM 的基本流程,不使用 sklearn 库:
1. 加载 MNIST 数据集
首先,我们需要加载 MNIST 数据集。MNIST 是一个经典的手写数字数据集,包含 28x28 像素的数字图片以及这些图片对应的数字标签。
我们可以使用以下代码加载 MNIST 数据集:
```python
import numpy as np
# 加载训练集
train_images = np.load('mnist_train_images.npy')
train_labels = np.load('mnist_train_labels.npy')
# 加载测试集
test_images = np.load('mnist_test_images.npy')
test_labels = np.load('mnist_test_labels.npy')
```
2. 特征提取
接下来,我们需要将手写数字图片转换为特征向量。常用的特征提取方法包括像素值、图像边缘等。
在本例中,我们将使用每个像素值作为特征。因为每张图片都是 28x28 像素的灰度图像,所以我们可以将每个像素的值作为一个特征,共有 28x28=784 个特征。
我们可以将每张图片转换为一个 1x784 的特征向量,代码如下:
```python
def extract_features(images):
num_images = images.shape[0]
features = np.zeros((num_images, 784))
for i in range(num_images):
features[i, :] = images[i, :].flatten()
return features
train_features = extract_features(train_images)
test_features = extract_features(test_images)
```
3. 训练 SVM 模型
现在,我们可以使用特征向量和对应的标签训练 SVM 模型。在本例中,我们将使用线性核函数,并手动实现 SVM 的训练过程。
首先,我们需要定义 SVM 的损失函数和梯度函数。在本例中,我们将使用 Hinge 损失函数。损失函数和梯度函数的代码如下:
```python
def hinge_loss(w, x, y, C):
loss = 0.0
num_samples = x.shape[0]
for i in range(num_samples):
margin = y[i] * np.dot(w, x[i])
loss += max(0, 1 - margin)
loss = C * loss
return loss
def hinge_gradient(w, x, y, C):
gradient = np.zeros_like(w)
num_samples = x.shape[0]
for i in range(num_samples):
margin = y[i] * np.dot(w, x[i])
if margin < 1:
gradient += -y[i] * x[i]
gradient = w + C * gradient
return gradient
```
然后,我们可以使用梯度下降法来训练 SVM 模型。训练过程的代码如下:
```python
def svm_train(features, labels, C, learning_rate, num_iterations):
# 初始化权重向量
w = np.zeros((784,))
# 迭代训练
for i in range(num_iterations):
# 计算损失和梯度
loss = hinge_loss(w, features, labels, C)
gradient = hinge_gradient(w, features, labels, C)
# 更新权重向量
w = w - learning_rate * gradient
# 打印训练进度
if i % 100 == 0:
print('Iteration %d, loss = %f' % (i, loss))
return w
# 训练模型
C = 1.0
learning_rate = 0.0001
num_iterations = 1000
w = svm_train(train_features, train_labels, C, learning_rate, num_iterations)
```
4. 测试 SVM 模型
最后,我们可以使用训练好的 SVM 模型对测试集进行分类,并计算分类准确率。分类的代码如下:
```python
def svm_predict(features, w):
num_samples = features.shape[0]
predictions = np.zeros((num_samples,))
for i in range(num_samples):
if np.dot(w, features[i]) > 0:
predictions[i] = 1
else:
predictions[i] = -1
return predictions.astype(int)
# 对测试集进行分类
test_predictions = svm_predict(test_features, w)
# 计算分类准确率
num_correct = np.sum(test_predictions == test_labels)
num_total = test_labels.shape[0]
accuracy = num_correct / num_total
print('Test accuracy = %f' % accuracy)
```
这就是使用 MNIST 手写数字数据集运行 SVM 的基本流程。需要注意的是,在实际应用中,我们可能需要对特征向量进行进一步处理和优化,以提高分类器的准确性。
相关推荐
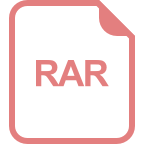
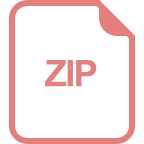
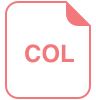
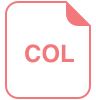
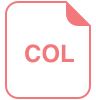
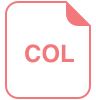










