Python中多元回归和一元线性回归的区别
时间: 2023-11-05 21:09:42 浏览: 213
一元线性回归是指只有一个自变量和一个因变量之间的线性关系,而多元回归则是指有多个自变量和一个因变量之间的线性关系。在Python中,可以使用scikit-learn库来实现一元线性回归和多元回归。
在一元线性回归中,我们可以使用以下Python代码进行实现:
```python
from sklearn.linear_model import LinearRegression
# 定义自变量和因变量
x = [[1], [2], [3], [4], [5]]
y = [2, 4, 5, 4, 5]
# 创建线性回归对象
model = LinearRegression()
# 训练模型
model.fit(x, y)
# 预测结果
y_pred = model.predict([[6]])
print(y_pred)
```
而在多元回归中,我们需要定义多个自变量,例如:
```python
from sklearn.linear_model import LinearRegression
# 定义自变量和因变量
x = [[1, 3], [2, 4], [3, 5], [4, 6], [5, 7]]
y = [2, 4, 5, 4, 5]
# 创建线性回归对象
model = LinearRegression()
# 训练模型
model.fit(x, y)
# 预测结果
y_pred = model.predict([[6, 8]])
print(y_pred)
```
可以看到,多元回归中需要定义多个自变量,而在预测时也需要传入多个自变量的值。
相关问题
如何通过python用多元线性回归预测一元线性回归
多元线性回归和一元线性回归是两种不同的回归分析方法,不能通过多元线性回归来预测一元线性回归。但是,可以使用多元线性回归来预测一个因变量,同时考虑多个自变量的影响,而一元线性回归只考虑一个自变量对因变量的影响。
以下是使用Python进行多元线性回归的步骤:
1. 收集数据,包括因变量和多个自变量。
2. 使用pandas库读取数据并进行清洗和预处理。
3. 将数据分为训练集和测试集。
4. 导入sklearn库并使用线性回归模型进行拟合。
5. 对测试集进行预测,并计算预测精度。
以下是一个示例代码,用于使用多元线性回归预测房价:
```python
import pandas as pd
from sklearn.linear_model import LinearRegression
from sklearn.model_selection import train_test_split
from sklearn.metrics import r2_score
# 读取数据
data = pd.read_csv('house_prices.csv')
# 数据预处理
X = data[['area', 'rooms', 'baths']] #自变量
y = data['price'] #因变量
# 划分数据集
X_train, X_test, y_train, y_test = train_test_split(X, y, test_size=0.2, random_state=0)
# 模型训练
model = LinearRegression()
model.fit(X_train, y_train)
# 预测结果
y_pred = model.predict(X_test)
# 计算预测精度
r2 = r2_score(y_test, y_pred)
print('R2 score:', r2)
```
在上面的代码中,“area”、“rooms”和“baths”是三个自变量,而“price”是因变量。首先,使用pandas库读取数据并进行清洗和预处理。然后,将数据分为训练集和测试集。接着,使用sklearn库中的线性回归模型进行拟合并对测试集进行预测。最后,使用r2_score函数计算预测精度。
线性回归算法一元线性回归、多元线性回归
线性回归是一种用于建立和预测变量之间线性关系的机器学习算法。它可以分为一元线性回归和多元线性回归两种类型。
一元线性回归是指只有一个自变量和一个因变量之间的线性关系。下面是一个使用Python实现的一元线性回归的例子:
```python
import numpy as np
from sklearn.linear_model import LinearRegression
# 定义自变量和因变量
x = np.array([5, 15, 25, 35, 45, 55]).reshape((-1, 1))
y = np.array([5, 20, 14, 32, 22, 38])
# 创建模型并拟合数据
model = LinearRegression()
model.fit(x, y)
# 预测新的数据
x_new = np.array([25]).reshape((-1, 1))
y_new = model.predict(x_new)
print(y_new) # 输出:[16.96]
```
多元线性回归是指有多个自变量和一个因变量之间的线性关系。下面是一个使用Python实现的多元线性回归的例子:
```python
import numpy as np
from sklearn.linear_model import LinearRegression
# 定义自变量和因变量
x = np.array([[5, 1], [15, 2], [25, 5], [35, 11], [45, 15], [55, 18]])
y = np.array([5, 20, 14, 32, 22, 38])
# 创建模型并拟合数据
model = LinearRegression()
model.fit(x, y)
# 预测新的数据
x_new = np.array([[25, 4]])
y_new = model.predict(x_new)
print(y_new) # 输出:[14.24]
```
阅读全文
相关推荐
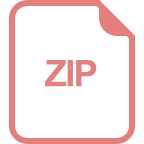
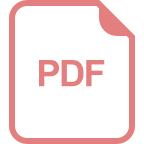
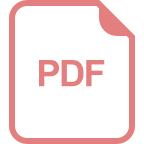
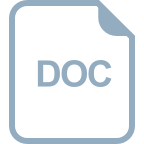
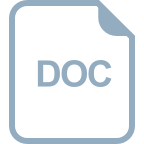
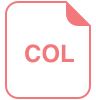




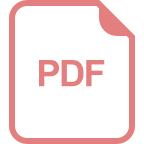
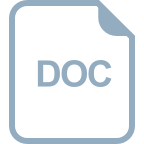