python联立方程组求解
时间: 2023-09-22 14:11:28 浏览: 76
Python中可以使用两阶段最小二乘法(Two-Stage Least Squares,2SLS)来求解联立方程组。以下是使用Python进行联立方程模型求解的步骤:
1. 获取数据:首先导入所需的库,并从数据源中获取数据。可以使用诸如pandas等库来读取和处理数据。
```python
import pandas as pd
import wooldridge as woo
mroz = woo.dataWoo('mroz')
mroz = mroz.dropna(subset=['lwage'])
```
2. 两阶段最小二乘法估计:在Python中,可以使用statsmodels或linearmodels库来进行2SLS回归。以下是使用statsmodels进行2SLS回归的示例代码。
```python
import statsmodels.api as sm
# 第一阶段:拟合第一阶段的回归方程
X = mroz[['educ', 'exper', 'expersq']]
X = sm.add_constant(X)
Y = mroz['lwage']
first_stage_model = sm.OLS(Y, X).fit()
first_stage_results = first_stage_model.predict(X)
# 第二阶段:将第一阶段的预测结果作为内生变量带入联立方程中进行回归
Z = mroz[['educ', 'exper', 'expersq', 'kidslt6', 'kidsge6']]
Z = sm.add_constant(Z)
X2 = mroz[['exper', 'expersq']] # 这里只选择了一部分外生变量
X2 = sm.add_constant(X2)
second_stage_model = sm.OLS(first_stage_results, X2).fit()
second_stage_results = second_stage_model.summary()
```
以上代码中,第一阶段回归中使用了educ、exper和expersq作为自变量,lwage作为因变量。第二阶段回归中使用了第一阶段的预测结果作为内生变量,exper和expersq作为外生变量。
可以根据具体的需求选择使用statsmodels或linearmodels库进行2SLS回归。以上是使用statsmodels库的示例代码。
请注意,这只是一个简单的示例,具体的实现可能会根据数据和模型的复杂程度而有所不同。这些代码只提供了一个基本的框架,你可以根据具体情况进行修改和调整。<span class="em">1</span><span class="em">2</span><span class="em">3</span>
#### 引用[.reference_title]
- *1* *3* [【Python计量】联立方程模型](https://blog.csdn.net/mfsdmlove/article/details/125856209)[target="_blank" data-report-click={"spm":"1018.2226.3001.9630","extra":{"utm_source":"vip_chatgpt_common_search_pc_result","utm_medium":"distribute.pc_search_result.none-task-cask-2~all~insert_cask~default-1-null.142^v93^chatsearchT3_2"}}] [.reference_item style="max-width: 50%"]
- *2* [线性联立方程的雅可比迭代解(jacobi Iteration).(python,数值积分)](https://blog.csdn.net/seventonight/article/details/115733582)[target="_blank" data-report-click={"spm":"1018.2226.3001.9630","extra":{"utm_source":"vip_chatgpt_common_search_pc_result","utm_medium":"distribute.pc_search_result.none-task-cask-2~all~insert_cask~default-1-null.142^v93^chatsearchT3_2"}}] [.reference_item style="max-width: 50%"]
[ .reference_list ]
相关推荐
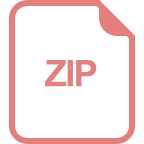
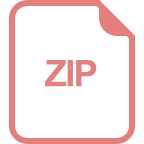














