plt.scatter.contains
时间: 2024-03-27 15:32:57 浏览: 80
plt.scatter.contains是一个函数,用于判断给定的点是否在散点图中的某个点上。它是matplotlib库中的一个方法,用于处理散点图的交互操作。
该函数的语法如下:
plt.scatter.contains(points, verts, transform=None)
其中,points是一个包含(x, y)坐标的数组,表示待判断的点的坐标;verts是一个包含散点图上各个点的路径的数组,表示散点图上各个点的形状。
函数会返回一个布尔值数组,表示每个待判断的点是否在散点图上的某个点上。
相关问题
X=pd.concat([card['Contacts_Count_12_mon'],card['Months_Inactive_12_mon'],card['Total_Amt_Chng_Q4_Q1'],card['Total_Relationship_Count'],card['Total_Trans_Amt'],card['Avg_Utilization_Ratio'],card['Total_Revolving_Bal'],card['Total_Trans_Ct'],card['Gender'],Education_Level_onehot,Income_Category_onehot,Marital_Status_onehot],axis=1) y=card['Attrition_Flag'] from sklearn.tree import DecisionTreeClassifier #X_train, X_test, y_train, y_test = train_test_split(X, y, test_size=0.3, random_state=0) clf = DecisionTreeClassifier() clf.fit(X, y) y_pred = clf.predict(X) #plt.scatter(y, y_pred) #plt.xlabel('True Values') #plt.ylabel('Predictions') #plt.show() plt.scatter(y, y_pred, color='red', label='Predicted Values') plt.scatter(y, clf.predict(y), color='blue', label='True Values') plt.xlabel('X') plt.ylabel('Y') plt.title('Decision Tree Regression') plt.legend() plt.show() plt.show()报错Expected 2D array, got 1D array instead: array=[0. 0. 0. ... 1. 1. 1.]. Reshape your data either using array.reshape(-1, 1) if your data has a single feature or array.reshape(1, -1) if it contains a single sample.
这个报错是因为在使用scatter函数绘制散点图时,y和y_pred都是一维数组,而scatter函数要求输入的数据是二维数组。可以使用reshape函数将y和y_pred转化为二维数组,例如:
```python
y = y.values.reshape(-1, 1)
y_pred = y_pred.reshape(-1, 1)
```
这样可以将y和y_pred都转化为二维数组,使其符合scatter函数的要求。修改后的代码如下:
```python
import pandas as pd
import numpy as np
import matplotlib.pyplot as plt
from sklearn.tree import DecisionTreeClassifier
# 读取数据
card = pd.read_csv('creditcard.csv')
# 特征选择和预处理
Education_Level_onehot = pd.get_dummies(card['Education_Level'], prefix='Education_Level')
Income_Category_onehot = pd.get_dummies(card['Income_Category'], prefix='Income_Category')
Marital_Status_onehot = pd.get_dummies(card['Marital_Status'], prefix='Marital_Status')
# 构造特征矩阵和标签向量
X = pd.concat([card['Contacts_Count_12_mon'],card['Months_Inactive_12_mon'],card['Total_Amt_Chng_Q4_Q1'],card['Total_Relationship_Count'],card['Total_Trans_Amt'],card['Avg_Utilization_Ratio'],card['Total_Revolving_Bal'],card['Total_Trans_Ct'],card['Gender'],Education_Level_onehot,Income_Category_onehot,Marital_Status_onehot],axis=1)
y = card['Attrition_Flag']
# 创建决策树模型
clf = DecisionTreeClassifier()
clf.fit(X, y)
y_pred = clf.predict(X)
# 将y和y_pred转化为二维数组
y = y.values.reshape(-1, 1)
y_pred = y_pred.reshape(-1, 1)
# 绘制散点图
plt.scatter(y, y_pred, color='red', label='Predicted Values')
plt.scatter(y, clf.predict(X), color='blue', label='True Values')
plt.xlabel('True Values')
plt.ylabel('Predictions')
plt.title('Decision Tree Classification')
plt.legend()
plt.show()
```
注意到在这个示例中,我们使用的是分类问题,因此修改了代码中的一些细节。
follow the step give me code and explan it :Forest Cover Type Prediction We shall follow the following steps to complete this challange: Understand the business problem Get the data Discover and visualize insights (univariate and multi variate analysis) Prepare data for ML algorithms Select a model and train it Fine tune your model Launch, monitor and maintain your system (not needed in this case).
Sure, here are the steps along with code explanations:
1. Understand the business problem:
This step involves understanding the problem statement and the objective of the competition. In the case of the Kaggle Forest Cover Type Prediction competition, the objective is to predict the type of forest cover (out of 7 possible types) based on various geographical features like elevation, slope, aspect, etc.
2. Get the data:
The data for this competition can be downloaded from the Kaggle website. It contains both training and testing datasets.
3. Discover and visualize insights:
In this step, we perform exploratory data analysis (EDA) to gain insights into the data. This involves plotting various visualizations like histograms, scatter plots, heat maps, etc. to understand the distribution of the data and the relationships between different features.
4. Prepare data for ML algorithms:
In this step, we preprocess the data to make it suitable for machine learning algorithms. This involves tasks like handling missing values, encoding categorical variables, scaling numerical features, etc.
5. Select a model and train it:
In this step, we select a suitable machine learning model based on the characteristics of the data and the problem statement. We then train the model on the preprocessed data.
6. Fine tune your model:
In this step, we try to improve the performance of the model by fine-tuning its hyperparameters. This involves using techniques like grid search, random search, and Bayesian optimization to find the optimal set of hyperparameters.
7. Launch, monitor and maintain your system:
This step is not relevant for this competition.
Here is some sample Python code for the first few steps:
```python
import pandas as pd
import numpy as np
import matplotlib.pyplot as plt
import seaborn as sns
# Load the data
train_df = pd.read_csv('train.csv')
test_df = pd.read_csv('test.csv')
# Explore the data
print(train_df.head())
# Visualize the target variable
sns.countplot(x='Cover_Type', data=train_df)
plt.show()
# Preprocess the data
from sklearn.preprocessing import StandardScaler
# Drop unnecessary columns
train_df.drop(['Id', 'Soil_Type7', 'Soil_Type15'], axis=1, inplace=True)
test_df.drop(['Id', 'Soil_Type7', 'Soil_Type15'], axis=1, inplace=True)
# Split the data into features and labels
X_train = train_df.drop(['Cover_Type'], axis=1)
y_train = train_df['Cover_Type']
# Scale the features
scaler = StandardScaler()
X_train = scaler.fit_transform(X_train)
```
Note that this code is just a sample and may need to be modified based on the specific requirements of the competition and the characteristics of the data.
阅读全文
相关推荐
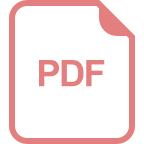
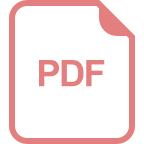
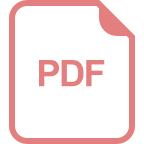
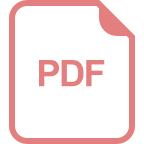
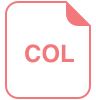
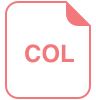
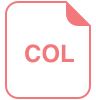
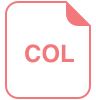
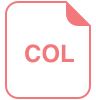
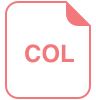
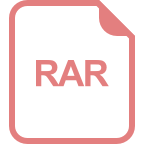
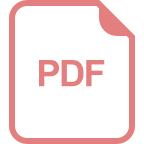
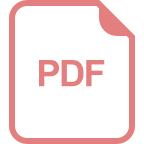
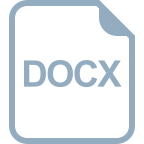
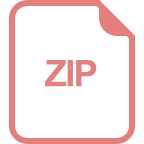
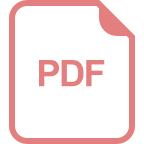