模拟退火算法重建三维多孔介质
时间: 2024-01-10 22:04:14 浏览: 32
模拟退火算法是一种启发式优化算法,可以用于解决路径规划、组合优化等问题。在重建三维多孔介质的问题中,模拟退火算法可以用来优化孔隙分布,使得重建的介质更加接近真实情况。
下面是一个使用模拟退火算法重建三维多孔介质的示例代码:
```python
import numpy as np
# 定义目标函数,评估介质的质量
def evaluate(solution):
# 计算介质的某种性质,例如孔隙率、渗透率等
# 这里假设介质的性质与孔隙分布有关
# 根据具体问题进行定义
return ...
# 定义模拟退火算法
def simulated_annealing():
# 初始化当前解和当前解的评估值
current_solution = ...
current_evaluation = evaluate(current_solution)
# 初始化最优解和最优解的评估值
best_solution = current_solution
best_evaluation = current_evaluation
# 设置初始温度和终止温度
initial_temperature = ...
final_temperature = ...
# 设置迭代次数
max_iterations = ...
# 迭代优化过程
for iteration in range(max_iterations):
# 更新温度
temperature = initial_temperature * (final_temperature / initial_temperature) ** (iteration / max_iterations)
# 生成新解
new_solution = ...
# 计算新解的评估值
new_evaluation = evaluate(new_solution)
# 判断是否接受新解
if new_evaluation < current_evaluation:
current_solution = new_solution
current_evaluation = new_evaluation
else:
# 计算接受新解的概率
acceptance_probability = np.exp((current_evaluation - new_evaluation) / temperature)
# 以一定概率接受新解
if np.random.rand() < acceptance_probability:
current_solution = new_solution
current_evaluation = new_evaluation
# 更新最优解
if current_evaluation < best_evaluation:
best_solution = current_solution
best_evaluation = current_evaluation
return best_solution
# 调用模拟退火算法进行重建
best_solution = simulated_annealing()
# 输出最优解
print(best_solution)
```
请注意,上述代码只是一个示例,具体的重建过程和目标函数需要根据实际问题进行定义和实现。你可以根据自己的需求修改代码,并根据实际情况进行参数调整。
相关推荐
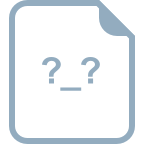














