densenet模型代码
时间: 2024-03-20 13:35:00 浏览: 15
以下是DenseNet模型的代码实现,其中包括DenseLayer、DenseBlock和Transition三个核心细节结构的实现:
```python
import torch
import torch.nn as nn
import torch.nn.functional as F
class DenseLayer(nn.Module):
def __init__(self, in_channels, growth_rate):
super(DenseLayer, self).__init__()
self.bn1 = nn.BatchNorm2d(in_channels)
self.conv1 = nn.Conv2d(in_channels, growth_rate, kernel_size=3, padding=1, bias=False)
def forward(self, x):
out = self.conv1(F.relu(self.bn1(x)))
out = torch.cat([x, out], 1)
return out
class DenseBlock(nn.Module):
def __init__(self, in_channels, growth_rate, num_layers):
super(DenseBlock, self).__init__()
self.layers = nn.ModuleList([DenseLayer(in_channels + i * growth_rate, growth_rate) for i in range(num_layers)])
def forward(self, x):
for layer in self.layers:
x = layer(x)
return x
class Transition(nn.Module):
def __init__(self, in_channels, out_channels):
super(Transition, self).__init__()
self.bn1 = nn.BatchNorm2d(in_channels)
self.conv1 = nn.Conv2d(in_channels, out_channels, kernel_size=1, bias=False)
self.avgpool = nn.AvgPool2d(kernel_size=2, stride=2)
def forward(self, x):
out = self.conv1(F.relu(self.bn1(x)))
out = self.avgpool(out)
return out
class DenseNet(nn.Module):
def __init__(self, growth_rate=32, block_config=(6, 12, 24, 16), num_classes=1000):
super(DenseNet, self).__init__()
self.conv1 = nn.Conv2d(3, 64, kernel_size=7, stride=2, padding=3, bias=False)
self.bn1 = nn.BatchNorm2d(64)
self.relu = nn.ReLU(inplace=True)
self.maxpool = nn.MaxPool2d(kernel_size=3, stride=2, padding=1)
self.block1 = DenseBlock(64, growth_rate, block_config[0])
in_channels = 64 + growth_rate * block_config[0]
self.trans1 = Transition(in_channels, in_channels // 2)
in_channels = in_channels // 2
self.block2 = DenseBlock(in_channels, growth_rate, block_config[1])
in_channels = in_channels + growth_rate * block_config[1]
self.trans2 = Transition(in_channels, in_channels // 2)
in_channels = in_channels // 2
self.block3 = DenseBlock(in_channels, growth_rate, block_config[2])
in_channels = in_channels + growth_rate * block_config[2]
self.trans3 = Transition(in_channels, in_channels // 2)
in_channels = in_channels // 2
self.block4 = DenseBlock(in_channels, growth_rate, block_config[3])
in_channels = in_channels + growth_rate * block_config[3]
self.bn2 = nn.BatchNorm2d(in_channels)
self.avgpool = nn.AdaptiveAvgPool2d((1, 1))
self.fc = nn.Linear(in_channels, num_classes)
def forward(self, x):
x = self.conv1(x)
x = self.bn1(x)
x = self.relu(x)
x = self.maxpool(x)
x = self.block1(x)
x = self.trans1(x)
x = self.block2(x)
x = self.trans2(x)
x = self.block3(x)
x = self.trans3(x)
x = self.block4(x)
x = self.bn2(x)
x = self.relu(x)
x = self.avgpool(x)
x = x.view(x.size(0), -1)
x = self.fc(x)
return x
```
相关推荐
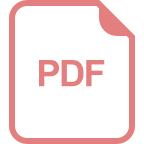
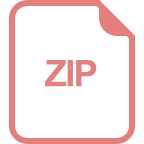
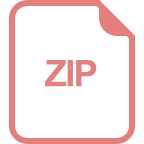














