python基于深度学习和opencv的车牌识别系统源码
时间: 2023-09-18 13:02:02 浏览: 195
车牌识别系统是一种基于深度学习和opencv的应用程序,通过对车辆图片或视频中的车牌进行识别。下面是一个简单的车牌识别系统的源码实现:
```python
import cv2
import numpy as np
import tensorflow as tf
# 加载预训练的深度学习模型
model = tf.keras.models.load_model('path/to/pretrained/model.h5')
# 加载车牌检测器
plate_cascade = cv2.CascadeClassifier('path/to/haarcascade_plate.xml')
# 车牌字符集
char_set = ['0', '1', '2', '3', '4', '5', '6', '7', '8', '9', 'A', 'B', 'C', 'D', 'E', 'F', 'G', 'H', 'J', 'K', 'L', 'M',
'N', 'P', 'Q', 'R', 'S', 'T', 'U', 'V', 'W', 'X', 'Y', 'Z']
# 读取车牌图片
image = cv2.imread('path/to/image.jpg')
# 转为灰度图像
gray = cv2.cvtColor(image, cv2.COLOR_BGR2GRAY)
# 检测车牌
plates = plate_cascade.detectMultiScale(gray, 1.1, 4)
# 遍历每个检测到的车牌
for (x, y, w, h) in plates:
# 提取车牌图像
plate_image = image[y:y + h, x:x + w]
# 调整尺寸为模型输入尺寸
resized_image = cv2.resize(plate_image, (80, 80))
# 转为灰度图像
grayscale_image = cv2.cvtColor(resized_image, cv2.COLOR_BGR2GRAY)
# 归一化
normalized_image = grayscale_image / 255.
# 添加批次维度
batched_image = np.expand_dims(normalized_image, axis=0)
# 使用深度学习模型进行预测
predictions = model.predict(batched_image)
# 解码预测结果
decoded_predictions = [char_set[np.argmax(p)] for p in predictions]
# 输出车牌识别结果
license_plate = ''.join(decoded_predictions)
print("识别结果:", license_plate)
# 绘制车牌框
cv2.rectangle(image, (x, y), (x + w, y + h), (0, 255, 0), 2)
# 标注车牌字符
cv2.putText(image, license_plate, (x, y), cv2.FONT_HERSHEY_SIMPLEX, 1, (0, 255, 0), 2)
# 显示结果图像
cv2.imshow('License Plate Detection', image)
cv2.waitKey(0)
cv2.destroyAllWindows()
```
以上是一个简单的车牌识别系统的源码示例,其中使用了训练好的深度学习模型对车牌进行识别,同时利用Haar特征的级联分类器对车牌进行检测。最终在图像上显示了检测到的车牌以及识别结果。请注意,这只是一个简单的示例,实际的车牌识别系统会更加复杂,需要根据具体需求进行进一步的优化和改进。
阅读全文
相关推荐
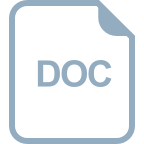
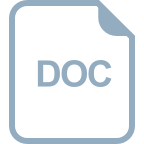
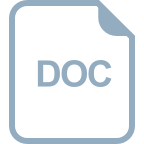
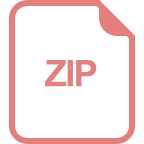
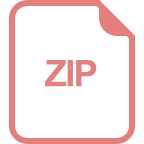
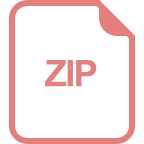
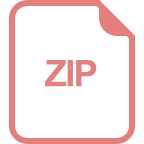
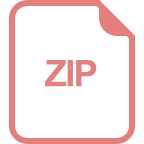
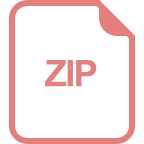
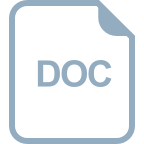
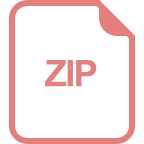
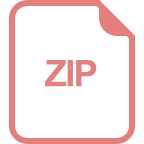
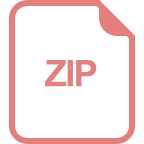
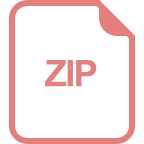
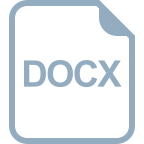
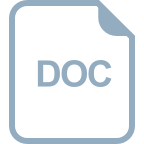
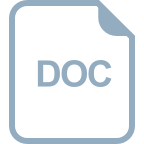
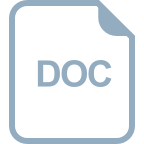
