解释代码contrast_feature = torch.cat(torch.unbind(features, dim=1), dim=0)
时间: 2024-04-18 09:23:31 浏览: 17
代码`contrast_feature = torch.cat(torch.unbind(features, dim=1 dim=0)`的作用是将维度为1的特征张量进行拼接,并将结果保存在`contrast_feature`中。
首先,`torch.unbind(features, dim=1)`将张量`features`在维度1上进行拆分,返回一个元组。这个元组包含了在维度1上切分的多个子张量。
然后,`torch.cat`函数接受一个可迭代对象作为输入,并沿指定的维度进行拼接。在这里,我们将通过`torch.unbind`得到的多个子张量作为可迭代对象传递给`torch.cat`函数,并指定`dim=0`表示在维度0上进行拼接。
最终,拼接的结果被赋值给变量`contrast_feature`,它将包含原始特征张量中所有维度为1的子张量按顺序拼接在一起的结果。
举个例子,假设原始特征张量`features`的形状为`(3, 4, 2)`,其中3表示样本数,4表示特征数,2表示维度1的大小。那么通过执行上述代码,`contrast_feature`将是一个形状为`(12, 2)`的张量,其中12是样本数和特征数的乘积,2是维度1的大小。
相关问题
这段代码有什么错误def forward(self,x): num_nodes = x.size(1) # sub_graph size batch_size = x.size(0) W = torch.cat([self.W] * batch_size, dim=0) representation = torch.matmul(x, W) r_sum = torch.sum(representation, dim=-1, keepdim=False) b = torch.zeros([batch_size, num_nodes]) b = Variable(b) one = torch.ones_like(r_sum) zero = torch.zeros_like(r_sum) label = torch.clone(r_sum) label = torch.where(label == 0, one, zero) b.data.masked_fill_(label.bool(), -float('inf')) num_iterations = 3 for i in range(num_iterations): c = torch.nn.functional.softmax(b, dim=-1) weight_coeff = c.unsqueeze(dim=1) representation_global = torch.matmul(weight_coeff, representation) representation_global_all = torch.cat([representation_global] * num_nodes, dim=1) representation_similarity = torch.nn.functional.cosine_similarity(representation, representation_global_all, dim=-1) representation_similarity.data.masked_fill_(label.bool(), -float('inf')) b = representation_similarity return representation_global.squeeze(dim=1)
这段代码中存在一个错误。在for循环中,代码更新了变量b,但是在更新后没有再次进行softmax操作,导致后续的计算结果不正确。因此,需要在for循环中,在更新b后再次进行softmax操作,如下所示:
```
for i in range(num_iterations):
c = torch.nn.functional.softmax(b, dim=-1)
weight_coeff = c.unsqueeze(dim=1)
representation_global = torch.matmul(weight_coeff, representation)
representation_global_all = torch.cat([representation_global] * num_nodes, dim=1)
representation_similarity = torch.nn.functional.cosine_similarity(representation, representation_global_all, dim=-1)
representation_similarity.data.masked_fill_(label.bool(), -float('inf'))
b = torch.nn.functional.softmax(representation_similarity, dim=-1) # 进行softmax操作
```
这样修改后,该段代码的功能应该能够正常运行。
把这段代码里的location_embedding_dim去掉class my_GAT(torch.nn.Module): def __init__(self, in_channels, out_channels, n_heads, location_embedding_dim, filters_1, filters_2, dropout): super(my_GAT, self).__init__() self.in_channels = in_channels self.out_channels = out_channels self.n_heads = n_heads location_embedding_dim = 0 self.filters_1 = filters_1 self.filters_2 = filters_2 self.dropout = dropout self.location_embedding_dim = location_embedding_dim self.setup_layers() def setup_layers(self): self.GAT_1 = GATConv(in_channels=self.in_channels,out_channels=self.filters_1, heads=self.n_heads, dropout=0.1) self.GAT_2 = GATConv(in_channels=self.filters_1 * self.n_heads + self.location_embedding_dim, out_channels=self.out_channels, heads=self.n_heads, dropout=0.1, concat=False) def forward(self, edge_indices, features, location_embedding): features = torch.cat((features, location_embedding), dim=-1) features = self.GAT_1(features, edge_indices) features = torch.nn.functional.relu(features) features = torch.nn.functional.dropout(features, p=self.dropout, training=self.training) features = torch.cat((features, location_embedding), dim=-1) features = self.GAT_2(features, edge_indices) return features
这段代码里的location_embedding_dim是一个参数,它表示位置嵌入的维度。如果你想去掉它,可以把它的值设为0,然后在构造函数中把self.location_embedding_dim也设为0即可。具体来说,修改后的代码如下:
```
class my_GAT(torch.nn.Module):
def __init__(self, in_channels, out_channels, n_heads, filters_1, filters_2, dropout):
super(my_GAT, self).__init__()
self.in_channels = in_channels
self.out_channels = out_channels
self.n_heads = n_heads
self.filters_1 = filters_1
self.filters_2 = filters_2
self.dropout = dropout
self.location_embedding_dim = 0
self.setup_layers()
def setup_layers(self):
self.GAT_1 = GATConv(in_channels=self.in_channels,out_channels=self.filters_1, heads=self.n_heads, dropout=0.1)
self.GAT_2 = GATConv(in_channels=self.filters_1 * self.n_heads, out_channels=self.out_channels, heads=self.n_heads, dropout=0.1, concat=False)
def forward(self, edge_indices, features, location_embedding):
features = self.GAT_1(features, edge_indices)
features = torch.nn.functional.relu(features)
features = torch.nn.functional.dropout(features, p=self.dropout, training=self.training)
features = self.GAT_2(features, edge_indices)
return features
```
相关推荐
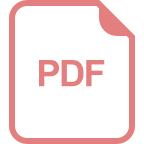
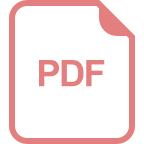
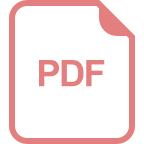













