C:\Users\adminstor\anaconda3\envs\python39\python.exe D:\daima\KalmanNet_TSP-main\main_lor_DT_NLobs.py Pipeline Start Current Time = 07.24.23_12:19:44 Using GPU 1/r2 [dB]: tensor(30.) 1/q2 [dB]: tensor(30.) Start Data Gen Data Load data_lor_v0_rq3030_T20.pt no chopping trainset size: torch.Size([1000, 3, 20]) cvset size: torch.Size([100, 3, 20]) testset size: torch.Size([200, 3, 20]) Evaluate EKF full Extended Kalman Filter - MSE LOSS: tensor(-26.4659) [dB] Extended Kalman Filter - STD: tensor(1.6740) [dB] Inference Time: 37.115127086639404 KalmanNet start Number of trainable parameters for KNet: 19938 Composition Loss: True Traceback (most recent call last): File "D:\daima\KalmanNet_TSP-main\main_lor_DT_NLobs.py", line 146, in <module> [MSE_cv_linear_epoch, MSE_cv_dB_epoch, MSE_train_linear_epoch, MSE_train_dB_epoch] = KalmanNet_Pipeline.NNTrain(sys_model, cv_input, cv_target, train_input, train_target, path_results) File "D:\daima\KalmanNet_TSP-main\Pipelines\Pipeline_EKF.py", line 150, in NNTrain MSE_trainbatch_linear_LOSS = self.alpha * self.loss_fn(x_out_training_batch, train_target_batch)+(1-self.alpha)*self.loss_fn(y_hat, y_training_batch) File "C:\Users\adminstor\anaconda3\envs\python39\lib\site-packages\torch\nn\modules\module.py", line 1102, in _call_impl return forward_call(*input, **kwargs) File "C:\Users\adminstor\anaconda3\envs\python39\lib\site-packages\torch\nn\modules\loss.py", line 520, in forward return F.mse_loss(input, target, reduction=self.reduction) File "C:\Users\adminstor\anaconda3\envs\python39\lib\site-packages\torch\nn\functional.py", line 3112, in mse_loss return torch._C._nn.mse_loss(expanded_input, expanded_target, _Reduction.get_enum(reduction)) RuntimeError: Expected all tensors to be on the same device, but found at least two devices, cuda:0 and cpu!
时间: 2023-09-08 14:10:06 浏览: 77
这错误是由于您的输入张量和目标张量位于不同的设备上(一个在 CUDA 设备上,另一个在 CPU 上)导致的。
为了解决这个问题,您可以使用 `.to()` 方法将两个张量都移动到相同的设备上。以下是一个示例修复:
```python
x_out_training_batch = x_out_training_batch.to(device)
train_target_batch = train_target_batch.to(device)
y_training_batch = y_training_batch.to(device)
```
在这个示例中,我们假设 `device` 是您指定的设备(例如 `cuda:0`)。通过使用 `.to()` 方法,我们将 `x_out_training_batch`、`train_target_batch` 和 `y_training_batch` 张量都移动到相同的设备上。
请确保在其他需要使用这些张量的地方也应用了类似的修复。
希望这可以帮助您解决问题!如果还有其他问题,请随时提问。
相关问题
Traceback (most recent call last): File "D:\daima\KalmanNet_TSP-main\main_lor_DT.py", line 204, in <module> [MSE_test_linear_arr, MSE_test_linear_avg, MSE_test_dB_avg,Knet_out,RunTime] = KNet_Pipeline.NNTest(sys_model_partial, test_input, test_target, path_results) File "D:\daima\KalmanNet_TSP-main\Pipelines\Pipeline_EKF.py", line 303, in NNTest x_out_test[:,:, t] = torch.squeeze(self.model(torch.unsqueeze(test_input[:,:, t],2))) File "C:\Users\adminstor\anaconda3\envs\python39\lib\site-packages\torch\nn\modules\module.py", line 1102, in _call_impl return forward_call(*input, **kwargs) File "D:\daima\KalmanNet_TSP-main\KNet\KalmanNet_nn.py", line 322, in forward return self.KNet_step(y) File "D:\daima\KalmanNet_TSP-main\KNet\KalmanNet_nn.py", line 229, in KNet_step self.step_KGain_est(y) File "D:\daima\KalmanNet_TSP-main\KNet\KalmanNet_nn.py", line 215, in step_KGain_est KG = self.KGain_step(obs_diff, obs_innov_diff, fw_evol_diff, fw_update_diff) File "D:\daima\KalmanNet_TSP-main\KNet\KalmanNet_nn.py", line 289, in KGain_step out_FC7 = self.FC7(in_FC7) File "C:\Users\adminstor\anaconda3\envs\python39\lib\site-packages\torch\nn\modules\module.py", line 1102, in _call_impl return forward_call(*input, **kwargs) File "C:\Users\adminstor\anaconda3\envs\python39\lib\site-packages\torch\nn\modules\container.py", line 141, in forward input = module(input) File "C:\Users\adminstor\anaconda3\envs\python39\lib\site-packages\torch\nn\modules\module.py", line 1102, in _call_impl return forward_call(*input, **kwargs) File "C:\Users\adminstor\anaconda3\envs\python39\lib\site-packages\torch\nn\modules\linear.py", line 103, in forward return F.linear(input, self.weight, self.bias) File "C:\Users\adminstor\anaconda3\envs\python39\lib\site-packages\torch\nn\functional.py", line 1848, in linear return torch._C._nn.linear(input, weight, bias) RuntimeError: mat1 and mat2 shapes cannot be multiplied (200x6 and 2x10)
这个错误通常是由于张量形状不匹配导致的。根据错误信息,`mat1`和`mat2`的形状无法进行矩阵相乘,因为`mat1`的形状是(200x6),而`mat2`的形状是(2x10)。
要解决这个问题,你需要调整输入张量的形状,使其能够进行矩阵乘法操作。这可能需要检查你的模型结构和输入数据的维度是否正确。
另外,你还可以使用`torch.matmul()`函数来执行矩阵乘法,它能够自动处理形状不匹配的情况。例如:
```python
output = torch.matmul(mat1, mat2)
```
请检查你的代码中涉及到矩阵乘法的部分,确保输入的形状是匹配的。如果还有其他问题,请提供更多的代码和上下文信息,以便我能够更好地帮助你。
Traceback (most recent call last): File "D:\daima\KalmanNet_TSP-main\main_linear_CA.py", line 182, in <module> Plot.plotTraj_CA(test_target, KF_out, KNet_out, dim=0, file_name=PlotfolderName+PlotfileName0)#Position File "D:\daima\KalmanNet_TSP-main\Plot.py", line 350, in plotTraj_CA plt.plot(x_plt, RTS_out[0][0,:], label=legend[2]) File "C:\Users\adminstor\anaconda3\envs\python39\lib\site-packages\matplotlib\pyplot.py", line 2840, in plot return gca().plot( File "C:\Users\adminstor\anaconda3\envs\python39\lib\site-packages\matplotlib\axes\_axes.py", line 1745, in plot self.add_line(line) File "C:\Users\adminstor\anaconda3\envs\python39\lib\site-packages\matplotlib\axes\_base.py", line 1964, in add_line self._update_line_limits(line) File "C:\Users\adminstor\anaconda3\envs\python39\lib\site-packages\matplotlib\axes\_base.py", line 1986, in _update_line_limits path = line.get_path() File "C:\Users\adminstor\anaconda3\envs\python39\lib\site-packages\matplotlib\lines.py", line 1011, in get_path self.recache() File "C:\Users\adminstor\anaconda3\envs\python39\lib\site-packages\matplotlib\lines.py", line 658, in recache y = _to_unmasked_float_array(yconv).ravel() File "C:\Users\adminstor\anaconda3\envs\python39\lib\site-packages\matplotlib\cbook\__init__.py", line 1289, in _to_unmasked_float_array return np.asarray(x, float) File "C:\Users\adminstor\anaconda3\envs\python39\lib\site-packages\numpy\core\_asarray.py", line 102, in asarray return array(a, dtype, copy=False, order=order) File "C:\Users\adminstor\anaconda3\envs\python39\lib\site-packages\torch\_tensor.py", line 680, in __array__ return self.numpy().astype(dtype, copy=False) TypeError: can't convert cuda:0 device type tensor to numpy. Use Tensor.cpu() to copy the tensor to host memory first.
这个错误是由于您正在尝试将 CUDA 上的张量直接转换为 NumPy 数组时引起的。在转换之前,您需要将 CUDA 上的张量复制到主机内存中。
您可以尝试使用 `.cpu()` 方法将张量从 CUDA 设备移动到主机内存,然后再进行转换。以下是一个示例:
```python
y = y.cpu().numpy()
```
在这个示例中,我们将 `y` 张量从 CUDA 设备移动到主机内存,然后使用 `.numpy()` 方法将其转换为 NumPy 数组。
请注意,您可能需要在其他需要将 CUDA 张量转换为 NumPy 数组的地方应用类似的修复。
希望这可以解决您的问题!如果还有其他问题,请随时提问。
相关推荐
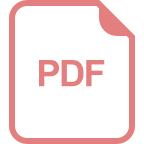

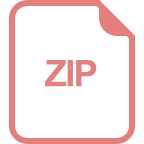
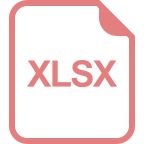
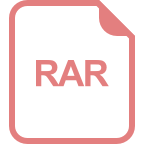
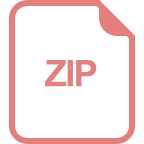
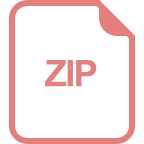
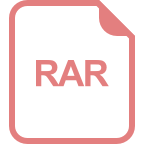
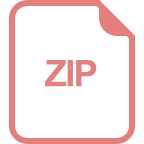
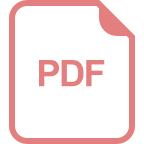
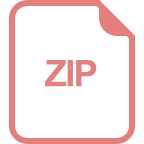
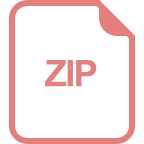
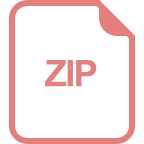
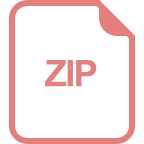