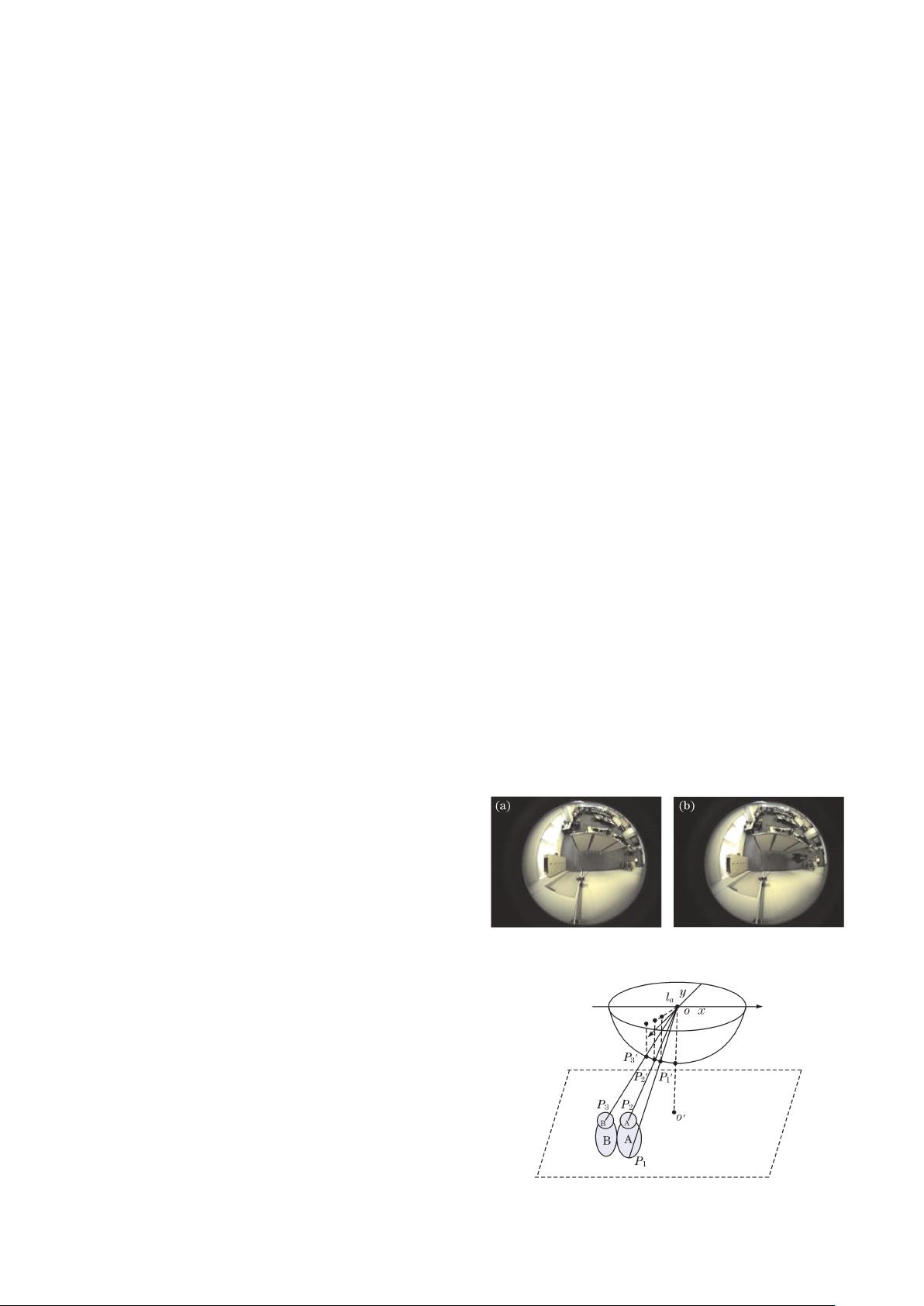
February 10, 2011 / Vol. 9, No. 2 / CHINESE OPTICS LETTERS 021101-1
Automatic surveillance system using f ish-eye lens camera
Xue Yuan ( ÈÈÈ)
∗
, Yongduan Song (yyy[[[ààà), and Xueye Wei (ÆÆÆ)
Center for Intelligent Systems and Renewable Energy, Beijing Jiaotong University, Beijing 100044, China
∗
Corresp onding author: xyuan@bjtu.edu.cn
Received June 24, 2010; accepted Octob er 21, 2010; posted online January 28, 2011
This letter presents an automatic surveillance system using fish-eye lens camera. Our system achieves
wide-area automatic surveillance without a dead angle using only one camera. We propose a new human
detection method to select the most adaptive classifier based on the locations of the human candidates.
Human regions are detected from the fish-eye image effectively and are corrected for perspective versions.
An experiment is performed on indoor video sequences with different illumination and crowded conditions,
with results demonstrating the efficiency of our algorithm.
OCIS co des: 110.0110, 100.0100, 150.0150.
doi: 10.3788/COL201109.021101.
Due to large field of view, wide-angle lens are popu-
larly used for various applications, such as surveillance,
robotic navigation, and semi-automatic parking systems.
Because the angle of view of the fish-eye lens used in
our system was up to 185
◦
, it achieved effective wide-
area surveillance without a dead angle only one camera.
However, it brought an inherent distortion in the image,
and this distorted image must be rectified or restored in
order to recognize and understand the image accurately.
Human detection and tracking is a necessary approach
for automatic surveillance systems. However, in the im-
age taken by our surveillance system, the region where
human enters the surveillance space is distorted and it is
difficult to detect humans using the original method in-
troduced in Refs. [1−9]. To our knowledge, there is still
no reliable pedestrian detection algorithm reported for
fish-eye image. Refernece [10] proposed human detection
method using fish-eye image to detect ellipses from the
subtraction images of fish-eye pictures as human area.
However, in a more crowded situation and when sudden
illumination changes occur, their method shows a clear
increase in false alarm rate.
In order to improve the efficiency of human detection
on fish-eye images even in crowded indoor environments,
we propose a human detection method. The rotations
and sizes of the human regions on the fish-eye image
change based on the locations of humans in the surveil-
lance area. We propose a method to normalize these
regions. Because a fish-eye lens camera is set on top of
the surveillance area, the shapes of humans are changed
based on their locations in the surveillance area. In
this letter, we create three types of classifiers to detect
humans in any part of the surveillance area; the most
adaptive classifier for each human is chosen automati-
cally from several classifiers. Moreover, we propose a
method to minimize the occlusion effects. We infer the
possible occlusion region in each human candidate region
based on its location on the fish-eye image. Once the
occluded regions are detected, the occlusion effects can
be minimized by adjusting the threshold of the classifier.
Unlike other systems such as those proposed in Refs.
[11,12], the human regions in our proposed method are
detected initially from the fish-eye image, and only the
human regions are corrected afterwards. In other sys-
tems, the entire input fish-eye images are corrected
first and then the human regions are detected from
the corrected images. Using our system, the processing
efficiency can be improved and the processing time can
be significantly reduced.
The system is designed as illustrated in Fig. 1, wherein
the fish-eye lens camera is set on top of the surveillance
area. The input image of the fish-eye lens camera is illus-
trated in Fig. 2, with the background image illustrated
in Fig. 2(a) and the input image illustrated in Fig. 2(b).
The edges of the background and input images are
extracted using Sobel operator
[13]
as illustrated in Figs.
3(a) and (b). In addition, the subtraction image between
the input edge image and the background edge image is
computed, as illustrated in Fig. 3(c). As shown in Fig.
3, all the head edges look like ellipses; thus, an efficient
ellipse detection method
[14]
is adopted to extract the el-
Fig. 1. Image taken by the proposed surveillance system. (a)
Background image; (b) input image.
Fig. 2. Proposed surveillance system.
1671-7694/2011/021101(5)
c
° 2011 Chinese Optics Letters