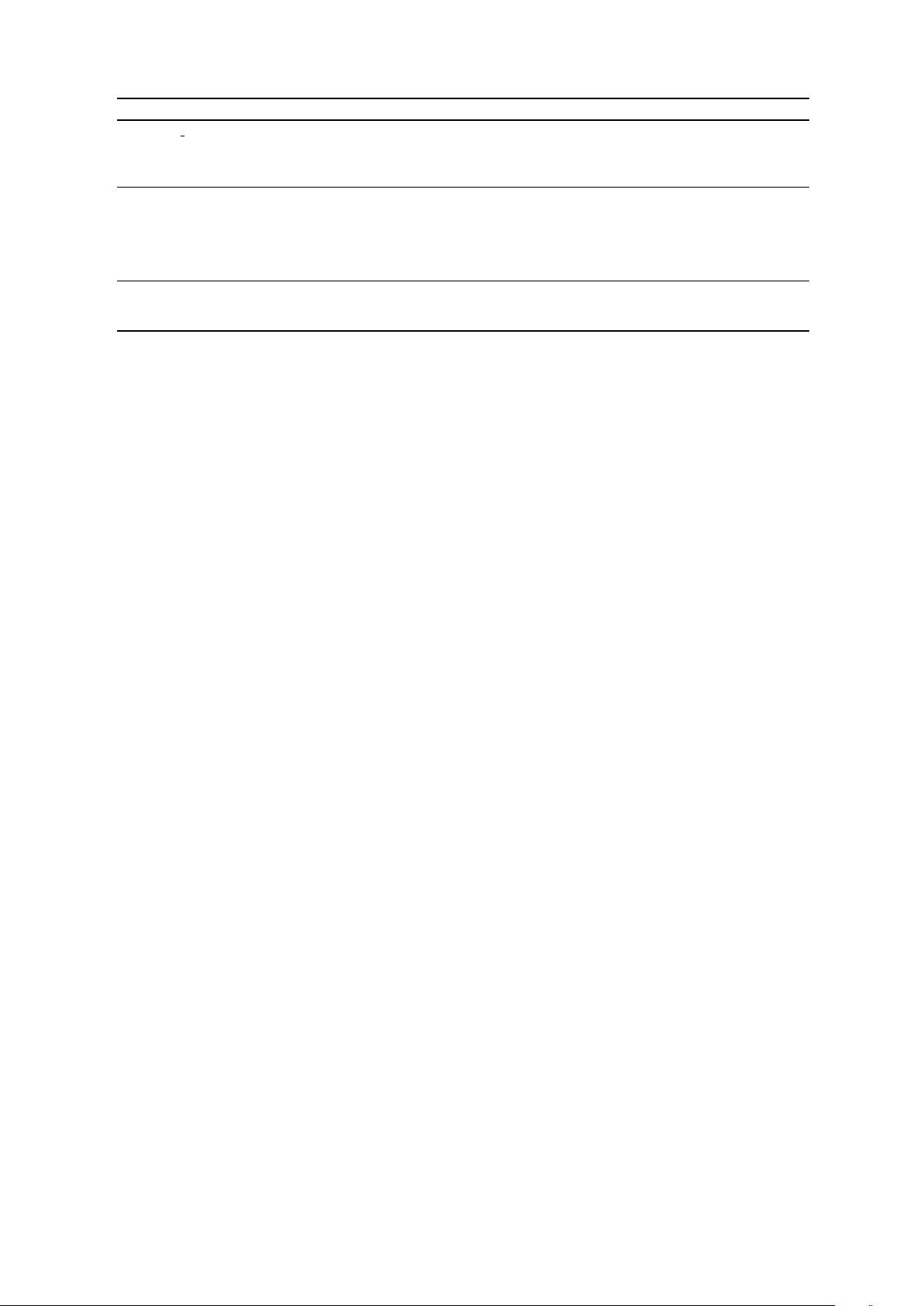
1038
Datasets↓ Metrics→ #Dialogs #Utterances Dialog types Domains User profile
Facebook Rec (Dodge et al., 2016)
1M 6M Rec. Movie No
REDIAL (Li et al., 2018) 10k 163k
Rec., chitchat
Movie No
GoRecDial (Kang et al., 2019) 9k 170k Rec. Movie Yes
OpenDialKG (Moon et al., 2019) 12k 143k Rec. Movie, book No
CMU DoG (Zhou et al., 2018a) 4k 130k Chitchat Movie No
IIT DoG (Moghe et al., 2018) 9k 90k Chitchat Movie No
Wizard-of-wiki (Dinan et al., 2019)
22k 202k Chitchat 1365 Wikipedia topics No
OpenDialKG (Moon et al., 2019) 3k 38k Chitchat Sports, music No
DuConv (Wu et al., 2019) 29k 270k Chitchat Movie No
KdConv (Zhou et al., 2020) 4.5k 86k Chitchat Movie, music, travel No
DuRecDial 10.2k 156k
Rec.,
chitchat,
QA, task
Movie, music, movie
star, food, restaurant,
news, weather
Yes
Table 1: Comparison of our dataset DuRecDial to recommendation dialog datasets and knowledge grounded dialog
datasets. “Rec.” stands for recommendation.
2 Related Work
Datasets for Conversational Recommendation
To facilitate the study of conversational recommen-
dation, multiple datasets have been created in pre-
vious work, as shown in Table 1. The first rec-
ommendation dialog dataset is released by Dodge
et al. (2016), which is a synthetic dialog dataset
built with the use of the classic MovieLens ratings
dataset and natural language templates. Li et al.
(2018) creates a human-to-human multi-turn rec-
ommendation dialog dataset, which combines the
elements of social chitchat and recommendation
dialogs. Kang et al. (2019) provides a recommen-
dation dialogue dataset with clear goals, and Moon
et al. (2019) collects a parallel Dialog
↔
KG cor-
pus for recommendation. Compared with them,
our dataset contains multiple dialog types, multi-
domain use cases, and rich interaction variability.
Datasets for Knowledge Grounded Conver-
sation
As shown in Table 1, CMU DoG (Zhou
et al., 2018a) explores two scenarios for Wikipedia-
article grounded dialogs: only one participant has
access to the document, or both have. IIT DoG
(Moghe et al., 2018) is another dialog dataset for
movie chats, wherein only one participant has ac-
cess to background knowledge, such as IMDB’s
facts/plots, or Reddit’s comments. Dinan et al.
(2019) creates a multi-domain multi-turn conversa-
tions grounded on Wikipedia articles. OpenDialKG
(Moon et al., 2019) provides a chit-chat dataset be-
tween two agents, aimed at the modeling of dialog
logic by walking over knowledge graph-Freebase.
Wu et al. (2019) provides a Chinese dialog dataset-
DuConv, where one participant can proactively lead
the conversation with an explicit goal. KdConv
(Zhou et al., 2020) is a Chinese dialog dataset,
where each dialog contains in-depth discussions
on multiple topics. In comparison with them, our
dataset contains multiple dialog types, clear goals
to achieve during each conversation, and user pro-
files for personalized conversation.
Models for Conversational Recommendation
Previous work on conversational recommender sys-
tems fall into two categories: (1) task-oriented
dialog-modeling approaches in which the systems
ask questions about user preference over prede-
fined slots to select items for recommendation
(Christakopoulou et al., 2018, 2016; Lee et al.,
2018; Reschke et al., 2013; Sun and Zhang, 2018;
Warnestal, 2005; Zhang et al., 2018b); (2) non-task
dialog-modeling approaches in which the models
learn dialog strategies from the dataset without pre-
defined task slots and then make recommendations
without slot filling (Chen et al., 2019; Kang et al.,
2019; Li et al., 2018; Moon et al., 2019; Zhou et al.,
2018a). Our work is more close to the second cat-
egory, and differs from them in that we conduct
multi-goal planning to make proactive conversa-
tional recommendation over multi-type dialogs.
Goal Driven Open-domain Conversation
Generation
Recently, imposing goals on open-
domain conversation generation models having at-
tracted lots of research interests (Moon et al., 2019;
Li et al., 2018; Tang et al., 2019b; Wu et al., 2019)
since it provides more controllability to conversa-
tion generation, and enables many practical appli-
cations, e.g., recommendation of engaging entities.
However, these models can just produce a dialog
towards a single goal, instead of a goal sequence as
done in this work. We notice that the model by Xu
et al. (2020) can conduct multi-goal planning for
conversation generation. But their goals are limited